Evaluation of recommendations: rating-prediction and ranking.
RecSys '13: Seventh ACM Conference on Recommender Systems Hong Kong China October, 2013(2013)
摘要
The literature on recommender systems distinguishes typically between two broad categories of measuring recommendation accuracy: rating prediction, often quantified in terms of the root mean square error (RMSE), and ranking, measured in terms of metrics like precision and recall, among others. In this paper, we examine both approaches in detail, and find that the dominating difference lies instead in the training and test data considered: rating prediction is concerned with only the observed ratings, while ranking typically accounts for all items in the collection, whether the user has rated them or not. Furthermore, we show that predicting observed ratings, while popular in the literature, only solves a (small) part of the rating prediction task for any item in the collection, which is a common real-world problem. The reasons are selection bias in the data, combined with data sparsity. We show that the latter rating-prediction task involves the prediction task 'Who rated What' as a sub-problem, which can be cast as a classification or ranking problem. This suggests that solving the ranking problem is not only valuable by itself, but also for predicting the rating value of any item.
更多查看译文
AI 理解论文
溯源树
样例
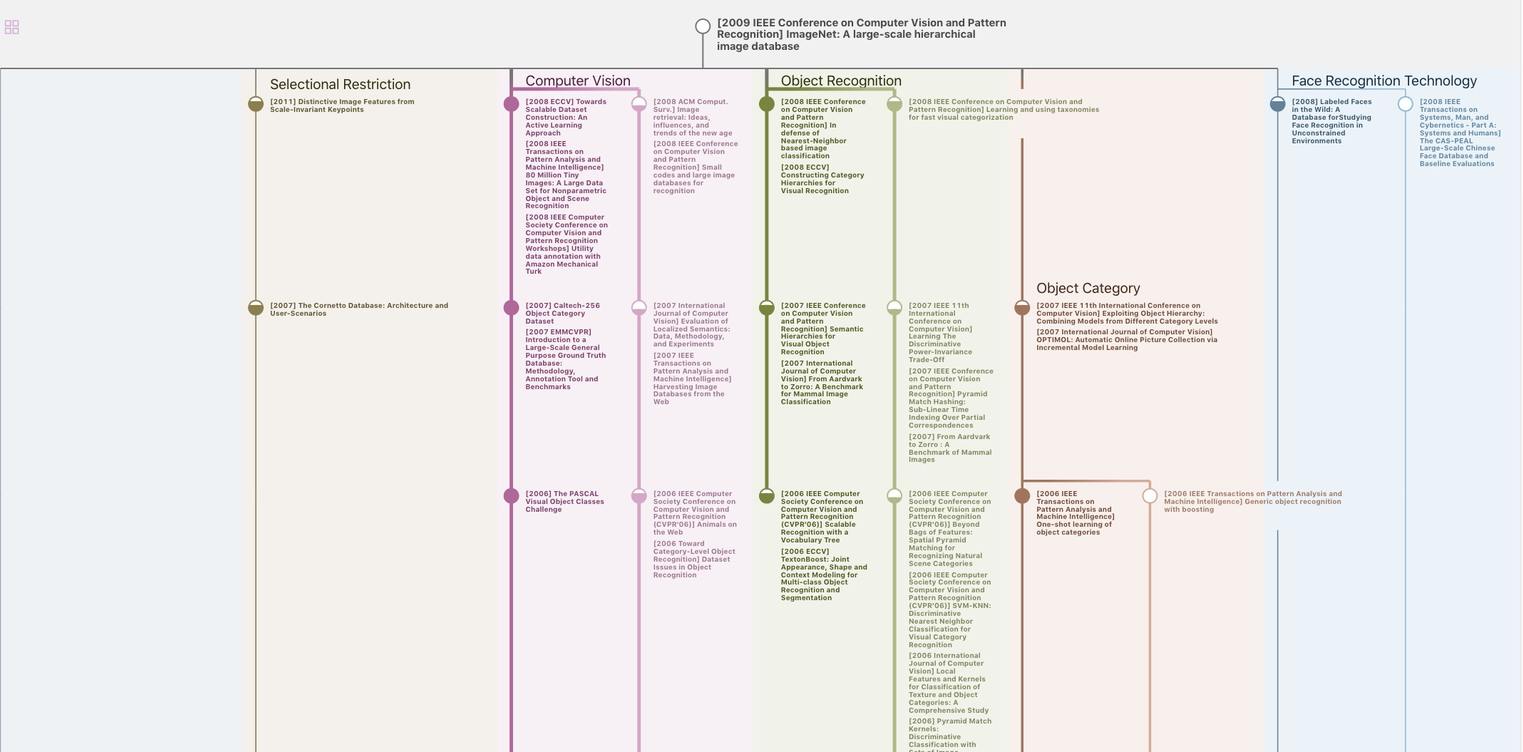
生成溯源树,研究论文发展脉络
Chat Paper
正在生成论文摘要