Demand Forecasting in Smart Grids
Bell Labs Technical Journal(2014)
摘要
Data analytics in smart grids can be leveraged to channel the data downpour from individual meters into knowledge valuable to electric power utilities and end-consumers. Short-term load forecasting STLF can address issues vital to a utility but it has traditionally been done mostly at system city or country level. In this case study, we exploit rich, multi-year, and high-frequency annotated data collected via a metering infrastructure to perform STLF on aggregates of power meters in a mid-sized city. For smart meter aggregates complemented with geo-specific weather data, we benchmark several state-of-the-art forecasting algorithms, including kernel methods for nonlinear regression, seasonal and temperature-adjusted auto-regressive models, exponential smoothing and state-space models. We show how STLF accuracy improves at larger meter aggregation at feeder, substation, and system-wide level. We provide an overview of our algorithms for load prediction and discuss system performance issues that impact real time STLF. © 2014 Alcatel-Lucent.
更多查看译文
关键词
meter reading,smart grids,forecasting,data analysis,supply and demand
AI 理解论文
溯源树
样例
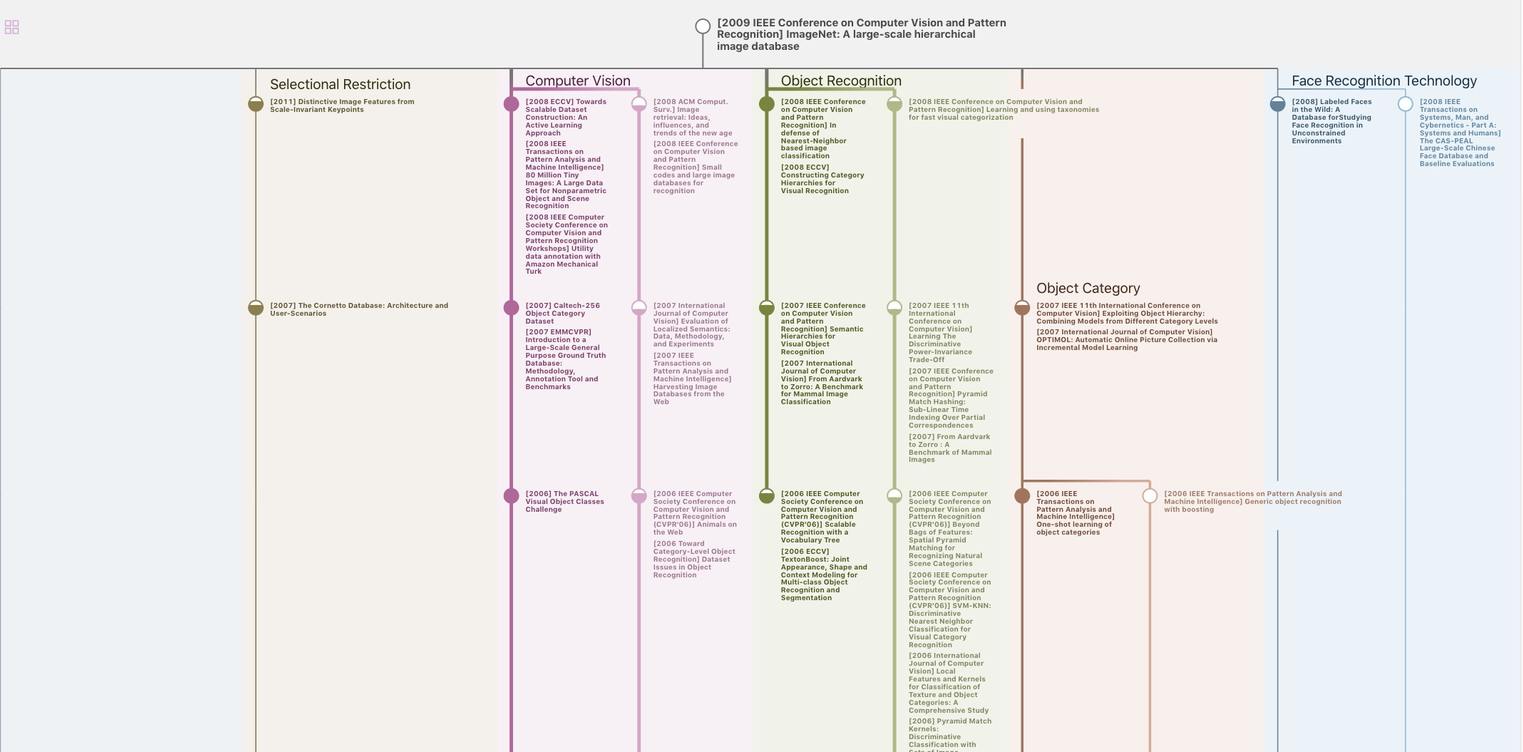
生成溯源树,研究论文发展脉络
Chat Paper
正在生成论文摘要