Integrating Remote Sensing Data and CNN-LSTM-Attention Techniques for Improved Forest Stock Volume Estimation: A Comprehensive Analysis of Baishanzu Forest Park, China
Remote Sensing(2024)
摘要
Forest stock volume is the main factor to evaluate forest carbon sink level. At present, the combination of multi-source remote sensing and non-parametric models has been widely used in FSV estimation. However, the biodiversity of natural forests is complex, and the response of the spatial information of remote sensing images to FSV is significantly reduced, which seriously affects the accuracy of FSV estimation. To address this challenge, this paper takes China’s Baishanzu Forest Park with representative characteristics of natural forests as the research object, integrates the forest survey data, SRTM data, and Landsat 8 images of Baishanzu Forest Park, constructs a time series dataset based on survey time, and establishes an FSV estimation model based on the CNN-LSTM-Attention algorithm. The model uses the convolutional neural network to extract the spatial features of remote sensing images, uses the LSTM to capture the time-varying characteristics of FSV, captures the feature variables with a high response to FSV through the attention mechanism, and finally completes the prediction of FSV. The experimental results show that some features (e.g., texture, elevation, etc.) of the dataset based on multi-source data feature variables are more effective in FSV estimation than spectral features. Compared with the existing models such as MLR and RF, the proposed model achieved higher accuracy in the study area (R2 = 0.8463, rMSE = 26.73 m3/ha, MAE = 16.47 m3/ha).
更多查看译文
关键词
forest stock volume,remote sensing (RS),Pearson correlation analysis,convolutional neural network (CNN),LSTM,attention mechanism
AI 理解论文
溯源树
样例
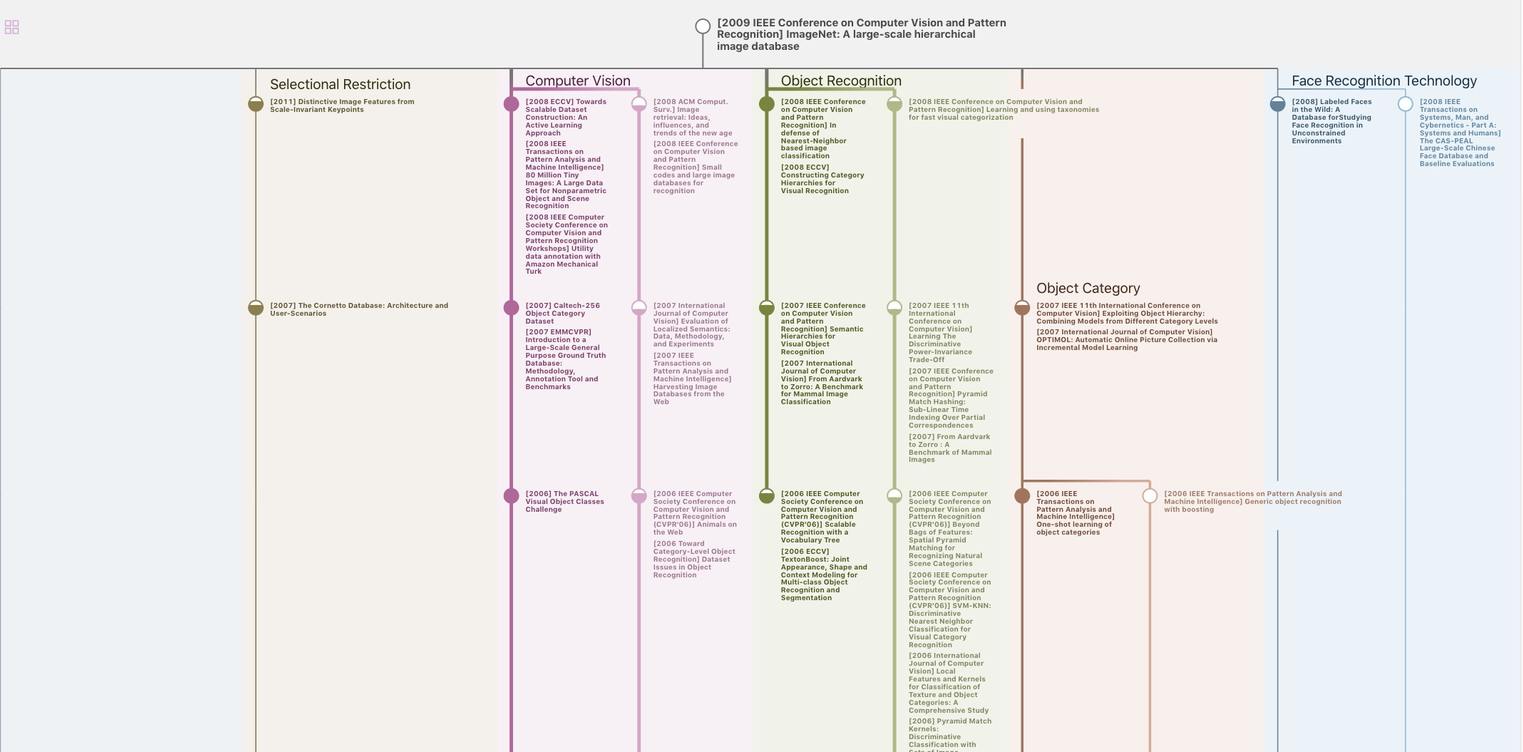
生成溯源树,研究论文发展脉络
Chat Paper
正在生成论文摘要