Better Word Alignments with Supervised ITG Models.
ACL '09: Proceedings of the Joint Conference of the 47th Annual Meeting of the ACL and the 4th International Joint Conference on Natural Language Processing of the AFNLP: Volume 2 - Volume 2(2009)
摘要
This work investigates supervised word alignment methods that exploit inversion transduction grammar (ITG) constraints. We consider maximum margin and conditional likelihood objectives, including the presentation of a new normal form grammar for canonicalizing derivations. Even for non-ITG sentence pairs, we show that it is possible learn ITG alignment models by simple relaxations of structured discriminative learning objectives. For efficiency, we describe a set of pruning techniques that together allow us to align sentences two orders of magnitude faster than naive bitext CKY parsing. Finally, we introduce many-to-one block alignment features, which significantly improve our ITG models. Altogether, our method results in the best reported AER numbers for Chinese-English and a performance improvement of 1.1 BLEU over GIZA++ alignments.
更多查看译文
关键词
ITG alignment model,ITG model,many-to-one block alignment feature,supervised word alignment method,inversion transduction grammar,new normal form grammar,AER number,CKY parsing,canonicalizing derivation,conditional likelihood objective,better word alignment,supervised ITG model
AI 理解论文
溯源树
样例
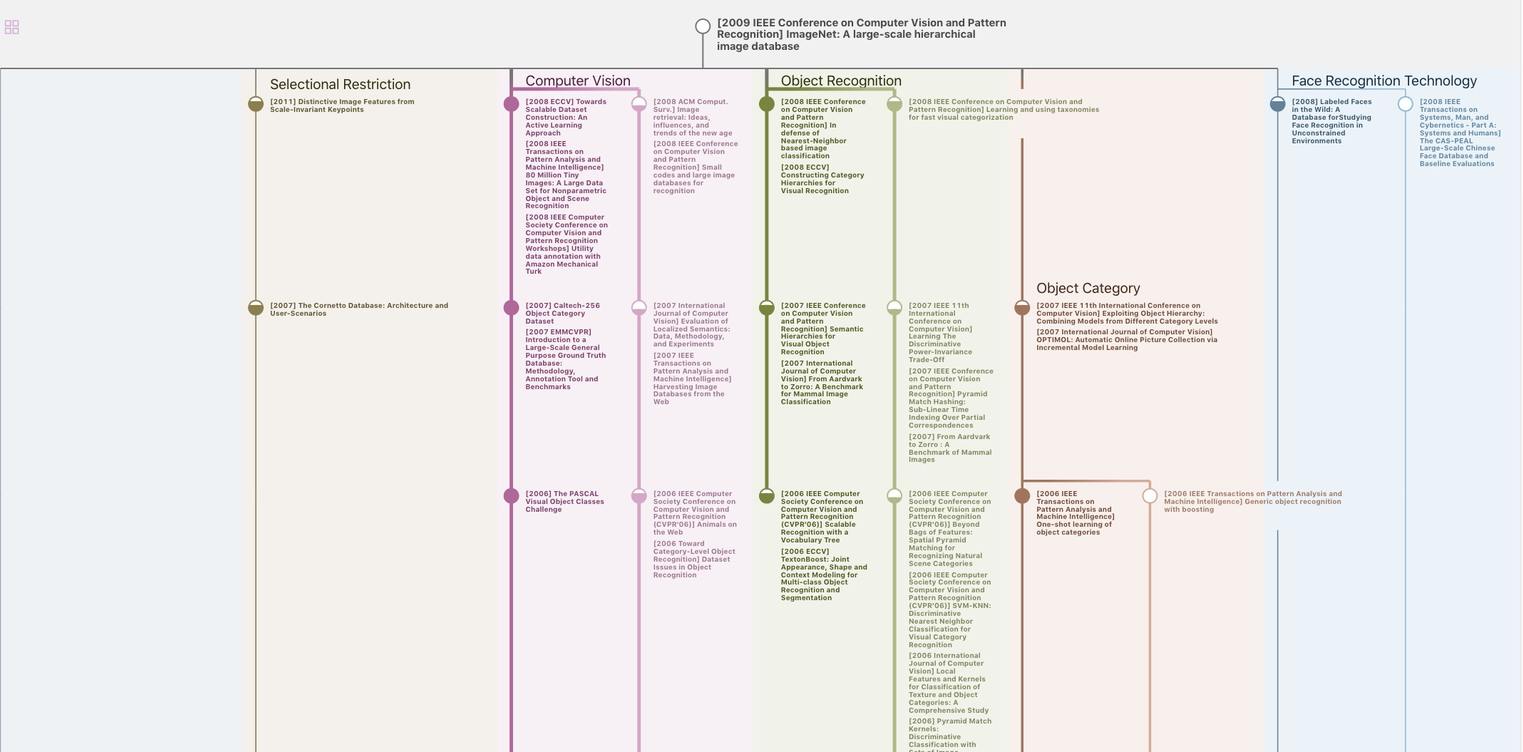
生成溯源树,研究论文发展脉络
Chat Paper
正在生成论文摘要