Selection of Subsets of Ordered Features in Machine Learning
Trans. MLDM(2009)
摘要
The new approach of relevant feature selection in machine learning is proposed for the case of ordered features. Feature selection and regularization of decision rule are combined in a single procedure. The selection of features is realized by introducing weight coefficients, characterizing degree of relevance of respective feature. A priori information about feature ordering is taken into account in the form of quadratic penalty or in the form of absolute value penalty on the difference of weight coefficients of neighboring features. Study of a penalty function in the form of absolute value shows computational complexity of such formulation. The effective method of solution is proposed. The brief survey of author's early papers, the mathematical frameworks, and experimental results are provided.
更多查看译文
关键词
feature selection,brief survey,neighboring feature,absolute value penalty,weight coefficient,absolute value,penalty function,respective feature,ordered features,support vector machines,quadratic penalty,relevant feature selection,machine learning,parametric dynamic programming,regularization of training,decision rule,computational complexity,support vector machine
AI 理解论文
溯源树
样例
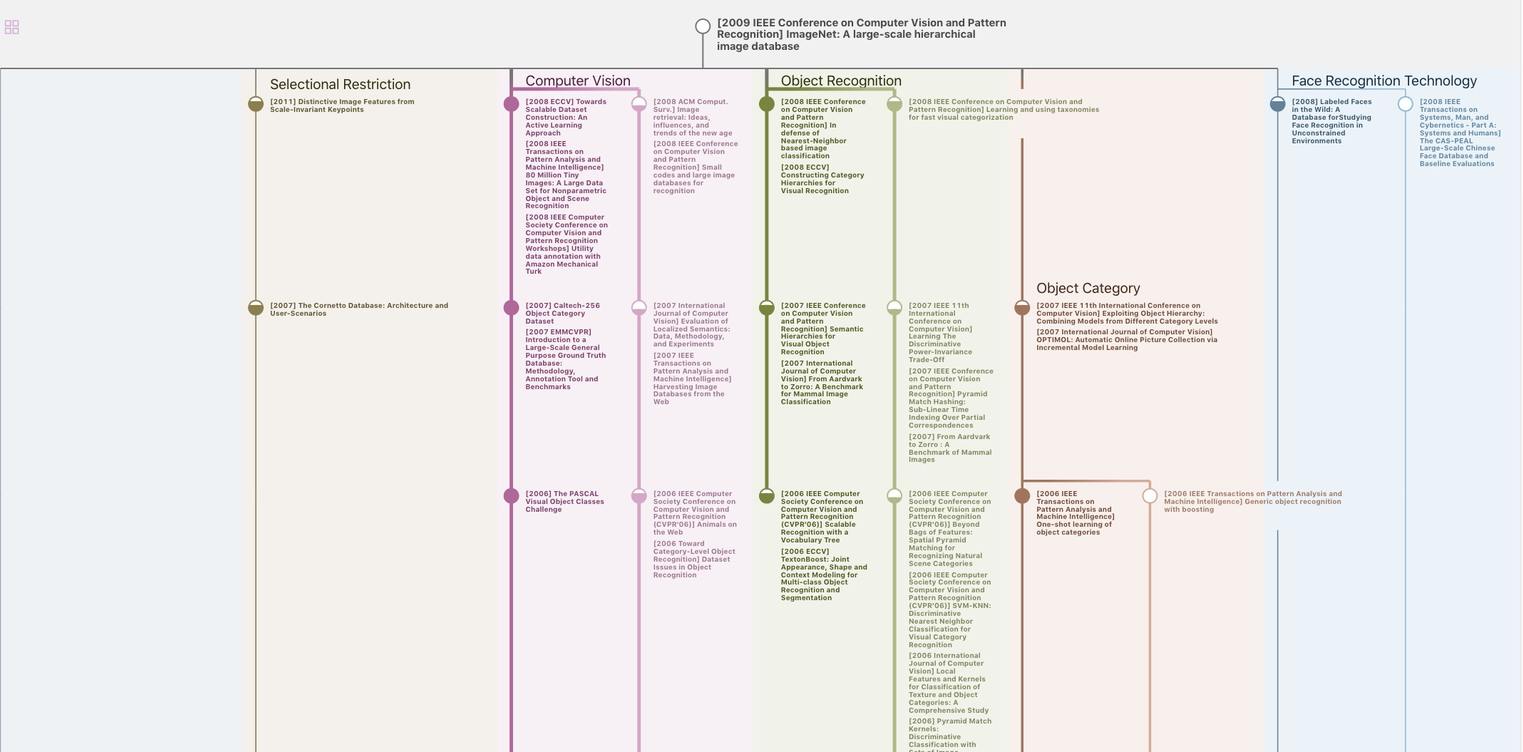
生成溯源树,研究论文发展脉络
Chat Paper
正在生成论文摘要