A framework for mining signatures from event sequences and its applications in healthcare data.
IEEE Trans. Pattern Anal. Mach. Intell.(2013)
摘要
This paper proposes a novel temporal knowledge representation and learning framework to perform large-scale temporal signature mining of longitudinal heterogeneous event data. The framework enables the representation, extraction, and mining of high-order latent event structure and relationships within single and multiple event sequences. The proposed knowledge representation maps the heterogeneous event sequences to a geometric image by encoding events as a structured spatial-temporal shape process. We present a doubly constrained convolutional sparse coding framework that learns interpretable and shift-invariant latent temporal event signatures. We show how to cope with the sparsity in the data as well as in the latent factor model by inducing a double sparsity constraint on the β-divergence to learn an overcomplete sparse latent factor model. A novel stochastic optimization scheme performs large-scale incremental learning of group-specific temporal event signatures. We validate the framework on synthetic data and on an electronic health record dataset.
更多查看译文
关键词
heterogeneous event sequence,event sequences,novel temporal knowledge representation,mining signatures,healthcare data,latent factor model,longitudinal heterogeneous event data,group-specific temporal event signature,encoding event,high-order latent event structure,temporal event signature,multiple event sequence,large-scale temporal signature mining,sparse coding,stochastic gradient descent,health care,stochastic programming,convolution,synthetic data,data mining,nonnegative matrix factorization,learning artificial intelligence,convergence,sparse matrices,knowledge representation
AI 理解论文
溯源树
样例
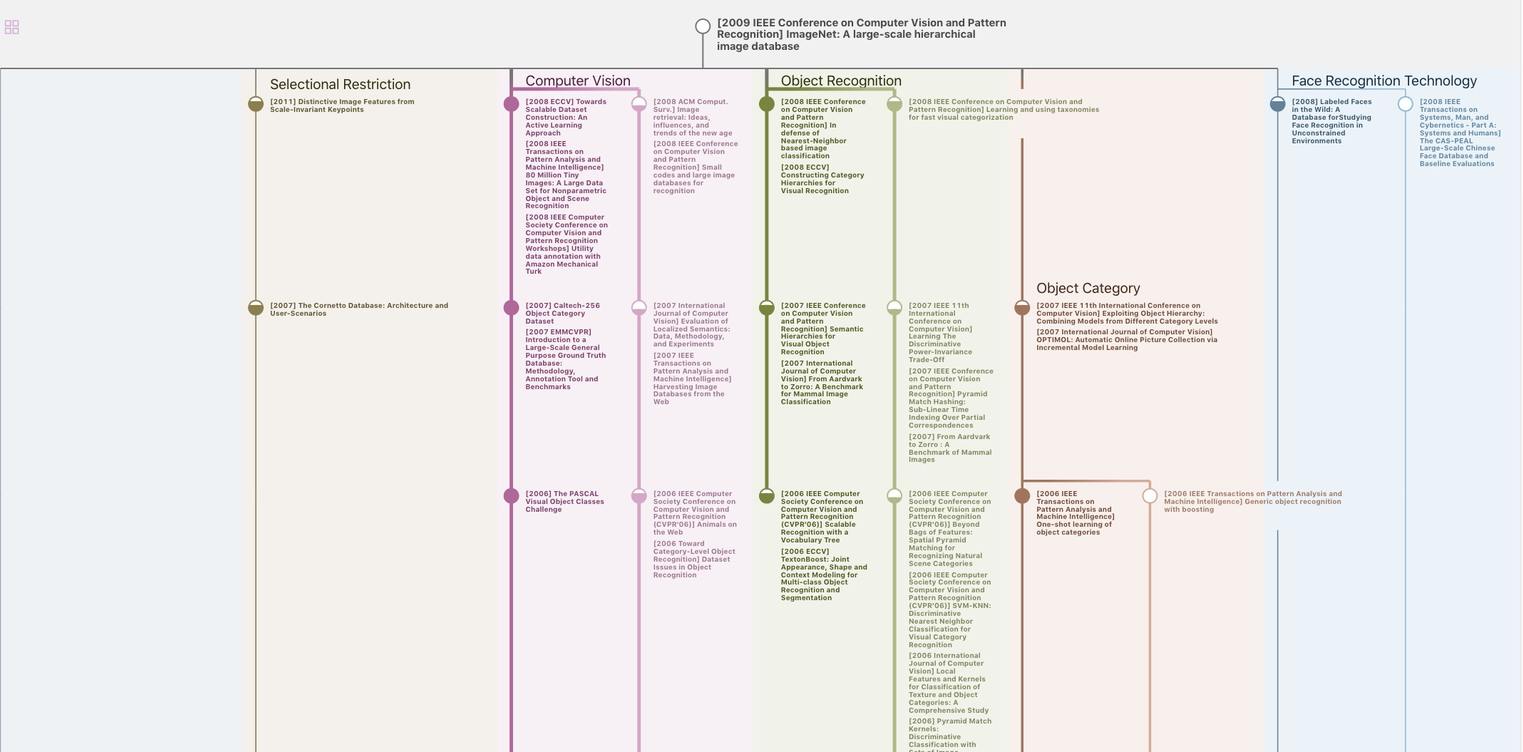
生成溯源树,研究论文发展脉络
Chat Paper
正在生成论文摘要