Curing the Prevalence Effect in Visual Search
Journal of Vision(2010)
摘要
In many socially important search tasks (e.g. medical and baggage screening) targets are rare. Previously, we have demonstrated that miss error rates are 2–3 times higher at low (1–2%) target prevalence than at high (50%) prevalence (Wolfe, Horowitz, & Kenner, Nature, 2005). This prevalence effect is robust and difficult to cure. Last year, we demonstrated that it reflects a persistent shift in decision criterion, rather than a loss of sensitivity (Van Wert, Horowitz, Place, & Wolfe, VSS06). At low prevalence, observers make more misses and fewer false alarms. In settings where miss errors are much less desirable than false alarms, we could “cure” the prevalence effect if we could get observers to maintain the high prevalence criterion (roughly equal false alarms and miss errors) during low prevalence search. Stimuli were simulations of baggage assembled from x-ray images of weapons and non-weapon objects. Bags contained 3, 6, 12, or 18 objects. Observers searched for guns and knives. In Experiment One, we added brief bursts of high prevalence trials to long periods of low prevalence search. This didn't work. Observers rapidly shifted criterion to the desired point when prevalence was high, but just as rapidly shifted back when prevalence was low. In Experiment Two, however, we had greater success by manipulating feedback. Observers received no feedback during low prevalence search and full feedback during 40-trial, high prevalence retraining episodes. Without retraining, miss error rates were 0.45 with these stimuli at low prevalence. With retraining, the low prevalence miss rate was 0.21, essentially the same as the 0.18 high prevalence rate. This retraining strategy may be practical in the field. Periodic high prevalence retraining with full feedback could proceed off-line, while the actual screening of baggage or medical images could proceed at low prevalence with minimal feedback.
更多查看译文
关键词
Visual Attention,Diagnostic Accuracy,Bottom-Up Attention,Top-Down Attention,Eye-Tracking Study
AI 理解论文
溯源树
样例
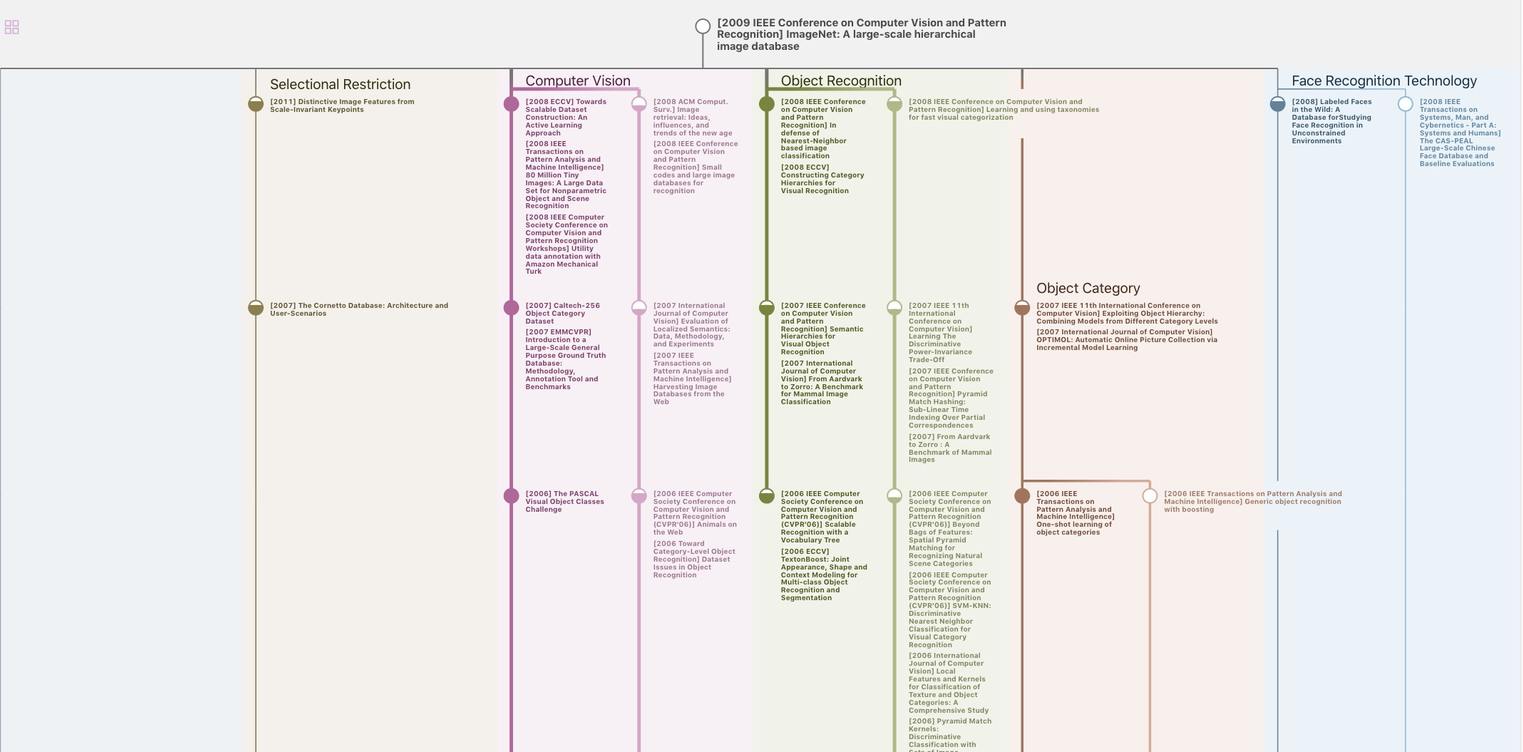
生成溯源树,研究论文发展脉络
Chat Paper
正在生成论文摘要