PLS in Data Mining and Data Integration
msra(2010)
摘要
Data mining by means of projection methods such as PLS (projection to latent structures), and their extensions is discussed.
The most common data analytical questions in data mining are covered, and illustrated with examples.
(a)
Clustering, i.e., finding and interpreting “natural” groups in the data
(b)
Classification and identification, e.g., biologically active compounds vs inactive
(c)
Quantitative relationships between different sets of variables, e.g., finding variables related to quality of a product, or
related to time, seasonal or/and geographical change
Sub-problems occurring in both (a) to (c) are discussed.
(1)
Identification of outliers and their aberrant data profiles
(2)
Finding the dominating variables and their joint relationships
(3)
Making predictions for new samples
The use of graphics for the contextual interpretation of results is emphasized.
With many variables and few observations (samples) – a common situation in data mining – the risk to obtain spurious models
is substantial. Spurious models look great for the training set data, but give miserable predictions for new samples. Hence,
the validation of the data analytical results is essential, and approaches for that are discussed.
更多查看译文
AI 理解论文
溯源树
样例
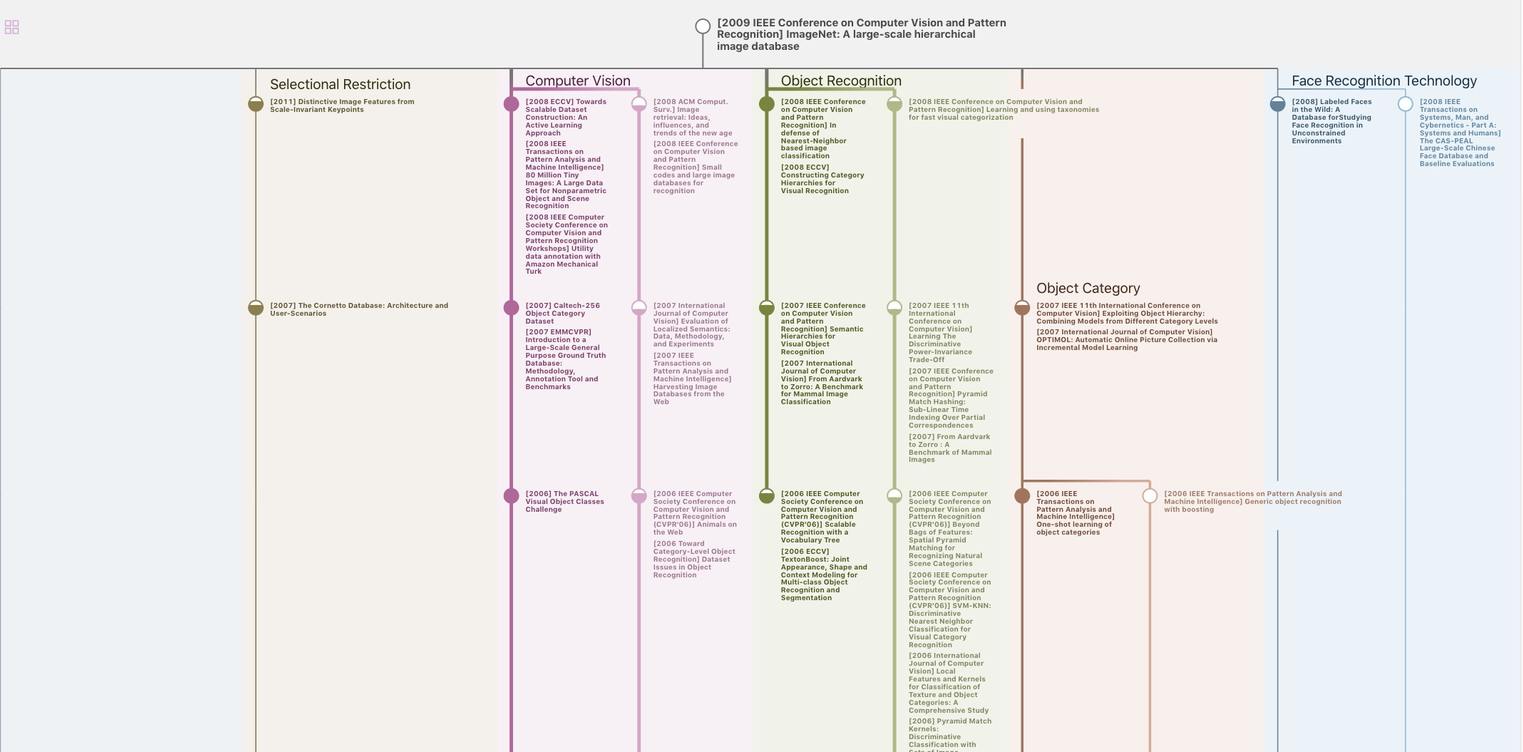
生成溯源树,研究论文发展脉络
Chat Paper
正在生成论文摘要