Incremental Topic-Based Translation Model Adaptation for Conversational Spoken Language Translation.
ACL(2013)
摘要
We describe a translation model adaptation approach for conversational spoken language translation (CSLT), which encourages the use of contextually appropriate translation options from relevant training conversations. Our approach employs a monolingual LDA topic model to derive a similarity measure between the test conversation and the set of training conversations, which is used to bias translation choices towards the current context. A significant novelty of our adaptation technique is its incremental nature; we continuously update the topic distribution on the evolving test conversation as new utterances become available. Thus, our approach is well-suited to the causal constraint of spoken conversations. On an English-to-Iraqi CSLT task, the proposed approach gives significant improvements over a baseline system as measured by BLEU, TER, and NIST. Interestingly, the incremental approach outperforms a non-incremental oracle that has up-front knowledge of the whole conversation.
更多查看译文
关键词
translation model adaptation,language,topic-based
AI 理解论文
溯源树
样例
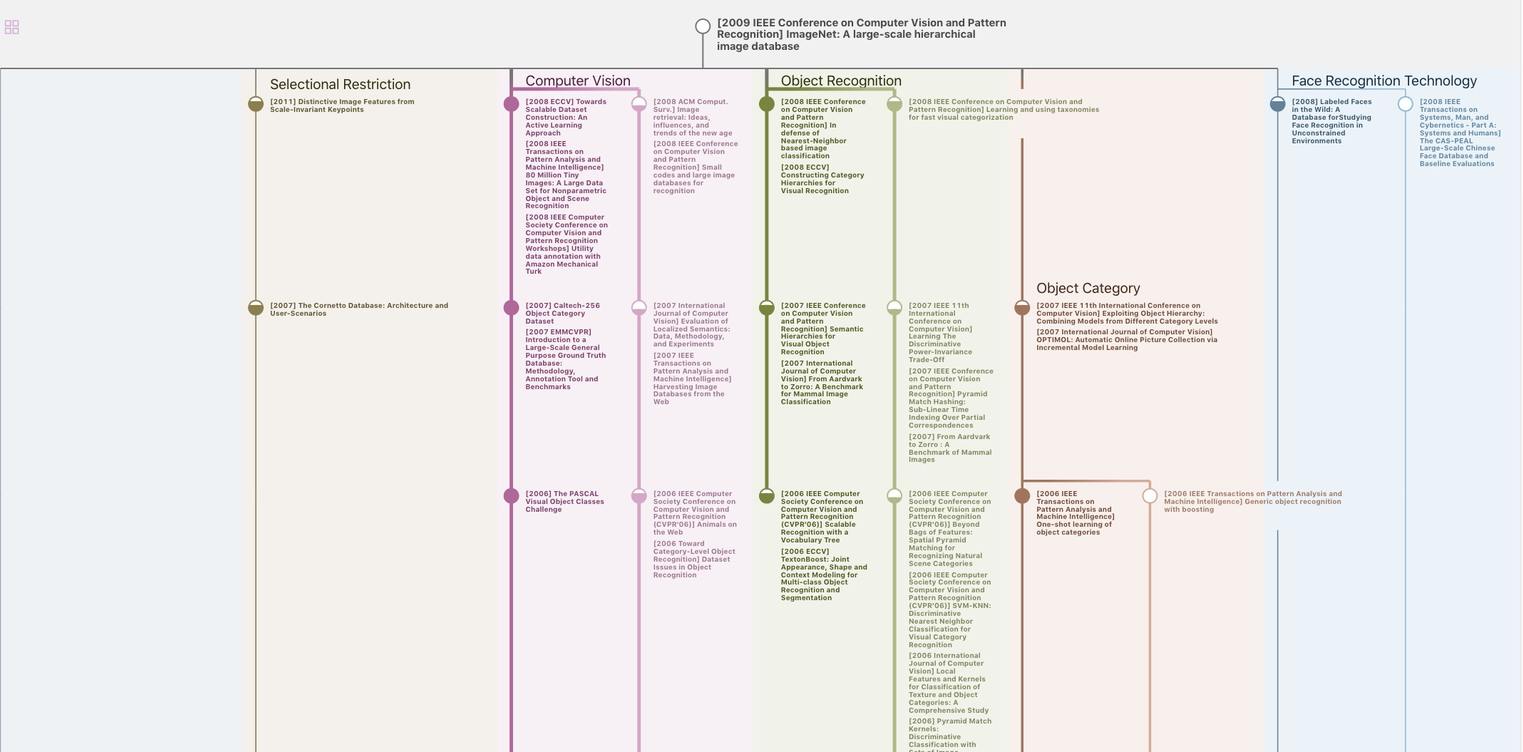
生成溯源树,研究论文发展脉络
Chat Paper
正在生成论文摘要