Magnitude Bounded Matrix Factorisation for Recommender Systems
IEEE Transactions on Knowledge and Data Engineering(2022)
摘要
Low rank matrix factorisation is often used in recommender systems as a way of extracting latent features. When dealing with large and sparse datasets, traditional recommendation algorithms face the problem of acquiring large, unrestrained, fluctuating values over predictions. Imposing bounding constraints has been proven an effective solution. However, existing bounding algorithms can only deal w...
更多查看译文
关键词
Sparse matrices,Prediction algorithms,Recommender systems,Optimization,Acceleration,Time complexity,Electronic mail
AI 理解论文
溯源树
样例
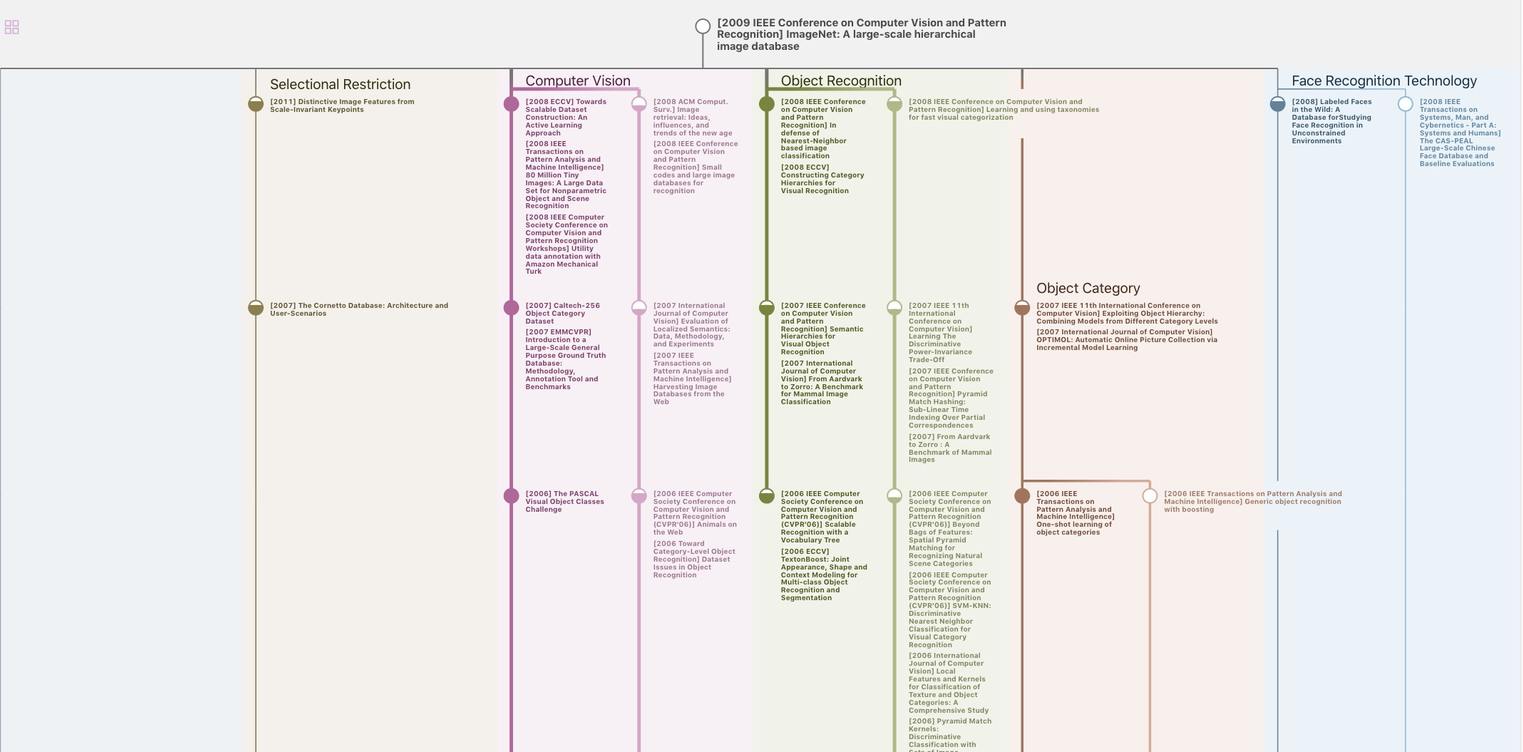
生成溯源树,研究论文发展脉络
Chat Paper
正在生成论文摘要