A Comparison of Variational and Markov Chain Monte Carlo Methods for Inference in Partially Observed Stochastic Dynamic Systems
Journal of Signal Processing Systems(2008)
摘要
In recent work we have developed a novel variational inference method for partially observed systems governed by stochastic differential equations. In this paper we provide a comparison of the Variational Gaussian Process Smoother with an exact solution computed using a Hybrid Monte Carlo approach to path sampling, applied to a stochastic double well potential model. It is demonstrated that the variational smoother provides us a very accurate estimate of mean path while conditional variance is slightly underestimated. We conclude with some remarks as to the advantages and disadvantages of the variational smoother.
更多查看译文
关键词
Data assimilation,Signal processing,Nonlinear smoothing,Variational approximation,Bayesian computation
AI 理解论文
溯源树
样例
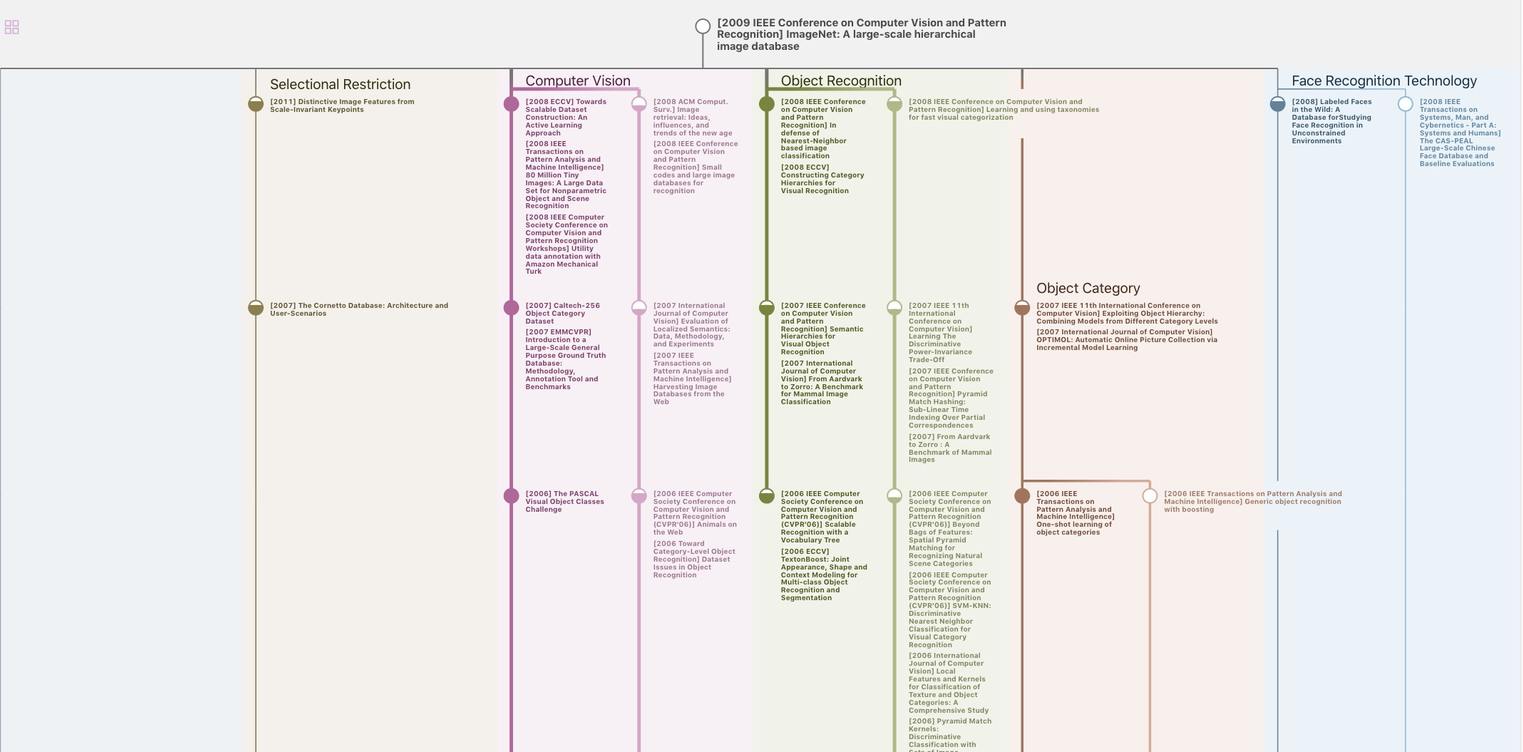
生成溯源树,研究论文发展脉络
Chat Paper
正在生成论文摘要