Recurrent Neural Network Based Language Model
11TH ANNUAL CONFERENCE OF THE INTERNATIONAL SPEECH COMMUNICATION ASSOCIATION 2010 (INTERSPEECH 2010), VOLS 1-2(2010)
摘要
A new recurrent neural network based language model (RNN LM) with applications to speech recognition is presented. Results indicate that it is possible to obtain around 50% reduction of perplexity by using mixture of several RNN LMs, compared to a state of the art backoff language model. Speech recognition experiments show around 18% reduction of word error rate on the Wall Street Journal task when comparing models trained on the same amount of data, and around 5% on the much harder NIST RT05 task, even when the backoff model is trained on much more data than the RNN LM. We provide ample empirical evidence to suggest that connectionist language models are superior to standard n-gram techniques, except their high computational (training) complexity.
更多查看译文
关键词
language modeling,recurrent neural networks,speech recognition
AI 理解论文
溯源树
样例
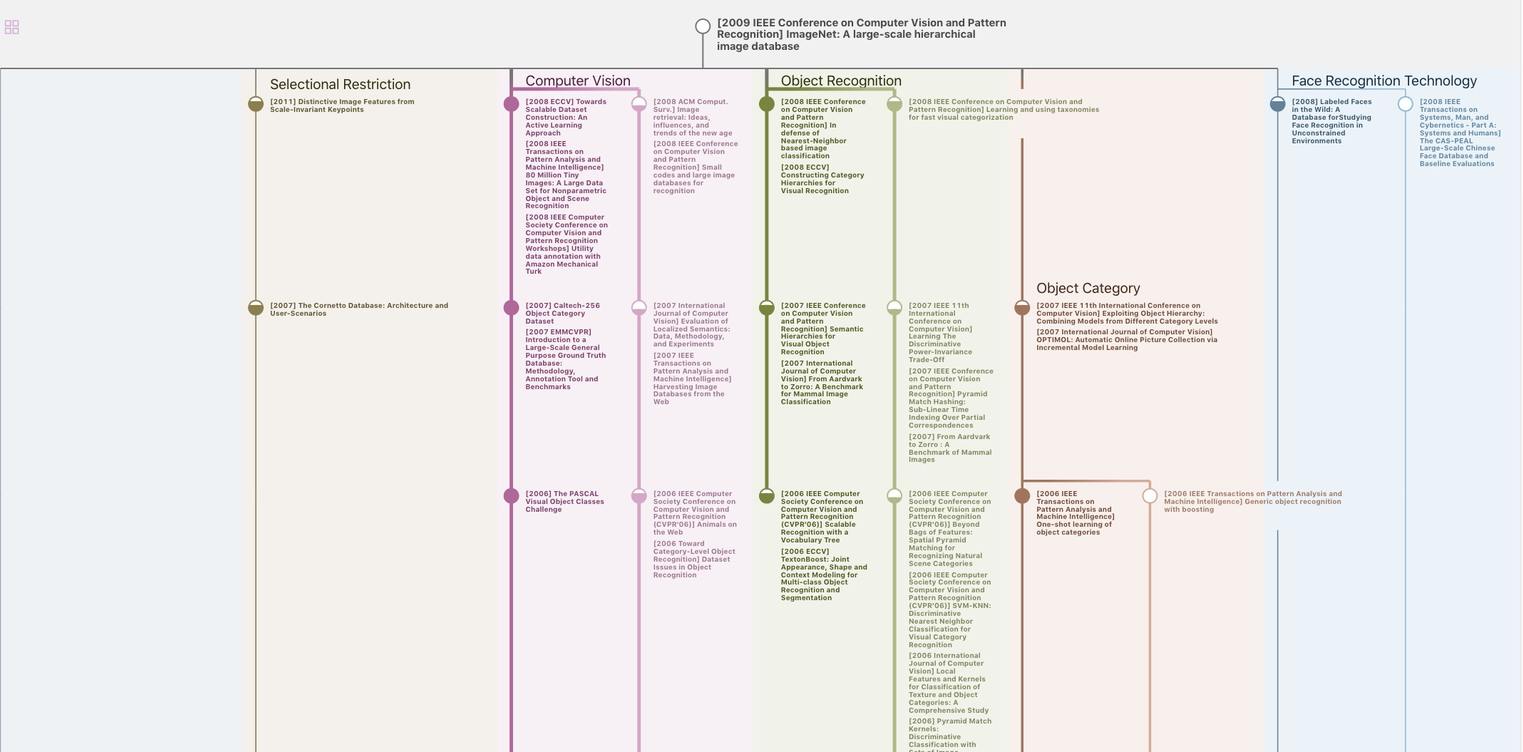
生成溯源树,研究论文发展脉络
Chat Paper
正在生成论文摘要