Identification of linear systems in canonical form through an EM framework
ICASSP(2010)
摘要
Least-squares estimation has always been the main approach when applying prediction error methods (PEM) in the identification of linear dynamical systems. Regardless of the estimation algorithm, if there are no restrictions on the form of the matrices we want to estimate, the matrices can be determined up to within a linear transformation and thus the result may be different than the true solution and the convergence of iterative algorithms may be affected. In this paper, we apply a new identification procedure based on the Expectation Maximization framework to a family of identifiable state-space models. To our knowledge, this is the first complete solution of Maximum-Likelihood estimation for general linear state-space models.
更多查看译文
关键词
mimo state space models,state-space methods,system identification,maximum likelihood estimation,state space models,information theory,prediction error methods,simulation,expectation maximization,linear systems,linear system identification,canonical form,expectation maximization framework,state space model,kalman filters,mimo,linear transformation,maximum likelihood estimate,steady state,linear system,mathematical model,linear dynamical system,iterative algorithm,convergence
AI 理解论文
溯源树
样例
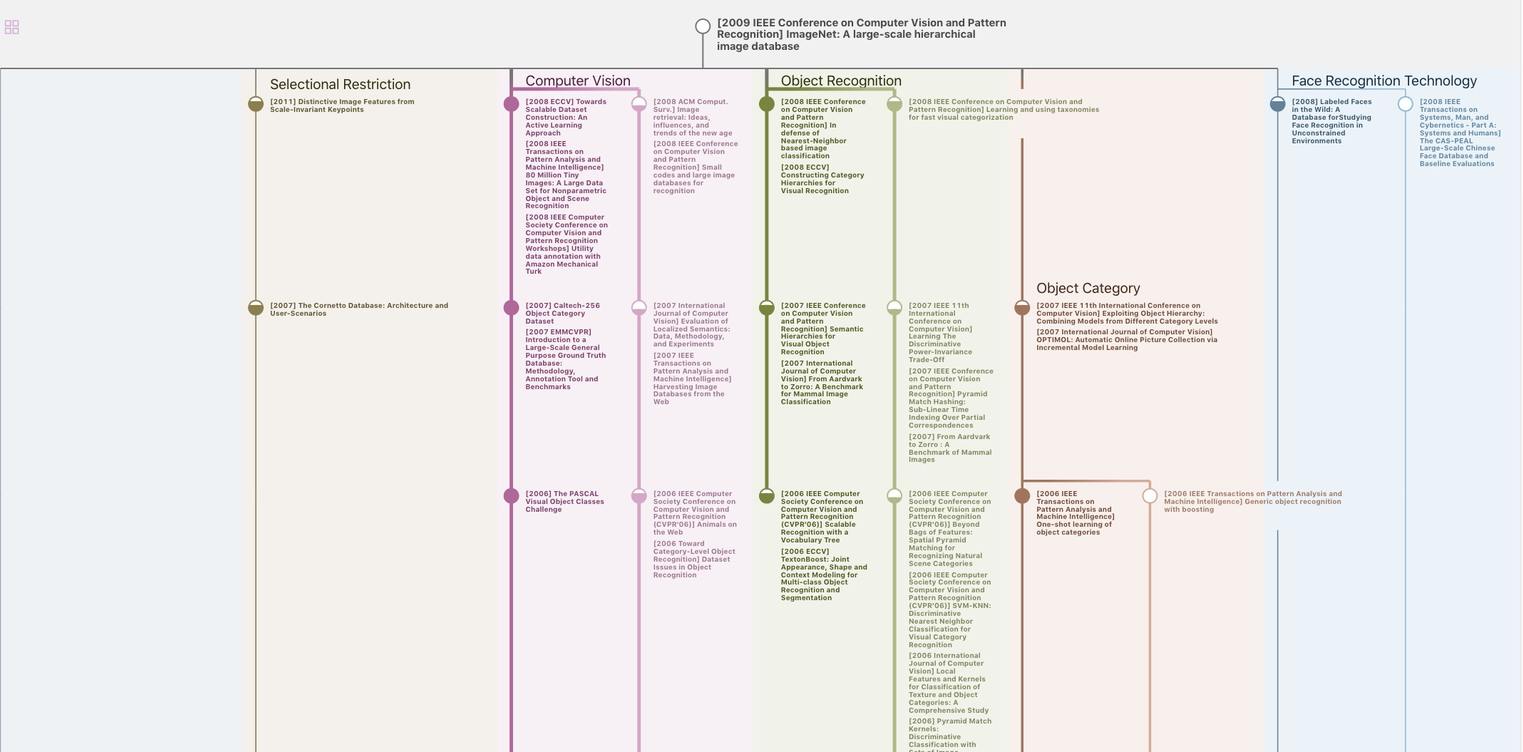
生成溯源树,研究论文发展脉络
Chat Paper
正在生成论文摘要