Spatially distributed modeling of the long-term carbon balance of a boreal landscape
Ecological Modelling(2011)
摘要
Spatially and temporally distributed information on the sizes of biomass carbon (C) pools (BCPs) and soil C pools (SCPs) is vital for improving our understanding of biosphere–atmosphere C fluxes. Because the sizes of C pools result from the integrated effects of primary production, age-effects, changes in climate, atmospheric CO 2 concentration, N deposition, and disturbances, a modeling scheme that interactively considers these processes is important. We used the InTEC model, driven by various spatio-temporal datasets to simulate the long-term C-balance in a boreal landscape in eastern Canada. Our results suggested that in this boreal landscape, mature coniferous stands had stabilized their productivity and fluctuated as a weak C-sink or C-source depending on the interannual variations in hydrometeorological factors. Disturbed deciduous stands were larger C-sinks (NEP 2004 = 150 gC m −2 yr −1 ) than undisturbed coniferous stands (e.g. NEP 2004 = 8 gC m −2 yr −1 ). Wetlands had lower NPP but showed temporally consistent C accumulation patterns. The simulated spatio-temporal patterns of BCPs and SCPs were unique and reflected the integrated effects of climate, plant growth and atmospheric chemistry besides the inherent properties of the C pool themselves. The simulated BCPs and SCPs generally compared well with the biometric estimates (BCPs: r = 0.86, SCPs: r = 0.84). The largest BCP biases were found in recently disturbed stands and the largest SCP biases were seen in locations where moss necro-masses were abundant. Reconstructing C pools and C fluxes in the ecosystem in such a spatio-temporal manner could help reduce the uncertainties in our understanding of terrestrial C-cycle. Key words Long-term C modeling InTEC Boreal ecosystem Climate change Changes in atmospheric chemistry Disturbance Biomass C pools [BCPs] Soil C pools [SCPs] 1 Introduction Studies have shown that the effects of global warming may change the status of the terrestrial biosphere from a weak carbon (C) sink to a source by the middle of this century due to the release of soil C stocks to the atmosphere ( Cox et al., 2000 ). Small variations in the C fluxes such as gross primary production (GPP) and total ecosystem respiration (TER) can result in large variations in the net ecosystem productivity (NEP). This is true especially in mature forest stands that have considerable quantities of C stocks in the plant and soil. For the ease of understanding the terrestrial C cycle, it is usually assumed that the C stocks are comprised of several conceptual C pools that have unique biophysical characteristics ( Shibu et al., 2006; Porporato et al., 2003 ). On a long term basis, the quantities of biomass C pools (BCPs) and soil C pools (SCPs) in an ecosystem depend on the disturbance history ( Sun et al., 2004 ), atmospheric CO 2 concentration ( Chen et al., 2000; Johnson et al., 2000; Alexandrov et al., 2003 ), nitrogen (N) deposition ( Magnani et al., 2007; Turunen et al., 2004 ), stand age ( Gower et al., 1996; Peltoniemi et al., 2004 ), soil texture ( Torn et al., 1997 ), drainage class ( Rapalee et al., 1998 ), and climate ( Apps et al., 2000; Black et al., 2000; Griffis et al., 2003 ). Temporally precise C-flux measurements are available for many locations in the world that have advanced our understanding of biosphere–atmosphere C fluxes (e.g. FLUXNET, Baldocchi, 2008 ). However, landscape-scale generalizations of C-fluxes need modeling efforts addressing spatio-temporal variability. Currently there are many inconsistencies among the models even though the fundamental mechanisms that describe the ecosystem processes are similar ( Franks et al., 1997; Schulz et al., 2001; Raupach et al., 2005; Mitchell et al., 2009 ). One of the major issues lies in how well, within the models, the C pools are [1] parameterized, [2] initialized and [3] altered. Because biogeochemical transformations are assumed to have first-order kinetics in most models ( Shibu et al., 2006; Porporato et al., 2003 ), accurate quantities of C pools are essential to simulate such processes. Long-term biogeochemical processes can thus govern the sizes of C pools and therefore, the current C-fluxes. Conventionally in distributed modeling approaches, remotely sensed information have been used to estimate C-fluxes at the regional (e.g. Turner et al., 2006; Coops et al., 2007; Potter et al., 2001 ) and global scales (e.g. MODIS-based global GPP product, MOD17A2). However, these efforts are confined to C-fluxes such as GPP or NPP and not NEP, because of the difficulty in estimating the C pools in the soil and plant that determines TER. NEP is the ideal indicator of the net C balance ( Janssens et al., 2003; Govind et al., 2009b; Lovett et al., 2006 ). Some studies have demonstrated mapping of daytime NEP fluxes at the landscape by integrating ground data and remotely sensed information ( Hassan et al., 2006; Kelly et al., 2002 ). However, such approaches are possible only when and where GPP fluxes are larger than TER fluxes. While aboveground biomass (AGB) can be estimated using techniques such as LIDAR (e.g. Popescu, 2007 ) and RADAR (e.g. Austin et al., 2003 ) and are operational, they are currently not being applied at large scales. Nevertheless, AGB gives information on only the aboveground BCPs. SCPs are more important and uncertain, considering their critical role in heterotrophic respiration ( R H ) ( Bond-Lamberty and Thomson, 2010 ). Many models initialize the sizes of C pools by running them for centuries until the C pool sizes are stabilized (e.g. RHESSys, Tague and Band (2004) ; CTEM, Arora and Boer (2003) ; ORCHIDEE, le Maire et al. (2010) . Effects of disturbances and changes in climate and atmospheric chemistry are often simplified in such spin-ups, resulting in potentially unrealistic C pool sizes. Furthermore, intensive point-scale models might have difficulties to capture the spatial heterogeneity necessary for landscape-scale calculations because of computational challenges (e.g. Grant et al., 2009 ). Although Bayseian inversion-based approach is an alternative method, this faces large uncertainties regarding parameter identifiability and equifinality ( Luo et al., 2009 ). Considering this research gap, it is important to study the dynamics of C pools and fluxes in the soil and plant under the effects of climate, disturbance (natural or anthropogenic), atmospheric chemistry and the associated feedback relationships. In this study, we present a long-term (1920–2005) simulation of the C-balance of a boreal forest landscape in a spatially explicit manner using various spatio-temporal datasets and an ecosystem model. Further, we investigate the dynamics of various C pools and C-fluxes in space and time. We employed the Integrated Terrestrial Ecosystem Carbon Balance Model (InTEC, Chen et al., 2000 ) to address these objectives. InTEC has been used in several studies that deal with the large-scale modeling of C-fluxes (e.g. Canada, Chen et al., 2003; Ju and Chen, 2005; Ju et al., 2006; Ju and Chen, 2008 ), (China, Wang et al., 2007; Shao et al., 2007 ). Here we demonstrate for the first time InTEC's ability to simulate the dynamics of various ecosystem C pools in a spatially and temporally explicit manner, under changes in climate, atmospheric chemistry and disturbances. 2 Model description InTEC employs a quasi-process-based approach to simulate the long-term C balance of an ecosystem. Biogeochemical processes are described based on the dynamics of various C pools subjected to abiotic and biotic factors. Primary production derived C alters the sizes of the four BCPs which are demarcated as [1] Wood ( C w ) [2] Foliage ( C l ), [3] Coarse roots ( C cr ) and [4] Fine Roots ( C fr ). These BCPs upon mortality, directly or indirectly alter the sizes of the nine SCPs, i.e., [1] Surface Structural Litter C pool ( C ssd ), [2] Fine root Structural Litter C pool Structural Litter C pool ( C fsd ), [3] Coarse-woody Litter C pool ( C cd ), [4] Metabolites in Surface Litter C pool ( C smd ), [5] Metabolites in Soil Litter C pool ( C fmd ), [6] Surface Microbial C pool ( C sm ), [7] Soil Microbial C pool ( C m ), [8] Slow C pool ( C s ), and [9] Passive C pool ( C p ). These SCPs undergo abiotic and biotic transformations and change their sizes and also produce C fluxes due to R H . Because InTEC operates at large spatio-temporal scales, rigorous mathematical strategies are adopted to upscale processes in space (canopy scale) and time (annual). Effects of climate, atmospheric chemistry and disturbances are considered as factors that modulate the stand productivity and hence the net C balance. C-fluxes in the form of dissolved organic carbon (DOC) and methane flux are not considered in the C-balance. InTEC assumes a dynamic equilibrium between NPP and R H during those periods in the ecological history when no information on stand age is available (e.g. time before the modeling period or the time before the latest disturbance event). Under this equilibrium condition, a stand is assumed to be at the “equilibrium age”. The NPP value that is in dynamic equilibrium with R H is defined as the NPP at equilibrium age (hereafter denoted as NPP 0 ). If we know the NPP 0 associated with each pixel in the modeling domain, NPP values of the subsequent years (NPP i ) can be progressively calculated after considering the effects of disturbance and non-disturbance factors as shown below. (1) NPP i = NPP 0 × ϕ dist , i × ϕ non-dist , i In the above equation, the term ϕ i = [ F ( a i )/ F ( a 0 )] represents the disturbance factor affecting the C balance of a forest stand because of changes in the stand age of perennial vegetation and therefore, its productivity. This factor is assumed as a normalized stand productivity at ages a 0 (the starting year) and a i (the i th year), respectively. This means that in order to simulate the disturbance effect, we need information on (1) an NPP–age relationship and (2) and a current stand age map. In this study, we used the generalized form of the NPP–age relationship developed by Chen et al. (2002) . This NPP–age relationship was obtained by fitting a Weibull distribution over the observed dendrochronological data. The non-disturbance factors that govern the stand productivity include the effects of climate, CO 2 fertilization and N availability. The integrated effects of these non-disturbance factors on the C balance are collectively represented by the term, ϕ non-dist , i = Π j = 1 i 2 + χ ( j ) 2 − χ ( j ) calculated in a spatially and temporally explicit manner as a function of climate, CO 2 fertilization and N deposition. The method to compute χ can be found in Chen et al. (2000) and Ju et al., 2007 . It is possible to calculate NPP 0 if we have reliable estimates of NPP in a reference year (hereafter denoted as NPP ref ). The reference year is usually a recent year (e.g. 2004 in this study) in which we have greater confidence in our understanding of the ecosystem. This is usually achieved by relying on multiple approaches such as remote sensing, biometric measurements, eddy covariance data, and intensive process-based models. Using [1] an NPP ref map, [2] information on the disturbance-type and disturbance-time, [3] information on various non-disturbance factors and [4] NPP–age relationship, the NPP 0 for each pixel is estimated by satisfying the condition that the simulated NPP i in the reference year (based on Eq. (1)) match with the NPP ref (from the input map). This is an iterative procedure wherein the pixel value of NPP 0 is tuned in each step until there is only negligible difference between the simulated NPP i =ref. year and the inputted NPP ref ( Ju and Chen, 2005, 2008 ). The whole process is conceptually represented in Fig. 1 a . Therefore, the accuracy of the NPP ref.year is crucial to reliably simulate the long-term C balance. In the process of estimating the optimal NPP 0 for each pixel, the sizes of C pools at the equilibrium age (e.g. in the pre-simulation period or for the time before the last disturbance) is also estimated. This is achieved by solving a set of differential equations that consider the interactions among the C pools under the equilibrium condition (i.e. NPP 0 = R H ) see Chen et al., 2003 ). Further, the sizes of BCPs and SCPs are updated on an annual basis as shown below (2) C x i = C x i − 1 + Δ C x i where, C x,i and C x , i −1 are the sizes of the C pool x in the previous ( i − 1) and the current ( i ) years, respectively. Δ C x , i is the change in the pool x in the year i . The procedure to calculate Δ C x , i for each C pool is different and depends on the integrated effects of various abiotic and biotic factors and the sizes of the precursor C pools as shown in the appendix. In general, the rates of change in BCPs are calculated as shown below. (3) Δ C j , i = f j ⋅ NPP i − k j ⋅ C j , i 1 + k j where f j refers to the C allocation rate to the j th BCP, and k j is the mortality rate of the j th BCP in the year i . The dynamics of SCPs are more complex and are calculated as shown below, (4) Δ C j , i = ∑ l = 1 n k l , j , i . C l , ( i − 1 ) − k j . C j , ( i − 1 ) 1 + k j where k l,j is the transfer rate of C from SCP l to SCP j , n is number of SCP transferring C to SCP j , and k j is the decomposition rate of SCP j . In the processes of decomposition and allocation of C between SCPs, and release C to the atmosphere, sizes of each SCP alter. Further, R H is calculated as the sum of all the atmospheric C-fluxes from individual SCPs as shown in Eq. (5) . The nature of SCP decomposition is conceptualized as a function of the existing SCP sizes and various abiotic factors (soil temperature, soil moisture and soil texture) that modulate the decomposition of each SCPs in a unique manner. (5) R H i = ∑ j = 1 9 k j . C j The actual decomposition rates of SCPs ( k j ) are determined by constraining SCP-specific potential decomposition rates ( K j ). Scalars that correspond to the factors such as soil temperature, moisture, soil texture, and SCP lignin contents ( Chen et al., 2003; Ju et al., 2007 ) constrain K j . The historical trend in soil moisture is calculated in a simplified manner by considering the annual precipitation, evapotranspiration (ET) and soil hydraulic conditions (a simplified bucket approach). During the process of SCP decomposition, N mineralization and immobilization alter the C:N ratios of each SCP, except for C m and C sm (living forms of SCPs). From the reconstructed annual NPP i ; NEP i and net biome productivity (NBP i ) are obtained by deducting the C-fluxes due to R H and disturbance (in the absence of disturbance, NBP = NEP), respectively ( Fig. 1 b). Table 1 shows the description of various conceptual C pools (BCPs and SCPs), factors governing their dynamics, and their fates within the InTEC domain. More details on these aspects can be found in Chen et al. (2003) . 3 Site description The long-term simulation of C-balance presented in this paper was conducted on a ∼40 km 2 boreal landscape located in north-central Quebec, Canada ( Fig. 2 ). This region has a mean annual temperature of ∼0 °C and a mean annual precipitation of ∼960 mm ( Environment Canada, 2006 ). The soil is a ferro-humic to humic podzol, covered by an organic layer with an average depth of ∼30 cm. In humid areas, the soil is mainly organic with an average depth of 125 cm ( Giasson et al., 2006 ). The soil drainage is fair and the water table is usually below 50 cm. Vegetation in this boreal landscape is diverse. In the coniferous stands, the overstory is predominated by black spruce ( Picea mariana ) whereas in the deciduous and mixed stands, aspen ( Poplus sp.) or birch ( Betula sp ) are commonly found. Sporadically, jack pine ( Pinus banksiana Lamb.), tamarack ( Larix laricina ) and balsam fir ( Abies balsamea ) also occur. In general, the understory is dominated by labrador tea ( Ledum groenlandicum) and the forest floor consists of a moss layer ( Bergeron et al., 2007 ), with feather moss and lichens in moderately dry locations and Sphagum sp. in relatively wetter locations. The Eastern Old Black Spruce (EOBS) site of the Canadian Carbon Program (CCP) is located at 49.69° N and 74.342° W in the southwestern part of this boreal watershed. The eddy covariance (EC) technique is being used at the EOBS site to record near-continuous 30-min CO 2 , water and energy fluxes since June 2003. Predominant wind comes from the S-SW, both during day and night. The fetch extends to 500 m in all directions. Using an inverse Lagrangian model, Kljun et al. (2004) conducted a flux footprint analysis and estimated that the site's fetch contributed at least 90% of the measured flux in the dominant wind directions. Therefore, we used a circular area subtended by a 500 m radius centered on the tower as “the footprint” for our data analysis, similar to Bergeron et al. (2007) . Undisturbed mature black spruce stands dominate in the footprint region, with the stand age ranging from 90 to 120 years. 4 Preparation of data sets Several spatially-explicit datasets were required to run the InTEC model. These datasets included reference year maps of land-cover, leaf are index (LAI), soil texture, current stand age, NPP ref , ET ref and N deposition. For the year 2004, we had a greater confidence in our understanding of the ecosystem due to intensive measurements and modeling activities. Therefore, we fixed 2004 as the reference year in this study. Table 2 gives a general description of the spatial and temporal datasets used in this study and Fig. 3 shows some of the spatial datasets. All the datasets have a spatial resolution of 25 m in the UTM Zone 18 N projection system. 5 Measurement of ecosystem C-indicators for model validation 5.1 Measurement of ecosystem C fluxes EC measurements made at EOBS site were used to compute the C fluxes. The flux data used in this study were quality controlled, gap-filled, and partitioned for the flux subcomponents by Bergeron et al. (2007) using a standard CCP algorithm developed by Barr et al. (2004) . Daytime TER, missing nighttime and cold season TER were estimated using an empirical function that relates TER and temperature. GPP was calculated from the NEP and TER estimates. These half-hourly data sets were directly obtained from the Data Information System of the Canadian Carbon Program ( http://fluxnet.ccrp.ec.gc.ca/ ) and were used for validation of the simulated C fluxes after converting to annual estimates. 5.2 Biometric measurement of ecosystem C-stocks In 2003, we measured the C-stocks in the soil and plant at 12 inventory plots (each 400 m 2 ) spread around the EC tower at EOBS ( Fig. 2 ). The location of the inventory plots were determined using aerial photographs and GIS, employing a randomized sampling scheme such that all major stand-type in the EC-tower footprint region was represented. In each plot, complete tree inventories were made by measuring the diameter at breast height (DBH). Further, C contents in AGB were computed using species-specific allometric equations ( Lambert et al., 2005 ), assuming the C fraction of biomass (CFB) to be 0.5 Soil C stocks were also estimated in the same year at four of the 12 inventory plots. For a representative spatial coverage, four to eight points were sampled within each inventory plot with a 30 cm sampling depth. Soil samples were used to estimate the C-content in the organic and mineral layers in the laboratory using a LECO CR12 Total C analyzer. The soil C stocks thus analyzed did not include C contained in the living root biomass. We used these measured soil C-stock data to validate the simulated SCPs for the year 2003. 5.3 Measurements of PAI as an indicator of AGB Plant area index (PAI), implies the amount of foliage and wood biomasses. PAI was estimated using a combination of Digital Hemispherical Photography and a Plant Canopy Analyzer (LAI-2000) in the growing season of 2003 ( Chen et al., 2006 ). We took PAI measurements at 10 m intervals along a 400 m long westbound transect starting from the EOBS tower and also at all the inventory plots mentioned above. We used these PAI estimates to test the accuracy of the simulated aboveground BCPs by comparing it with the simulated AGB in 2003. 6 Results and discussion 6.1 Temporal dynamics of C fluxes, AGB and their validation Fig. 4 shows the simulated time series of the three main ecological indicators i.e. NPP, NBP and AGB (panels D, E and F) vis-a-vis the hydroclimatic factors (panels A–C). We present the simulations for two locations that underwent distinct disturbance regimes on this boreal landscape: (1) an undisturbed location (EOBS) and (2) a disturbed site (clear-cut in 1963). Time series of the modeled NPP and AGB suggested that productivity of the EOBS stand has stabilized in the recent years while it is still increasing at the disturbed site. In the recent years, the magnitude of NEP in the disturbed site was higher (150 gC m −2 yr −1 ) and showed and increasing trend in comparison to the undisturbed EOBS site where the NEP was near C neutrality (8 gC m −2 yr −1 ). The AGB was higher (∼4.5 kgC m −2 ) but reached a saturation in the undisturbed site while in the disturbed site it was lower but showed (∼2.5 kgC m −2 ) an increasing trend. The difference seen on the dynamics of C indicators among the two disturbance regimes can be linked to stand ages. This age effects on productivity and its cascading effects on the overall C-balance is important for modeling the long-term C cycle. Many studies have demonstrated the age effects in boreal forest productivity using biometric approaches (e.g. Amiro et al., 2010; Coursolle et al., 2006; Gower et al., 1997; Ryan et al., 1997 ). The stand ages at EOBS was ∼120 years and our results were consistent with some of the chrononosequence-based measurements made by Bond-Lamberty et al., 2004 and Wang et al., 2003 in mature black spruce ecosystems in central Canada. Panels D–F of Fig. 4 show how the model simulates the effects of disturbance and regrowth and the consequent C balance following a disturbance event. Even though stand regeneration started quickly following the disturbance, the ecosystem remained as a C source for at least 20–25 years before turning into a C sink. This was because the SCPs continued to decompose at higher rates releasing soil C into the atmosphere through TER fluxes rather than photosynthetic C assimilation. These results imply that almost 2 decades are required for the disturbed system to reach a C-neutral condition. Validation of the simulated NPP is often difficult because of the absence of temporally and spatially fine-scale NPP measurements. Assuming that measured NPP was 47% (after Landsberg and Waring, 1997 ) of the GPP estimated using the EC technique; we compared the simulated annual NPP with the annual NPP derived from the GPP estimates reported by Bergeron et al. (2008) . The simulated annual NPP in 2004 (288.1 gC m −2 yr −1 ) and EC-derived annual NPP in 2004 (278.2 gC m −2 yr −1 i.e. 47% of GPP = 592 gC m −2 yr −1 ) at the EOBS EC-tower footprint region was in reasonable agreement. This good agreement between the simulated and the measured NPP in a recent year occurred because within InTEC there is a mechanism to equate the simulated NPP i =ref with the NPP ref as described in Section 2. Nevertheless, our NPP estimates were consistent with the field measurements taken in a similar ecosystem in northern Manitoba, Canada ( Bond-Lamberty et al., 2004; Wang et al., 2003 ). The year 2005 was a prospective simulation year, i.e. a year beyond the reference year. In 2005, the simulated NPP at the EOBS EC-tower footprint region was 335 gC m −2 yr −1 whereas EC-derived NPP was 320 gC m −2 yr −1 (i.e. 47% of annual GPP = 680 ± 4 gC m −2 yr −1 ). It should be noted that the 2005 primary productivity was higher than 2004, consistent with another process-intensive simulation conducted by Govind et al. (2009a) at a daily time step. A higher GPP was observed because in 2005, unlike 2004, the ecosystem was relatively warmer and drier with a longer length of growing season. From late June until late September, soil moisture status remained below the field capacity, but still above the permanent wilting point. This created conducive growth conditions. In this humid boreal ecosystem, water scarcity is not a limiting factor for plant growth. Instead, excessive soil moisture (beyond the field capacity) is a plant stress factor. Previous studies at this site demonstrated that, due to the perhumid nature of this ecosystem, soil drying enhanced plant productivity primarily due to increased stomatal conductance ( Govind et al., 2010 ). Also, drier but optimal soil hydrothermal conditions increased N availability due to accelerated decomposition of SCPs. This explains the higher primary production in 2005, which was drier and warmer, compared to 2004, which was wetter and cooler. For the year 2004, InTEC simulated an average NEP value of 8 gC m −2 yr −1 for the EC-tower footprint region as against 6±12 gC m −2 yr −1 measured by the EC technique ( Bergeron et al., 2008 ). However in 2005, the simulated NEP was 30 gC m −2 yr −1 , whereas the EC-based estimate was 0 ± 3 gC m −2 yr −1 ( Bergeron et al., 2008 ). This discrepancy arose mainly due to an underestimation of TER fluxes (mainly due to R H ) by the model. Because 2005 was relatively dry, soil moisture remained conducive for the soil C-dynamics that resulted in a larger C-flux to the atmosphere via TER that brought the ecosystem to a C-neutral situation even though the primary production occurred at higher rates. The model's underestimation of R H could be attributed to its coarse temporal resolution (annual), whereby it was not able to efficiently capture the non-linearities that occurred at finer temporal resolutions (e.g. daily or sub-daily). For example, while analyzing daily simulations of NEP, Govind et al. (2009b) noted that even during the mid-growing season, on some warm-dry days, the ecosystem behaved as a strong C-source even though GPP occurred at high rates. From these datasets, we understand that the inter-annual variability in hydrometeorological factors made the mature coniferous stand to fluctuate between a weak C-source and a weak C-sink. Thus, it seems that models such as InTEC that run at an annual time-step, although adequate for long-term simulation of C-balance in a spatially distributed manner, are prone to some uncertainties while simulating the C-balance of C-neutral ecosystems. Despite these discrepancies, we should consider the fact that the main purpose of InTEC is to reconstruct the long-term ecosystem C balance and get reasonable estimates of the spatio-temporal dynamics of various C pool sizes and C fluxes, which is difficult with conventional process-based models. 6.2 Temporal dynamics of BCPs and SCPs Fig. 5 shows the temporal dynamics of various ecosystem C pools at the EOBS EC-tower footprint region. The panels A–D, respectively display the simulated temporal patterns of the BCPs such as wood ( C w ), coarse-root ( C cr ), fine-root ( C fr ) and foliage ( C l ). In general, the sizes of the BCPs showed an increase with time, which saturated as the stand got older. In the model, as the stand age increased, relatively higher amount of C is used for growth and maintenance respiration rather than increasing the sizes of BCPs by itself. Therefore, even though photosynthesis occurred at higher rates, there were only smaller increments in the sizes of the BCPs. Among the four BCPs, C fr and C l showed higher interannual variability, which suggested their dynamic nature owing to higher sensitivities to abiotic factors. Biometric studies have shown the rapidity in the annual mortality of fine-root and leaf biomasses as a function of various environmental factors (e.g. Nadelhoffer et al., 1985; Gower and Vitousek, 1989; Kalyn and Van Rees, 2006 ). Among the different land-cover types, deciduous species showed higher inter annual variability for C fr and C l agreeing with the studies conducted by Barr et al. (2004) and King et al. (2001) , respectively. The larger inter annual variability of C fr and C l (panels C and D) were due to the presence of deciduous species within the EC-tower footprint region in addition to coniferous species. In the early years of stand establishment, C w and C cr progressively increased their sizes with smaller interannual variability ( Fig. 5 A and B). This happened because C w and C cr had lower mortality rates (losses) and constant C allocation rates (gains) as opposed to C fr and C l . Unlike the BCPs, the sizes of SCPs had unique temporal patterns. For example, the coarse–woody litter pool ( C cd ) readily reduced during the early stages of stand establishment (between 1920 and 1940). C cd decomposed at rapid rates into the slow soil organic matter pool ( C s ) and to the surface microbial pool ( C m ), with C-fluxes to the atmosphere that contributed to R H . Because of the juvenile nature of the forest stand, the rate of production of C cd was smaller than its decomposition rates, which resulted in a net decrease in its size. However, as the stand age increased, C cd accumulated due to the increased addition of deadwood and coarse root debris, consequent of higher biomass production and mortality, and reached a magnitude as much as 1500 gC m −2 in 2003. Continuous production and mortality of foliage and fine roots even during the initial years resulted in a steady increase in the sizes of the surface structural litter pool ( C ssd ) and the fine root structural litter pool ( C fsd ). These litter-based SCPs were produced by decomposing C l and C fr , respectively. We found that C fsd had pronounced inter-annual variability than C ssd , which further implied the dynamic nature of its precursor BCP, C fr consequent of the annual mortality and regrowth of fine roots ( Fig. 5 F and H). Depending on the lignin fractions and abiotic decomposition factors, C ssd and C fsd , were transformed partly to their metabolic ( C smd and C fmd ) and microbial ( C sm and C m ) counterparts. The simulated sizes of C fsd and C ssd in 2003 were about 450 and 375 gC m −2 , respectively. The sum of the simulated litter-based SCPs accounted as much as 23.25 t C ha −1 in 2003 which was comparable to the measurements taken in a similar black spruce landscape by the studies conducted by Wang et al. (2003) in northern Manitoba, Canada. In the InTEC model, the metabolic SCPs correspond to the C present in active biomolecules (for example enzymes) found in the ecosystem and therefore constituted the most dynamic portion of the C cycle due to their smaller residence times. Temperature and moisture were the main reasons that governed the dynamics of these small-sized metabolic SCPs. The simulated sizes of C smd and C fmd in 2003 were about 5–10 gC m −2 , which were plausible sizes under pristine conditions ( Landgraf et al., 2005; Kielland et al., 2007 ) ( Figs. 5 G and I). The temporal dynamics of those SCPs that are associated with the microbial biomasses on the soil surface ( C sm ) and soil subsurface ( C m ) showed a slight resemblance to their precursor SCPs. While C sm resulted from the microbial decomposition of C ssd and C smd , several precursor SCPs interactively governed the dynamics of C m (refer Table 1 ). Multiple-sources of C m explained its larger built up in comparison to C sm . In this boreal ecosystem, because the near-surface soil temperature was not conducive for microbial proliferation for relatively longer periods on an annual basis, it is quite probable that microbes thrived deeper in the soil. There are studies that show that much of the winter-time soil respiration originates from deeper layers of the soil rather than at the soil surface (e.g. Nobrega and Grogan, 2007 ). The slow ( C s ) and passive ( C p ) SCPs are the most significant and recalcitrant portion of the soil organic matter that are associated with humus and humic substances in the soil. Our simulation suggested that C s only slightly decreased between 1920 and the midsixties, after which it began to build up in the soil. The steady build-up of C s since the sixties was consistent with the increase in the mean annual temperature, precipitation and the atmospheric CO 2 concentration that increased the primary productivity, BCPs and hence, the SCPs. However, higher temperatures also rapidly decomposed the SCPs and increased in size of C s , a recalcitrant type of SCP. From Table 1 , it can be identified that C s is formed as a decomposition product of several other SCPs such as C cd , C ssd , C fsd and C m . The quantities of these SCPs were related to primary productivity, BCP mortalities the consequent litter production. Despite the large size of C p (1453 gC m −2 yr −1 in 2003), it changed only slightly over the temporal span (∼9 gC m −2 yr −1 in 86 years) which implied its recalcitrant nature in comparison to the other SCPs. The small rate of decrease in C p suggested that its precursor SCP, C s , decomposed more into C m , which also showed an increase since the midsixties due to microbial proliferation. Table 1 shows the nature and the dynamics of various BCPs and SCPs and their rates of decomposition. 6.3 Spatial distribution of BCPs and SCPs The simulated spatial distributions of the C pools for the year 2003 are displayed in Fig. 6 . For the ease of presentation, we organize the 13 C pools into 4 broad categories i.e. Aboveground BCPs [ C w + C l ]; Belowground BCPs [ C cr + C fr ]; Surface SCPs [ C cd + C ssd + C smd + C sm ], and subsurface SCPs [ C fsd + C fmd + C m + C s + C p ]. The simulated spatial distributions of these C-stocks reflected the integrated effects of various biotic and abiotic factors on the net C balance. From the spatial distributions of the simulated aboveground and belowground BCPs, we can infer that higher magnitudes of BCPs existed in mixed forest stands than that of coniferous stands and wetlands. In this boreal landscape, aspen and jack pine that rapidly established following a disturbance dominated mixed forest stands. Deciduous and mixed stands had higher annual NPPs (landscape average ∼440 gC m −2 yr −1 ) compared to black spruce-dominated coniferous stands (average ∼220 gC m −2 yr −1 ). Various reasons (physiological, morphological, edaphic, hydrological and topographic) contributed to these spatial trends. Aspen-dominated stands had higher BCPs because of their higher photosynthetic capacity, owing to higher ecophysiological traits such as LAI, stomatal conductance, leaf N concentration and carboxylation rates. The patterns of C turnover in deciduous stands were more intense in comparison to coniferous or wetland species because of large litter inputs from the annual leaf-fall. Note that although mixed stands having deciduous species were relatively younger than coniferous stands, the former had larger sizes of BCPs than the later. Wetlands, in general, showed lower sizes of BCPs in comparison to forested locations because of the lower rates of NPP (landscape average 150–200 gC m −2 yr −1 ). Unlike forests, wetlands showed a temporal consistency in the rates of C stocks accumulation. Age on wetland productivity were comparatively less important. Because BCPs built-up was a direct function of the annual primary productivity, the differences of the sizes of BCPs among the various landcover types increased with time. Hence, the accuracies of various spatial datasets such as land-cover, LAI, soil-type, stand age integratively governed the accuracy of the simulated spatial distributions of the BCPs. Stand age, which implies the productive potential, was an important factor that governed the spatial patterns of the simulated BCPs. Hence, accuracy of the stand age map affected the quality of the simulated C-balance in both space and time. Chen et al. (2003) estimates that InTEC's sensitivity to stand age can be as much as 12–22% of NBP for ±5 years bias in the stand age. Considering this, we believe that efforts to map stand age and forest disturbance regimes using geomatics and modeling should be given higher priority in various research consortia that deal with the large-scale simulation of the terrestrial C-cycle. Currently, few spatially explicit datasets on forest disturbance and stand-age data are available for C modelers. Most of these datasets confine to the high-latitude ecosystems (e.g. Canadian landmass, Chen et al., 2003 ) and the (e.g. United States, Goward et al., 2008 ). The spatial distributions of the simulated SCPs (especially the surface-SCPs) resembled that of the BCPs because they were primarily derived from the mortality of BCPs. Wetlands showed larger accumulation of surface SCPs because of higher soil moisture conditions that limited their decomposition. The simulated spatial distributions revealed that the sizes of surface SCPs in wetlands were similar to that of deciduous forests (∼3000 gC m −2 ) even though primary production in the former was relatively much lower (∼150 gC m −2 yr −1 ) than the latter (∼700 gC m −2 yr −1 ). Studies have shown that reduced aeration due to excess soil water reduces microbial activities limit the decomposition of SCPs ( Davidson and Lefebvre, 1993; Schuur and Trumbore, 2006; Sun et al., 2004 ) leading to an accumulation of surface SCPs (e.g. moss necromass and fibric and hemic peat). The accumulation of surface SCPs in wetlands resulted in smaller quantities of sub-surface SCPs unlike other land-cover types where the subsurface SCPs were higher because of rapid bigeochemical transformations. Even though we simulated the spatial trends of BCPs and SCPs in wetlands, we believe that there is more room for improvement. Addressing wetland-specific biogeochemical processes such as DOC and methane fluxes that uniquely govern wetland biogeochemistry needs further research. We acknowledge the fact that the use of lower values of decomposition coefficients for various SCPs is not the ideal solution to comprehensively capture wetland C-dynamics. Future works in this direction should incorporate wetland-specific biogeochemical processes within the InTEC framework. This approach warrants the need for spatially-distributed hydro-thermal considerations that incorporate topography and landscape scale hydrological processes that govern the local-scale biogeochemical processes (after Govind et al., 2010 ). 6.4 Validation of simulated BCPs Fig. 7 a shows the comparison of the simulated above ground BCPs [ C w and C l ] that constitute the C contained in the AGB with the biometric measurements taken in 2003 at the 12 inventory plots. In general, the simulated BCPs agreed reasonably with the biometric estimates ( R = 0.86, RMSE = 0.96 kgC m −2 , p < 0.0001). The spatial scale at which these comparisons were made, were not far apart. The 400 m 2 inventory plots were comparable to an InTEC modeling unit (pixel size = 625 m 2 ). There was a general tendency for the model to underestimate the aboveground BCPs. This was especially true in the plots 16 T, 17 T and 51 T ( Fig. 2 ). There are three possible reasons that could explain these discrepancies. Firstly, the allometric equations used to estimate biomass from the DBH measurements might have species-specific biases ( Lambert et al., 2005 ). Secondly, AGB C-stocks were biometrically computed with the assumption that the C fraction in biomass (CFB) is 50%, an assumption that may not be fully valid under field conditions. The C-fraction of BCPs could vary depending on plant physiological status and plant parts. Some studies show that CFB in wood ranges between 47% and 50% ( Thomas and Malczewski, 2007 ) while others show that CFB of wood and leaves are 50% and 45%, respectively ( Ajtay et al., 1977 ). Therefore, an assumption of CFB = 50% can create discrepancies in the simulation of C-stocks at those locations where the foliage contributions were high. Thirdly, the inherent conceptualizations of InTEC may also have shortcomings with regard to addressing all the ecological complexities. Fig. 7 c shows a linear relationship between the simulated AGB and the PAI measured. PAI is a strong indicator of the aboveground C stocks. The relationship indicates that the spatial variability of AGB in the field was reasonably captured by the model. We opted to use PAI instead of the LAI because the former is indicative of the amount of both foliage and wood per unit ground area rather than LAI, which is indicative only of the foliar abundance. The moderate correlation ( r = 0.87) could be attributed partly due to variability in woody and foliage fractions that affected the PAI estimates at each point. Nevertheless, the probability that this trend occurred by chance was <0.001 ( H 0 : β 1 = 0; H 1 : β 1 ≠ 0). 6.5 Validation of simulated SCPs Fig. 7 b shows a comparison of the simulated soil C-stocks in 2003 (as the sum of all the SCPs, C cd , C ssd , C smd , C sm , C fmd , C fsd , C m , C s , and C p ) with the measured soil C-stocks (2003). The simulated soil C-stock agreed reasonably well with the measurements ( r = 0.84, p = 0.0004). The EOBS footprint average soil C-stock was simulated to be 10.01 kgC m −2 as against 10.40 kgC m −2 measured for the year 2003. There was an underestimation in the plots 17 T and 24 T and an overestimation in the plots 36 T and 39 T. These discrepancies might have arose due to (1) errors encountered while upscaling point-scale measurements to the plot-scale of 400 m 2 area and (2) InTEC's inherent limitations in describing some of the ecological processes. During our 2004 and 2005 field campaigns, we noted that these locations (17 T and 24 T) had a predominant moss-layer with thick necro-mass (dead moss layer). We believe that moss necro-mass on the forest floor might have contributed to the large built-up of C in the soil organic layer. There are studies that demonstrate the importance of bryophytes in the boreal C cycle ( Frolking et al., 1996; Bisbee et al., 2001; Bond-Lamberty et al., 2004 ) which strengthens our hypothesis. Although our NPP ref map was simulated using the BEPS-TerrainLab V2.0 model that has adequate representations of moss-layer contribution to C fluxes. However, this alone is insufficient. In the InTEC model, the temporal dynamics of moss productivity is not explicitly considered. The historical trends in moss growth and its contribution towards SCP could be quite different from that of vascular plants. With the advances in the remote sensing science, it may be possible to map the moss and lichen abundance on the forest floor (e.g. Peckham et al., 2009 ). Mapping of forest understory might aid in better understanding the C cycling in high latitude humid ecosystems. Another explanation for the discrepancies in SCPs could also be because InTEC ignores C fluxes related to DOC and methane. Studies have shown that sizable quantities of DOC could be exchanged between soil complexes along with topographically driven lateral water fluxes ( Hornberger et al., 1994; Aitkenhead-Peterson et al., 2007 ). Govind et al. (2009a) has demonstrated that considerable amount of lateral water fluxes occur in this perhumid ecosystem mainly in the form of sub surface flow via mineral and organic layers of the soil. 7 Summary and conclusions Modeling of terrestrial C cycle needs detailed information on BCPs and SCPs because their sizes govern the magnitudes and directions of C fluxes. Incorrectly initialized ecosystem C pools could create systematic errors in the simulated biogeochemical processes. Although measured values are the best estimates, it is not practical to measure sizes of C pools at spatially and temporally at fine resolutions. One possible alternative to reduce this knowledge-gap is to use a long-term and spatially distributed modeling approach that considers the multiple factors that govern the long-term ecological and biogeochemical processes. We simulated the C balance of a boreal region by using a spatially and temporally explicit C balance model that uses various types of datasets. The spatio-temporal dynamics of various conceptual C pools and their fluxes were simulated under changes in climate, atmospheric chemistry and disturbances. The study showed that the productivity of the undisturbed stands in this boreal landscape was low in the recent years and the ecosystem was almost near C neutrality. In the recent years, the EOBS site has been shifting its status as a weak C sink or a weak C source depending on the interannual variation of the hydrometeorological conditions. Locations dominated by middle-aged stands behaved as larger C sinks. The comparison of the simulated and the measured C stocks in plant and soil revealed that InTEC simulations were reasonably accurate although some discrepancies were observed. The importance of this work lies in the simulation of the long-term C balance (dynamics of C pools and fluxes) in a spatially-explicit manner integrating the effects of climate, atmospheric chemistry and disturbances. Considering the current levels of disagreements between the “bottom-up” and “top-down” estimates of the terrestrial C balance at large scales, improved bottom-up estimates of C sources and sinks of this sort might help in reducing the uncertainties. Acknowledgements This work was supported by the Canadian Carbon Program, funded by the Canadian Foundation for Climate and Atmospheric Sciences, Natural Sciences and Engineering Research Council of Canada, and the BIOCAP Canada. We thank Prof. Weimin Ju, Nanjing University, China, for useful scientific discussions during the modeling phase. The authors are grateful to Onil Bergeron, Carole Coursolle, and Marc-André Giasson for their contributions in obtaining the flux data at EOBS.The authors thank Sebastien Dagnault, Marie-Claude Lambert, and Jean-Pierre Berube at the Laurentian Forestry Center of the Canadian Forest Service. AG is grateful to Jyothi Kumari, INRA-EPHYSE, for discussions on soil C dynamics. The authors would like to thank the anonymous reviewers whose comments improved the clarity of the paper. Appendix A Acronyms Used in this Paper C carbon N nitrogen GPP gross primary productivity NPP net primary productivity NPP 0 NPP at the equilibrium condition NPP i NPP at the year i NPP ref reference year NPP NEP net ecosystem productivity NBP net biome productivity ET evapotranspiration AGB aboveground biomass CFB carbon fraction in biomass TER total ecosystem respiration R A autotrophic respiration R H heterotrophic respiration LAI leaf area index PAI plant area index EOBS eastern old black spruce site of CCP DBH diameter at breast height BCP biomass C pools SCP soil C pools GIS geographic information system Appendix B Calculating the dynamics of BCPs and SCPs Subscripts denoting various C pools. C x C content in pool x in gC m −2 a C released to the atmosphere c r coarse-root biomass pool f r fine-root biomass pool l foliage biomass pool w wood biomass pool c d coarse-woody structural detritus pool f sd fine-root structural detritus pool s sd surface structural detritus pool s md metabolites in surface litter pool f md metabolites in soil litter pool s m surface microbial pool m soil microbial pool p passive soil organic matter pool s slow soil organic matter pool k x , y transfer coefficient of C from pool x to y in a year F m Partitioning coefficient for foliage and fine-root litters into their corresponding metabolic C pools f y fraction of NPP allocated to a particular biomass pool y ξ x C loss from pool x from the ecosystem due to disturbance ξ l = 1 fraction of foliage lost ξ w = 0.25 fraction of wood/stem lost ξ r = 0 fraction of root lost ξ slb = 1 fraction of surface litter lost ξ cd = 0.2 fraction of coarse woody litter lost Equations of carbon pool decomposition at annual time steps. Under conditions of no disturbance, the BCPs change in the following manner. (A1) Δ C w , i = f w . N P P i − k w , cd . C w i − 1 1 + k w , cd (A2) Δ C cr , i = f cr . NPP i − k cr , cd . C c r i − 1 1 + k cr , cd (A3) Δ C l , i = f l ⋅ NPP i − k l , ssd . C l i − 1 1 + k l , ssd (A4) Δ C fr , i = f fr ⋅ NPP i − k fr , fmd . C f r i − 1 1 + k fr , fmd In the model, currently, all forms of disturbances are manifested as pyrogenic C emissions. When a disturbance when a disturbance event (fire) occurs, it is assumed that 100% foliage C and 25% of woody C are rapidly lost to the atmosphere. The remaining BCPs are transferred to the corresponding SCPs via the pathways built in the model. The following equations show how the temporal changes in SCPs are calculated under undisturbed and disturbed conditions. Change in the coarse-woody litter C pool (undisturbed case) (A5) Δ C c d i = k w , cd . C w i + k cr , cd . C c r i − ( k cd , a + k cd , m + k cd , s ) C c d i − 1 1 + ( k cd , a + k cd , m + k cd , s ) Change in the coarse-woody litter C pool (disturbed case) (A6) Δ C c d i = ( ( 1 − ξ w ) . C w i − 1 ) + ( ( 1 − ξ r ) . C c r i − 1 ) − ( ξ cd . C c d i − 1 ) − ( 1 − ξ cd ) . ( k cd , a + k cd , m + k cd , s ) C c d i − 1 1 + ( k cd , a + k cd , m + k cd , s ) Change in the surface structural litter C pool (undisturbed case) (A7) Δ C ssd i = ( 1 − F m ) ⋅ k l , sl . C l i − ( k ssd , sm + k ssd , s + k ssd , a ) C ssd i − 1 1 + ( k ssd , sm + k ssd , s + k ssd , a ) Change in the surface structural litter C pool (disturbed case) (A8) Δ C ssd i = ( 1 − F m ) ⋅ ( 1 − ξ l ) . C l i − 1 − ( ( 1 − ξ slb ) . C ssd i − 1 ) − ( 1 − ξ s l b ) ⋅ ( k ssd , sm + k ssd , s + k ssd , a ) C ssd i − 1 1 + ( k ssd , sm + k ssd , s + k ssd , a ) Change in the surface metabolic C pool (undisturbed case) (A9) Δ C smd i = ( F m i ) k l , sl . C l i − ( k fd , a + k fd , m + k fd , s ) C smd i − 1 1 + ( k smd , a + k smd , sm ) Change in the surface metabolic C pool (disturbed case) (A10) Δ C smd i = ( F m i ) ( 1 − ξ l ) ⋅ C l i − ( ξ slb ⋅ C smd i − 1 ) − ( 1 − ξ slb ) ⋅ ( k fd , a + k fd , m + k fd , s ) C smd i − 1 1 + ( k smd , a + k smd , sm ) Change in the soil structural litter C pool (undisturbed case) (A11) Δ C fsd i = ( 1 − F m ) ⋅ k fr , fl ⋅ C f r i − ( k fsd , m + k fsd , s + k fsd , a ) C fsd i − 1 1 + ( k fsd , m + k fsd , s + k fsd , a ) Change in the soil structural litter C pool (disturbed case) (A12) Δ C fsd i = ( 1 − F m ) ⋅ ( 1 − ξ r ) ⋅ C f r i − 1 − ( k f s d , s m + k f s d , s + k fsd , a ) C fsd i − 1 1 + ( k fsd , sm + k fsd , s + k fsd , a ) Change in the soil metabolic C pool (undisturbed case) (A13) Δ C fmd i = ( F m i ) k fr , fl ⋅ C f r i − ( k fmd , a + k fmd , m ) C fmd i − 1 1 + ( k fmd , a + k fmd , m ) Change in the soil metabolic C pool (disturbed case) (A14) Δ C smd i = ( F m i ) ( 1 − ξ r ) ⋅ C f r i − ( k fmd , a + k fmd , m ) C fmd i − 1 1 + ( k fmd , a + k fmd , m ) Disturbance does not directly affect the remaining C pools. Change in the surface microbial C pool (A15) Δ C s m i = ( k s s d , s m . C ssd i + k smd , sm ⋅ C smd i i ) − . C s m i − 1 ( k sm , a + k sm , s ) 1 + ( k sm , a + k sm , s ) Change in the soil microbial C pool (A16) Δ C m i = ( k fsd , m ⋅ C fsd i + k fmd , m ⋅ C fmd i + k cd , m ⋅ C c d i + k s , m ⋅ C s i − 1 + k p , m . C p i − 1 ) ( k m , a + k m , s + k m , p ) ⋅ C m i − 1 1 + ( k m , a + k m , s + k m , p ) Change in the Slow C pool (A17) Δ C s i = ( k cd , s . C c d i + k fd , s ⋅ C f d i + k m , s C m i ) − ( k s , a + k s , p ⋅ C s i − 1 ) 1 + ( k s , a + k s , p + k s , m ) Change in the Passive C pool (A19) Δ C p i = ( k m , p . C m i + k s , p . C s i ) − C p i − 1 ( k p , m + k p , a ) 1 + ( k p , m + k p , a ) References Aitkenhead-Peterson et al., 2007 J.A. Aitkenhead-Peterson R.P. Smart M.J. Aitkenhead M.S. Cresser W.H. Mcdowell Spatial and temporal variation of dissolved organic carbon export from gauged and ungauged watersheds of Dee Valley, Scotland: effect of land cover and C:N Water Resources Research 2007 43 Ajtay et al., 1977 G.L. Ajtay P. Ketner P. Duvigneaud Terrestrial primary production and phytomass The Global Carbon Cycle 1977 John Wiley New York pp. 129–181 Alexandrov et al., 2003 G.A. Alexandrov T. Oikawa Y. Yamagata Climate dependence of the CO 2 fertilization effect on terrestrial net primary production Tellus Series B: Chemical and Physical Meteorology 55 2003 669 675 Amiro et al., 2010 Amiro, B.D., Barr, A.G., Black, T.A., Brown, M., Chen, J.M., Clark, K.L., Davis, K., Desai, A., S. Dore, S., Fuentes, J., Goulden, M., Kolb, T., Lavigne, M., Law, B., Margolis, H.A., Martin, T., McCaughey, J.H., Montes-Helu, M., Noormets, A., Randerson, J., Xiao, J., (2010). Eddy covariance measurements of carbon flux in North American forest disturbance chronosequences. J. Geophys. Res., 115, G00K02, doi:10.1029/2010JG001390 . Apps et al., 2000 M.J. Apps J.S. Bahatti D. Halliwell H. Jiang Influence of uniform versus random disturbance regime on carbon dynamics in the boreal forest of central Canada R. Lal Kimble J. Eswarn H. Stewart B.A. Global Change and Cold Ecosystems 2000 CRC Press Boca Raton 413 426 Arora and Boer, 2003 V.K. Arora G.J. Boer A representation of variable root distribution in dynamic vegetation models Earth Interactions 7 2003 Paper 6, 19 pp Austin et al., 2003 J.M. Austin B.G. Mackey K.P. Van Niel Estimating forest biomass using satellite radar: an exploratory study in a temperate Australian Eucalyptus forest Forest Ecology and Management 176 2003 575 583 Baldocchi, 2008 D Baldocchi Breathing of the terrestrial biosphere: lessons learned from a global network of carbon dioxide flux measurement systems Australian Journal of Botany 56 2008 1 26 Barr et al., 2004 A.G. Barr T.A. Black E.H. Hogg N. Kljun K. Morgenstern Z. Nesic Inter-annual variability in the leaf area index of a boreal Aspen-Hazelnut forest in relation to net ecosystem production Agricultural and Forest Meteorology 126 2004 237 255 Bergeron et al., 2007 O. Bergeron H.A. Margolis T.A. Black C. Coursolle A.L. Dunn A.G. Barr S.C. Wofsy Comparison of carbon dioxide fluxes over three boreal black spruce forests in Canada Global Change Biology 13 2007 89 107 Bergeron et al., 2008 O. Bergeron H.A. Margolis How does forest harvest influence carbon dioxide fluxes of black spruce ecosystems in eastern North America? Agricultural and Forest Meteorology 148 4 2008 537 548 Bernier et al., 2010 P.Y. Bernier L. Guindon W.A. Kurz G. Stinson Reconstructing and modelling 71 years of forest growth in a Canadian boreal landscape: a test of the CBM-CFS3 carbon accounting model Canadian Journal of Forest Research 40 2010 109 118 Bisbee et al., 2001 K.E. Bisbee S.T. Gower J.M. Norman E.V. Nordheim Environmental controls on ground cover species composition and productivity in a Boreal Black Spruce forest Oecologia 129 2001 261 270 Black et al., 2000 T.A. Black W.J. Chen A.G. Barr M.A. Arain Z. Chen Z. Nesic E.H. Hogg H.H. Neumann P.C. Yang Increased carbon sequestration by a boreal deciduous forest in years with a warm spring Geophysical Research Letters 27 2000 1271 1274 Bond-Lamberty and Thomson, 2010 B. Bond-Lamberty A.M. Thomson Temperature-associated increases in the global soil respiration record Nature 464 2010 579 582 Bond-Lamberty et al., 2004 B. Bond-Lamberty C.K. Wang S.T. Gower Net primary production and net ecosystem production of a boreal black spruce wildfire chronosequence Global Change Biology 10 2004 473 487 Chen et al., 2000 W.J. Chen J. Chen J. Cihlar An integrated terrestrial ecosystem carbon-budget model based on changes in disturbance, climate, and atmospheric chemistry Ecological Modelling 135 2000 55 79 Chen et al., 2002 W Chen J.M. Chen D.T. Price J Cihlar Effects of stand age on net primary productivity of boreal black spruce forests in Canada Canadian Journal of Forest Research 32 2002 833 842 Chen et al., 2003 J.M. Chen W.M. Ju J. Cihlar D. Price J. Liu W.J. Chen J.J. Pan A. Black A. Barr Spatial distribution of carbon sources and sinks in Canada's forests Tellus Series B: Chemical and Physical Meteorology 55 2003 622 641 Chen et al., 2006 J.M. Chen A. Govind O. Sonnentag Y.Q. Zhang A. Barr B. Amiro Leaf area index measurements at Fluxnet-Canada forest sites Agricultural and Forest Meteorology 140 2006 257 268 Coops et al., 2007 N.C. Coops T.A. Black R.P.S. Jassal J.A.T. Trofymow K. Morgenstern Comparison of Modis, Eddy covariance determined and physiologically modelled gross primary production (GPP) in a Douglas-Fir forest stand Remote Sensing of Environment 107 2007 385 401 Coursolle et al., 2006 C. Coursolle H.A. Margolis A.G. Barr T.A. Black B.D. Amiro J.H. McCaughey L.B. Flanagan P.M. Lafleur N.T. Roulet C.P-A. Bourque M.A. Arain S.C. Wofsy A. Dunn K. Morgenstern A.L. Orchansky P.-Y. Bernier J.M. Chen J. Kidston N. Saigusa N. Hedstrom Late-summer carbon fluxes from Canadian forests and peatlands along an east-west continental transect Canadian Journal of Forest Research 36 2006 783 800 Cox et al., 2000 P.M. Cox R.A. Betts C.D. Jones S.A. Spall I.J. Totterdell Acceleration of global warming due to carbon-cycle feedbacks in a coupled climate model Nature 408 2000 750 (vol. 408, pp. 184, 2000) Davidson and Lefebvre, 1993 E.A. Davidson P.A. Lefebvre Estimating regional carbon stocks and spatially covarying edaphic factors using soil maps at 3 scales Biogeochemistry 22 1993 107 131 Environment Canada, 2006 Environment Canada Canadian Climate Normals or Averages 1971–2000 2006 2007-05-15 Franks et al., 1997 S.W. Franks K.J. Beven P.F. Quinn I.R. Wright On the sensitivity of soil-vegetation-atmosphere transfer SVAT schemes: equifinality and the problem of robust calibration Agricultural and Forest Meteorology 86 1997 63 75 Frolking et al., 1996 S. Frolking M.L. Goulden S.C. Wofsy S.M. Fan D.J. Sutton J.W. Munger A.M. Bazzaz B.C. Daube P.M. Crill J.D. Aber L.E. Band X. Wang K. Savage T. Moore R.C. Harriss Modelling temporal variability in the carbon balance of a spruce/moss boreal forest Global Change Biology 2 1996 343 366 Giasson et al., 2006 M.A. Giasson C. Coursolle H.A. Margolis Ecosystem-level CO 2 fluxes from a boreal cutover in Eastern canada before and after scarification Agricultural and Forest Meteorology 140 2006 23 40 Govind et al., 2009a A. Govind J.M. Chen H. Margolis W. Ju O. Sonnentag M.A. Giasson A spatially explicit hydro-ecological modeling framework (BEPS-TerrainLab V2. 0): model description and test in a Boreal ecosystem in Eastern North America Journal of Hydrology 367 2009 200 216 Govind et al., 2009b Govind, A., Chen, J.M., Ju, W., 2009b. Spatially explicit simulation of hydrologically controlled carbon and nitrogen cycles and associated feedback mechanisms in a Boreal ecosystem. Journal of Geophysical Research 114, G02006, doi:10.1029/2008JG000728. Govind et al., 2010 Govind, A., Chen, J.M., McDonnell, J.J., Kumari, J., Sonnentag, O. (2010). Effects of lateral hydrological processes on photosynthesis and evapotranspiration in a boreal ecosystem. Ecohydrology, doi:10.1002/eco.141. Goward et al., 2008 S.N. Goward J.G. Masek W. Cohen G. Moisen G.J. Collatz S. Healey R.A. Houghton C. Huang R. Kennedy B. Law S. Powell D. Turner M.A. Wulder Forest disturbance and North American carbon flux EOS, Transactions, American Geophysical Union 89 11 2008 105 116 Gower and Vitousek, 1989 S.T. Gower P.M. Vitousek Effects of nutrient amendments on fine root biomass in a primary successional forest in Hawaii Oecologia 81 1989 566 568 Gower et al., 1996 S.T. Gower R.E. Mcmurtrie D. Murty Aboveground net primary production decline with stand age: potential causes Trends in Ecology and Evolution 11 1996 378 382 Gower et al., 1997 S.T. Gower J.G. Vogel J.M. Norman C.J. Kucharik S.J. Steele T.K. Stow Carbon distribution and aboveground net primary production in aspen, jack pine, and black spruce stands in Saskatchewan and Manitoba Canada, Journal of Geophysical Research: Atmospheres 102 1997 29029 29041 Grant et al., 2009 R.F. Grant A.G. Barr T.A. Black H.A. Margolis A.L. Dunn J. Metsaant S. Wang J.H. McCaughey C.-A. Bourque Interannual variation in net ecosystem productivity of Canadian forests as affected by regional weather patterns – a Fluxnet-Canada synthesis Agricultural and Forest Meteorology 149 2009 2022 2039 Griffis et al., 2003 T.J. Griffis T.A. Black K. Morgenstern A.G. Barr Z. Nesic G.B. Drewitt D. Gaumont-Guay J.H. Mccaughey Ecophysiological controls on the carbon balances of three southern boreal forests Agricultural and Forest Meteorology 117 2003 53 71 Hassan et al., 2006 Q.K. Hassan C.P-A. Bourque F.-R. Meng Estimation of daytime net ecosystem CO 2 exchange over balsam fir forests in eastern Canada: combining averaged tower-based flux measurements with remotely sensed MODIS data Canadian Journal of Remote Sensing 32 2006 405 416 Hornberger et al., 1994 G.M. Hornberger K.E. Bencala D.M. Mcknight Hydrological controls on dissolved organic-carbon during snowmelt in the snake river near Montezuma, Colorado Biogeochemistry 25 1994 147 165 Janssens et al., 2003 I.A. Janssens A. Freibauer P. Ciais P. Smith G.J. Nabuurs G. Folberth B. schlamadinger R.W.A. Hutjes R. Ceulemans E.-D. Schulze R. Valentini A.J. Dolman Europe's terrestrial biosphere absorbs 7–12% of European anthropogenic CO 2 emissions Science 300 2003 1538 1542 Johnson et al., 2000 D.W. Johnson W. Cheng J.T. Ball Effects of CO 2 and N fertilization on decomposition and N immobilization in ponderosa pine litter Plant and Soil 224 2000 115 122 Ju and Chen, 2005 W.M. Ju J.M. Chen Distribution of soil carbon stocks in Canada's forests and wetlands simulated based on drainage class, topography and remotely sensed vegetation parameters Hydrological Processes 19 2005 77 94 Ju and Chen, 2008 Ju, W., Chen, J.M., 2008. Effects of past changes in climate, atmospheric composition, and disturbance on soil carbon in Canada's forests and wetlands. Global Biogeochemical Cycles, 22, GB3010, doi:10.1029/2007GB002935. Ju et al., 2006 W.M. Ju J.M. Chen T.A. Black A.G. Barr H. Mccaughey N.T. Roulet Hydrological effects on carbon cycles of Canada's forests and wetlands Tellus Series B: Chemical and Physical Meteorology 58 2006 16 30 Ju et al., 2007 W. Ju J.M. Chen D. Harvey S. Wang Future carbon balance of China's forests under climate change and increasing CO 2 Journal of Environmental Management 85 2007 538 562 Kalyn and Van Rees, 2006 A.L. Kalyn K.C.J. Van Rees Contribution of fine roots to ecosystem biomass and net primary production in black spruce, aspen, and jack pine forests in Saskatchewan Agricultural and Forest Meteorology 140 2006 236 243 Kelly et al., 2002 Kelly, R.D., Hunt, E.R.Jr., Reiners, W.A., Smith, W.K., Welker, J.M., 2002. Relationships between daytime carbon dioxide uptake and absorbed photosynthetically active radiation for three different mountain/plains ecosystems, J. Geophys. Res., 107(D14), 4223, doi:10.1029/2001JD001181. Kielland et al., 2007 K. Kielland J.W. Mcfarland R.W. Ruess K. Olson Rapid cycling of organic nitrogen in Taiga forest ecosystems Ecosystems 10 2007 360 368 King et al., 2001 King J.S. Pregitzer K.S. Zak D.R. Sober J. Isebrands J.G. Dickson R.E. Hendrey G.R. Karnosky D.F. Fine-root biomass and fluxes of soil carbon in young stands of paper birch and trembling aspen as affected by elevated atmospheric CO 2 and tropospheric O 3 Oecologia 128 2001 237 250 Kljun et al., 2004 N Kljun P. Calanca M.W. Rotach H.P. Schmid A simple parameterisation for flux footprint predictions Boundary-Layer Meteorology 112 2004 503 523 Lambert et al., 2005 M.C. Lambert C.H. Ung F. Raulier Canadian national tree aboveground biomass equations Canadian Journal of Forest Research-Revue Canadienne De Recherche Forestiere 35 2005 1996 2018 Landgraf et al., 2005 D. Landgraf S. Wedig S. Klose Medium- and short-term available organic matter, microbial biomass, and enzyme Activities in soils under Pinus Sylvestris L. and robinia pseudoacacia L. In a sandy soil in Ne Saxony, Germany Journal of Plant Nutrition and Soil Science-Zeitschrift Fur Pflanzenernahrung Und Bodenkunde 168 2005 193 201 Landsberg and Waring, 1997 J.J. Landsberg R.H. Waring A generalised model of forest productivity using simplified concepts of radiation-use efficiency, carbon balance and partitioning Forest Ecology and Management 95 1997 209 228 le Maire et al., 2010 le Maire, G., et al. (2010), Detecting the critical periods that underpin interannual fluctuations in the carbon balance of European forests, J. Geophys. Res., 115, G00H03, doi:10.1029/2009JG001244. Lovett et al., 2006 G.M. Lovett J.J. Cole M.L. Pace Is net ecosystem production equal to ecosystem carbon accumulation? Ecosystems 9 2006 152 155 Luo et al., 2009 Y.Q. Luo E.S. Weng X.W. Wu C. Gao X.H. Zhou L. Zhang Parameter identifiability, constraint, and equifinality in data assimilation with ecosystem models Ecological Applications 19 2009 571 574 Magnani et al., 2007 F. Magnani M. Mencuccini M. Borghetti P. Berbigier F. Berninger S. Delzon A. Grelle P. Hari P.G. Jarvis P. Kolari A.S. Kowalski H. Lankreijer B.E. Law A. Lindroth D. Loustau J. Manca J. Moncrieff M. Rayment V. Tedeschi R. Valentini J. Grace The human footprint in the carbon cycle of established temperate and boreal forests Nature 447 2007 848 850 Mitchell et al., 2009 S. Mitchell K. Beven J. Freer Multiple sources of predictive uncertainty in modeled estimates of net ecosystem CO 2 exchange Ecol. Modelling. 220 2009 3259 3270 Nadelhoffer et al., 1985 K.J. Nadelhoffer J.D. Aber J.M. Melillo Fine roots, net primary production, and soil-nitrogen availability – a new hypothesis Ecology 66 1985 1377 1390 Nobrega and Grogan, 2007 S. Nobrega P. Grogan Deeper snow enhances winter respiration from both plant-associated and bulk soil carbon pools in birch hummock tundra Ecosystems 10 3 2007 419 431 Peckham et al., 2009 S.D. Peckham D.E. Ahl S.T. Gower Bryophyte cover estimation in a boreal black spruce forest using airborne lidar and multispectral sensors Remote Sensing of Environment 113 2009 1127 1132 Peltoniemi et al., 2004 M. Peltoniemi R. Makipaa J. Liski P. Tamminen Changes in soil carbon with stand age – an evaluation of a modelling method with empirical data Global Change Biology 10 2004 2078 2091 Popescu, 2007 S.C. Popescu Estimating biomass of individual pine trees using airborne LiDAR Biomass and Bioenergy 31 2007 646 655 Porporato et al., 2003 A. Porporato P. D’odorico F. Laio I. Rodriguez-Iturbe Hydrologic controls on soil carbon and nitrogen cycles. I. modeling scheme Advances in Water Resources 26 2003 45 58 Potter et al., 2001 C. Potter S. Klooster C.R. De Carvalho V.B. Genovese A. Torregrosa J. Dungan M. Bobo J. Coughlan Modeling seasonal and interannual variability in ecosystem carbon cycling for the Brazilian Amazon Region Journal of Geophysical Research Atmospheres 106 2001 10423 10446 Rapalee et al., 1998 G. Rapalee S.E. Trumbore E.A. Davidson J.W. Harden H. Veldhuis Soil carbon stocks and their rates of accumulation and loss in a boreal forest landscape Global Biogeochemical Cycles 12 1998 687 701 Raupach et al., 2005 M.R. Raupach P.J. Rayner D.J. Barrett Model-data synthesis in terrestrial carbon observation: methods, data requirements and data uncertainty specifications Global Change Biology 11 2005 378 397 Regniere and St-Amant, 2007 J. Regniere R. St-Amant Stochastic simulation of daily air temperature and precipitation from monthly normals in North America North of Mexico International Journal of Biometeorology 51 2007 415 430 Ro et al., 1995 C. Ro R. Vet D. Ord A. Holloway Canadian air and precipitation monitoring network (CAPMoN) annual summary reports (1983-1994). National atmospheric chemistry database (NAtChem), Atmospheric Environment Service Environment Canada 1995 Ryan et al., 1997 M.G. Ryan M.B. Lavigne S.T. Gower Annual carbon cost of autotrophic respiration in boreal forest ecosystems in relation to species and climate Journal of Geophysical Research – Atmospheres 102 1997 28871 28883 Schulz et al., 2001 K. Schulz A. Jarvis K. Beven H. Soegaard The predictive uncertainty of land. surface fluxes in response to increasing ambient carbon dioxide Journal of Climate 14 2001 2551 2562 Schuur and Trumbore, 2006 E.A.G. Schuur S.E. Trumbore Partitioning sources of soil respiration in boreal black spruce forest using radiocarbon Global Change Biology 12 2006 165 176 Shao et al., 2007 Y. Shao J. Pan L. Yang J.M. Chen W. Ju X Shi Validation of soil organic carbon density using the InTEC model Journal of Environmental Management 85 2007 696 701 Shibu et al., 2006 M.E. Shibu P.A. Leffelaar H. Van Keulen P.K. Aggarwal Quantitative description of soil organic matter dynamics – a review of approaches with reference to rice-based cropping systems Geoderma 137 2006 1 18 Sun et al., 2004 O.J. Sun J. Campbell B.E. Law V. Wolf Dynamics of carbon stocks in soils and detritus across chronosequences of different forest types in the Pacific Northwest, USA Global Change Biology 10 2004 1470 1481 Tague and Band, 2004 C. Tague L. Band RHESSys: regional hydro-ecologic simulation system: an object-oriented approach to spatially distributed modeling of carbon, water and nutrient cycling Earth Interactions 8 19 2004 1 42 Thomas and Malczewski, 2007 S.C. Thomas G. Malczewski Wood carbon content of tree species in Eastern China: interspecific variability and the importance of the volatile fraction: carbon sequestration in China's forest ecosystems Journal of Environmental Management 85 3 2007 659 662 Torn et al., 1997 M.S. Torn S.E. Trumbore O.A. Chadwick P.M. Vitousek D.M. Hendricks Mineral control of soil organic carbon storage and turnover Nature 389 1997 170 173 Turner et al., 2006 D.P. Turner W.D. Ritts J.M. Styles Z. Yang W.B. Cohen B.E. Law P.E. Thornton A diagnostic carbon flux model to monitor the effects of disturbance and interannual variation in climate on regional Nep Tellus Series B: Chemical and Physical Meteorology 58 2006 476 490 Turunen et al., 2004 J. Turunen N.T. Roulet T.R. Moore P.J.H. Richard Nitrogen deposition and increased carbon accumulation in ombrotrophic peatlands in Eastern Canada Global Biogeochemical Cycles 2004 18 Wang et al., 2003 C.K. Wang B. Bond-Lamberty S.T. Gower Carbon distribution of a well- and poorly-drained black spruce fire chronosequence Global Change Biology 9 2003 1066 1079 Wang et al., 2007 P. Wang R. Sun J. Hu Q. Zhu Y. Zhou L. Li J.M. Chen Measurements and simulation of forest leaf area index and net primary productivity in Northern China Journal of Environmental Management 85 2007 607 615
更多查看译文
关键词
Long-term C modeling,InTEC,Boreal ecosystem,Climate change,Changes in atmospheric chemistry,Disturbance,Biomass C pools [BCPs],Soil C pools [SCPs]
AI 理解论文
溯源树
样例
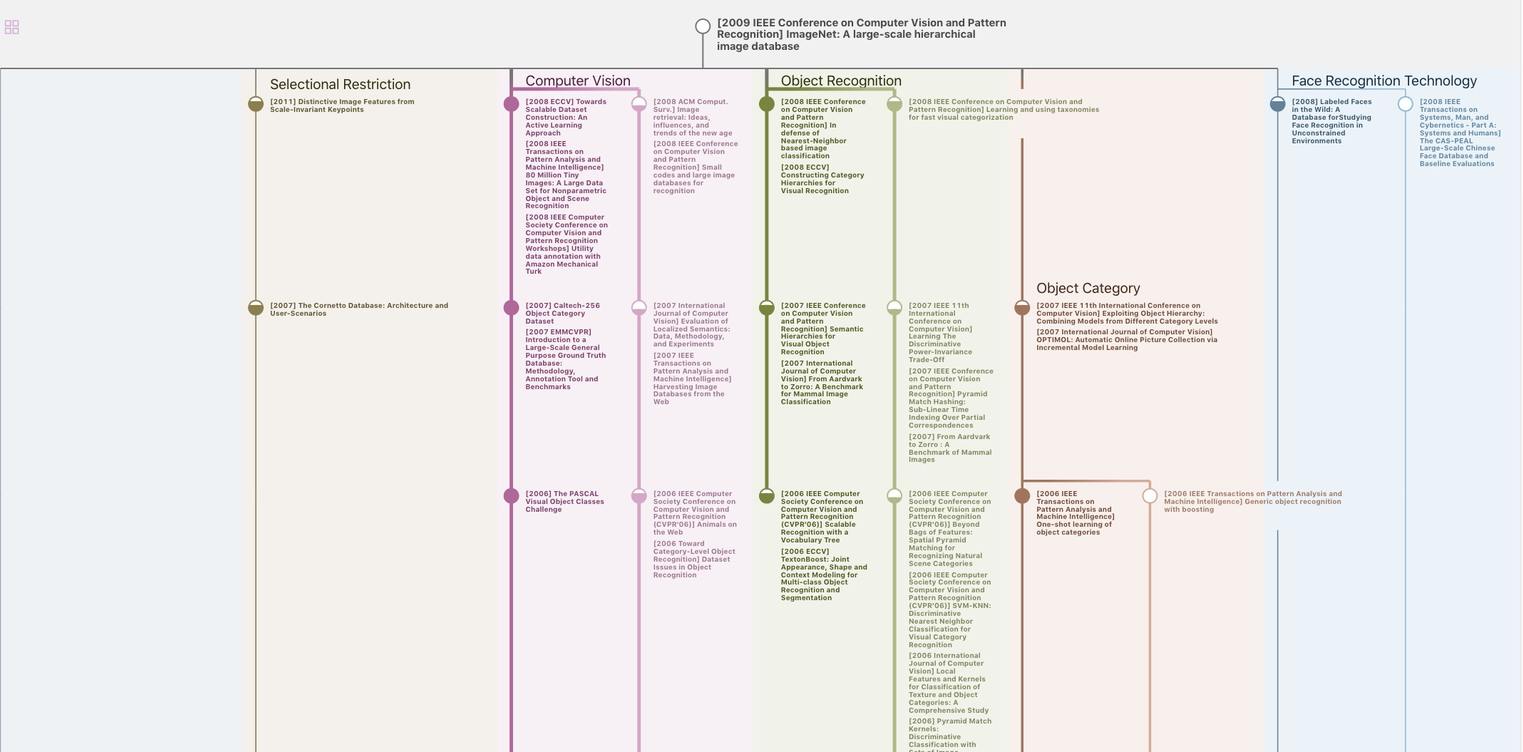
生成溯源树,研究论文发展脉络
Chat Paper
正在生成论文摘要