Improved phonotactic language identification using random forest language models
ICASSP(2008)
摘要
Recently a new language model, the random forest language model (RFLM), has been proposed and shown encouraging results in speech recognition tasks. In this paper we applied the RFLM to language identification tasks. We proposed a shared backoff smoothing to deal with data sparseness problem. Experiments were conducted on a subset of NIST 2003 language recognition evaluation data. The RFLM obtained 15.7% relative error rate reduction comparing with the standard trigram LM. The RFLM can be used as a counterpart to n-gram LM and BTLM for system fusion. We also empirically studied the relation between system performance and the tree numbers in a RFLM.
更多查看译文
关键词
random forest language models,shared backoff smoothing,language identification,speech recognition,system fusion,sparseness problem,decision tree language models,n-gram lm,phonotactic language identification,natural language processing,decision tree,decision trees,empirical study,language model,system performance,random forest,relative error
AI 理解论文
溯源树
样例
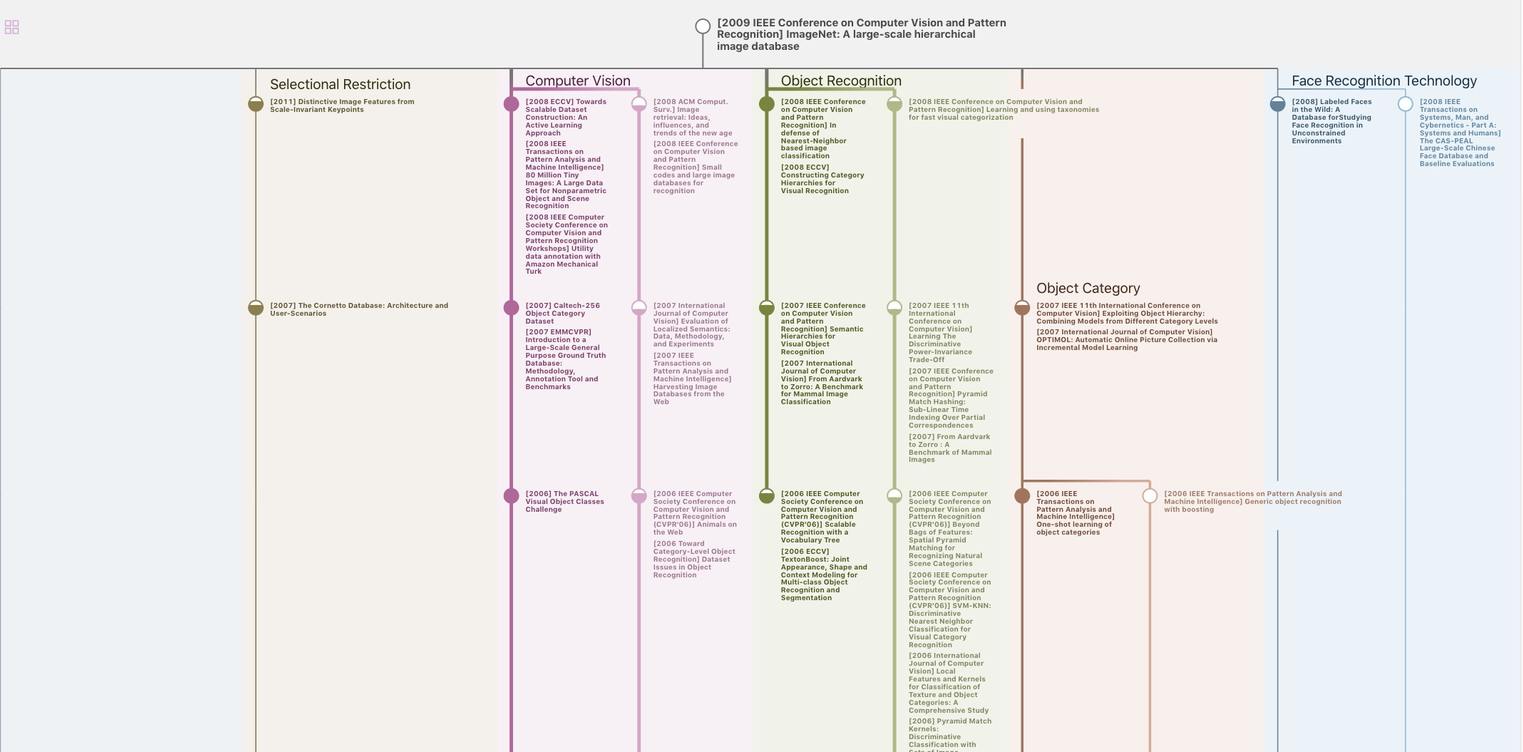
生成溯源树,研究论文发展脉络
Chat Paper
正在生成论文摘要