Joint Uncertainty Decoding for Noise Robust Subspace Gaussian Mixture Models
IEEE Transactions on Audio, Speech & Language Processing(2013)
摘要
Joint uncertainty decoding (JUD) is a model-based noise compensation technique for conventional Gaussian Mixture Model (GMM) based speech recognition systems. Unlike vector Taylor series (VTS) compensation which operates on the individual Gaussian components in an acoustic model, JUD clusters the Gaussian components into a smaller number of classes, sharing the compensation parameters for the set of Gaussians in a given class. This significantly reduces the computational cost. In this paper, we investigate noise compensation for subspace Gaussian mixture model (SGMM) based speech recognition systems using JUD. The total number of Gaussian components in an SGMM is typically very large. Therefore direct compensation of the individual Gaussian components, as performed by VTS, is computationally expensive. In this paper we show that JUD-based noise compensation can be successfully applied to SGMMs in a computationally efficient way. We evaluate the JUD/SGMM technique on the standard Aurora 4 corpus. Our experimental results indicate that the JUD/SGMM system results in lower word error rates compared with a conventional GMM system with either VTS-based or JUD-based noise compensation.
更多查看译文
关键词
noise robust subspace gaussian mixture models,compensation parameters,gaussian components,gaussian processes,speech recognition,standard aurora 4 corpus,acoustic model,model-based noise compensation technique,word error rates,aurora 4,noise robust asr,subspace gaussian mixture model,vts compensation,jud-sgmm technique,speech coding,compensation,joint uncertainty decoding,gmm based speech recognition systems,gaussian noise,decoding,vector taylor series
AI 理解论文
溯源树
样例
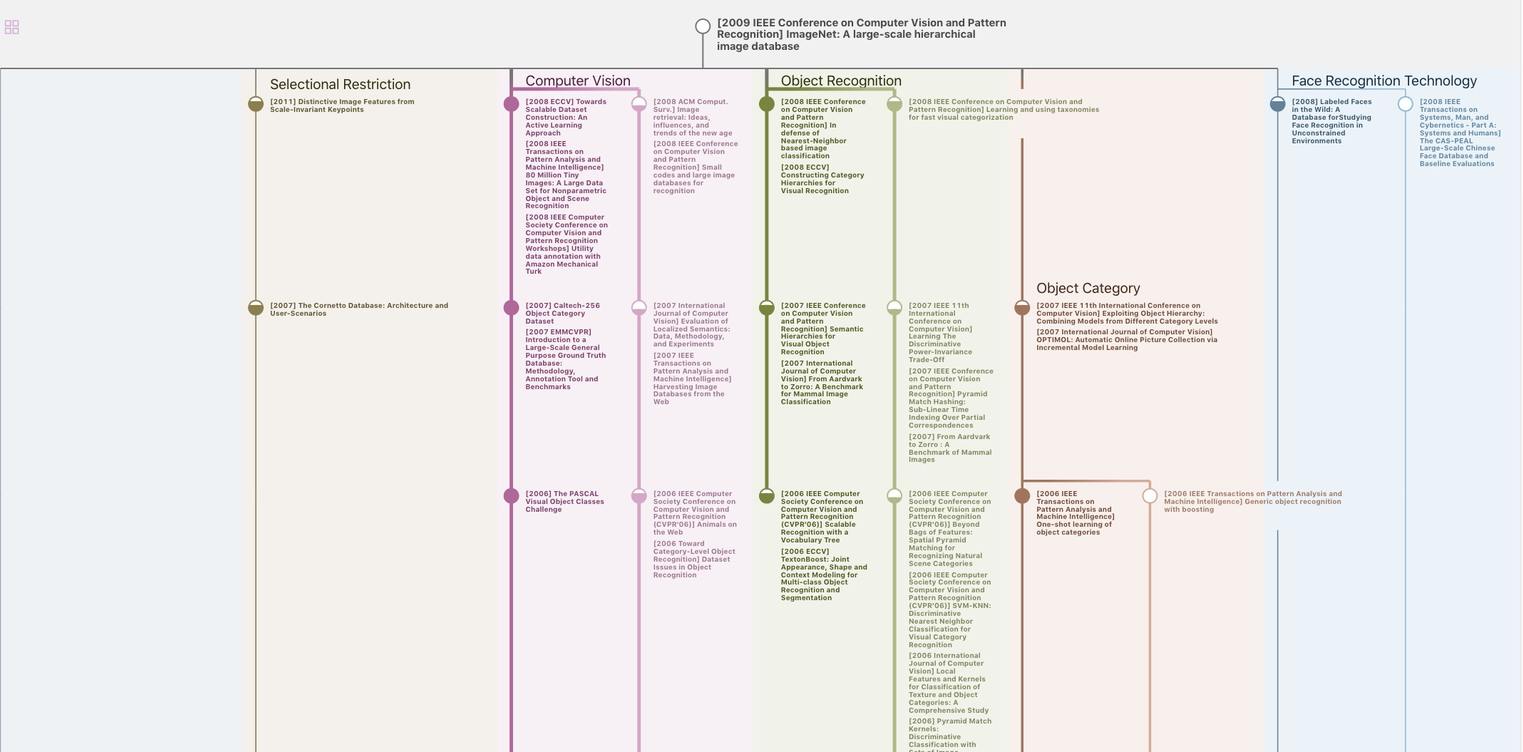
生成溯源树,研究论文发展脉络
Chat Paper
正在生成论文摘要