Integrating MHC Class I visibility targets into the ProteinMPNN protein design process
biorxiv(2024)
摘要
ProteinMPNN is crucial in many protein design pipelines, identifying amino acid (AA) sequences that fold into given 3D protein backbone structures. We explore ProteinMPNN in the context of designing therapeutic proteins that need to avoid triggering unwanted immune reactions. More specifically, we focus on intra-cellular proteins that face the challenge of evading detection by Cytotoxic T-lymphocytes (CTLs) that detect their presence via the MHC Class I (MHC-I) pathway. To reduce visibility of the designed proteins to this immune-system component, we develop a framework that uses the large language model (LLM) tuning method, Direct Preference Optimization (DPO), to guide ProteinMPNN in minimizing the number of predicted MHC-I epitopes in its designs. Our goal is to design proteins with low MHC-I immune-visibility while preserving the original structure and function. For our assessment, we first use AlphaFold to predict the 3D structures of designed protein sequences. We then use TM-score, that measures the structural alignment between the predicted design and original protein, to evaluate fidelity to the original protein structure. We find our LLM-based tuning method for constraining MHC-I visibility is able to effectively reduce visibility without compromising structural similarity to the original protein.
### Competing Interest Statement
The authors have declared no competing interest.
* AA
: amino acid
Ab
: antibody
AR
: auto-regressive
CTL
: Cytotoxic T-lymphocyte
DPO
: Direct Preference Optimization
GAN
: Generative Adversarial Network
LLM
: large language model
MD
: Molecular Dynamics
MHC-II
: MHC Class II
MHC-I
: MHC Class I
ML
: machine learning
MPNN
: message passing neural network
NLP
: Natural Language Processing
PPO
: Proximal Policy Optimization
PWM
: position weight matrix
RBF
: radial basis function
RL
: reinforcement learning
RLHF
: reinforcement learning from human feedback
RNA
: ribonucleic acid
SOTA
: state of the art
VAE
: Variational Autoencoder
更多查看译文
AI 理解论文
溯源树
样例
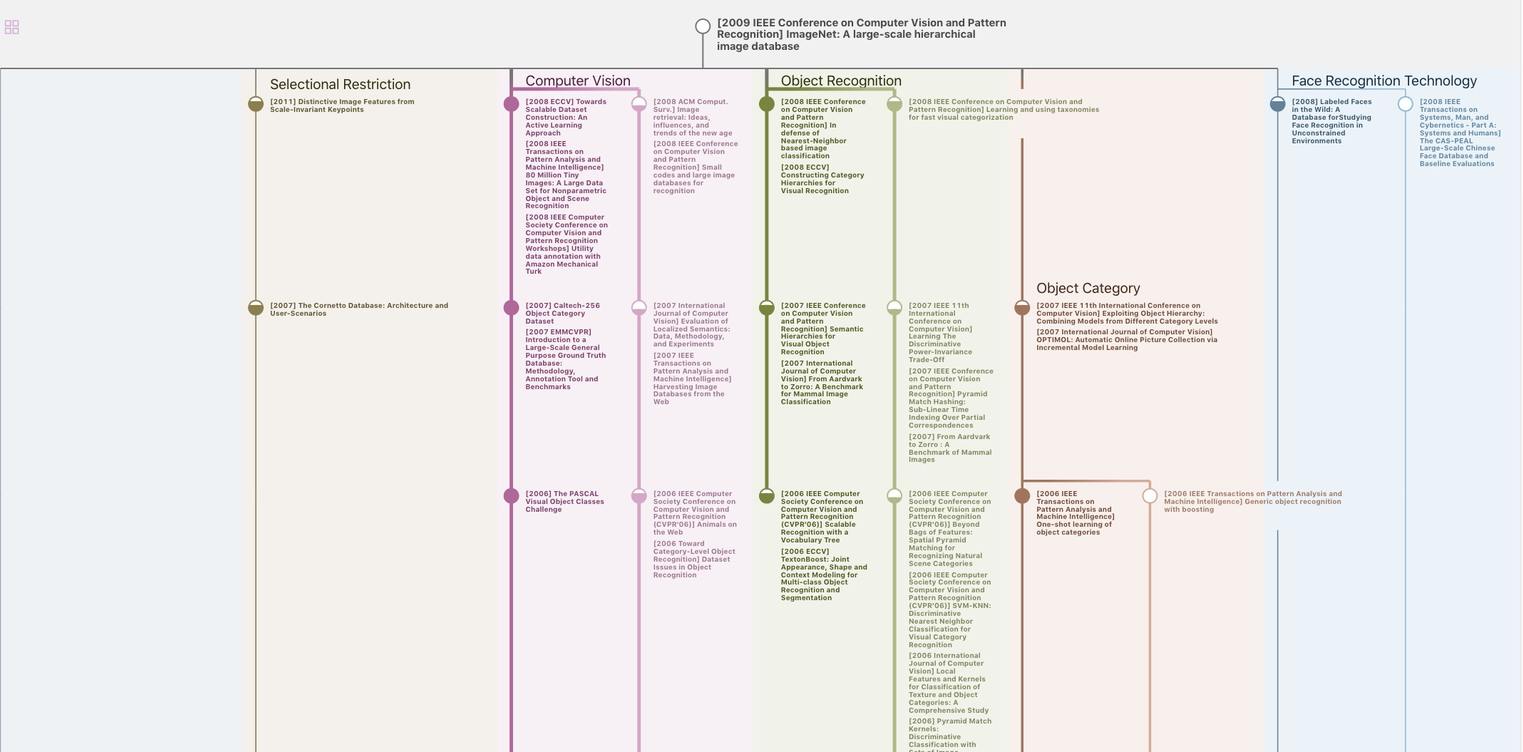
生成溯源树,研究论文发展脉络
Chat Paper
正在生成论文摘要