Implementing random scan Gibbs samplers
Computational Statistics(2005)
摘要
Summary The Gibbs sampler, being a popular routine amongst Markov chain Monte Carlo sampling methodologies, has revolutionized the application of Monte Carlo methods in statistical computing practice. The performance of the Gibbs sampler relies heavily on the choice of sweep strategy, that is, the means by which the components or blocks of the random vector X of interest are visited and updated. We develop an automated, adaptive algorithm for implementing the optimal sweep strategy as the Gibbs sampler traverses the sample space. The decision rules through which this strategy is chosen are based on convergence properties of the induced chain and precision of statistical inferences drawn from the generated Monte Carlo samples. As part of the development, we analytically derive closed form expressions for the decision criteria of interest and present computationally feasible implementations of the adaptive random scan Gibbs sampler via a Gaussian approximation to the target distribution. We illustrate the results and algorithms presented by using the adaptive random scan Gibbs sampler developed to sample multivariate Gaussian target distributions, and screening test and image data.
更多查看译文
关键词
Markov chain Monte Carlo,Gaussian approximation,adaptive algorithms,optimal sweep strategies,convergence rate,asymptotic risk
AI 理解论文
溯源树
样例
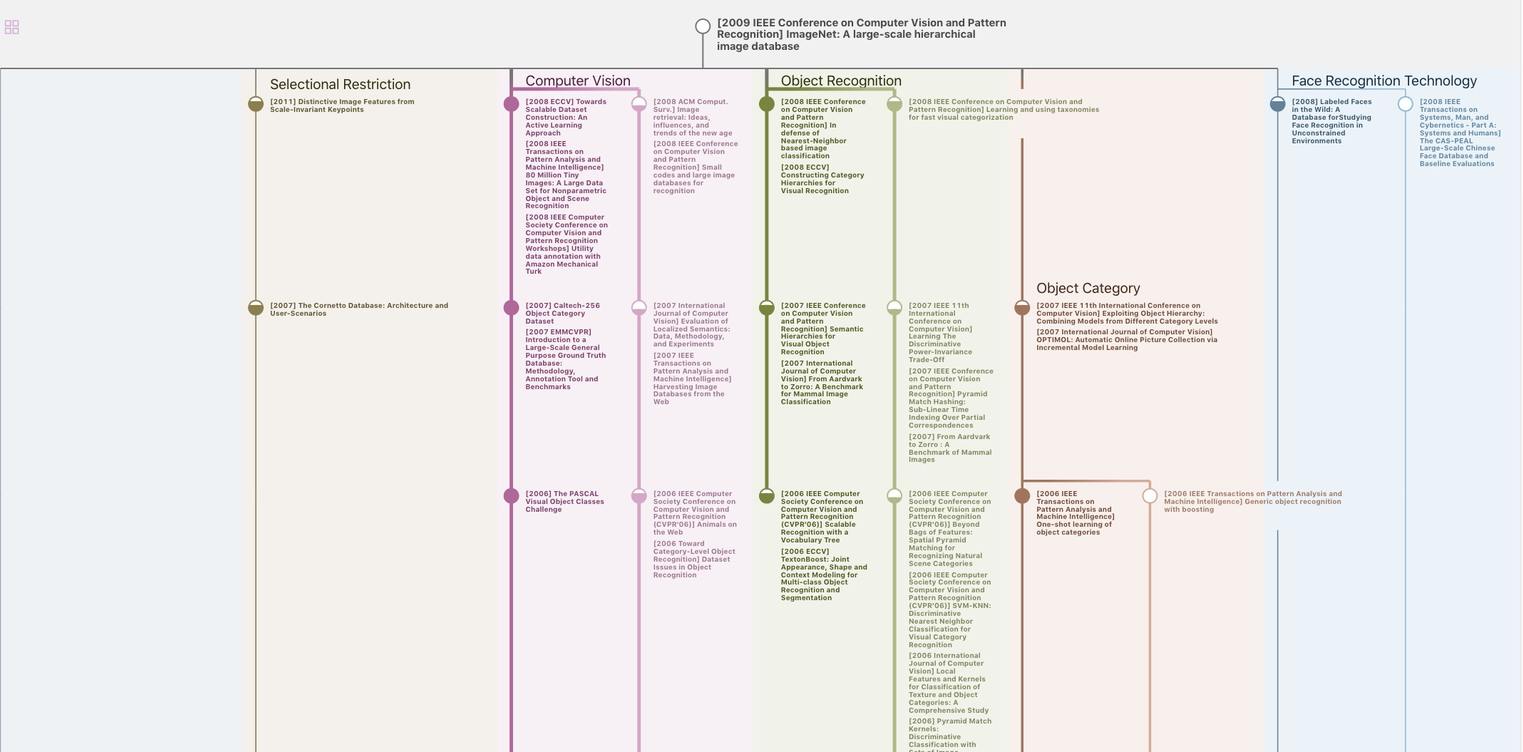
生成溯源树,研究论文发展脉络
Chat Paper
正在生成论文摘要