Factorization Models for Forecasting Student Performance
EDM(2011)
摘要
Predicting student performance, one of the tasks in educational data mining, has been taken into account recently [Toscher and Jahrer 2010; Yu et al. 2010; Cetintas et al. 2010; Thai-Nghe et al. 2011]. It was selected as a challenge task for the KDD Cup 2010 [Koedinger et al. 2010]. Concretely, predicting student performance is the task where we would like to know how the students learn (e.g. generally or narrowly), how quickly or slowly they adapt to new problems or if it is possible to infer the knowledge requirements to solve the problems directly from student performance data [Corbett and Anderson 1995; Feng et al. 2009], and eventually, we would like to know whether the students perform the tasks (exercises) correctly (or with some levels of certainty). As discussed in Cen et al. [2006], an improved model for predicting student performance could save millions of hours of students’ time and effort in learning algebra. In that time, students could move to other specific fields of their study or doing other things they enjoy. From educational data mining point of view, an accurate and reliable model in predicting student performance may replace some current standardized tests, and thus, reducing the pressure, time, as well as effort on “teaching and learning for examinations” [Feng et al. 2009; Thai-Nghe et al. 2011]. To address the problem of predicting student performance, many papers have been published but most of them are based on traditional classification/regression techniques [Cen et al. 2006; Feng et al. 2009; Yu et al. 2010; Pardos and Heffernan 2010]. Many other works can be found in Romero et al. [2010]. Recently, [Thai-Nghe et al. 2010; Toscher and Jahrer 2010; Thai-Nghe et al. 2011] have proposed using recommendation techniques, e.g. matrix factorization, for predicting student performance. The authors have shown that predicting student performance can be considered as rating prediction since the student, task, and performance would become user, item, and rating in recommender systems, respectively. We know that learning and problem-solving are complex cognitive and affective processes that are different to shopping and other e-commerce transactions, however, as discussed in Thai-Nghe et al. [2011], the factorization models in recommender systems are implicitly able to encode latent factors of students and tasks (e.g. “slip” and “guess”), and especially in case where we do not have enough meta data about students and tasks (or even we have not enough background knowledge of the domain), this mapping is a reasonable approach.
更多查看译文
关键词
factor model
AI 理解论文
溯源树
样例
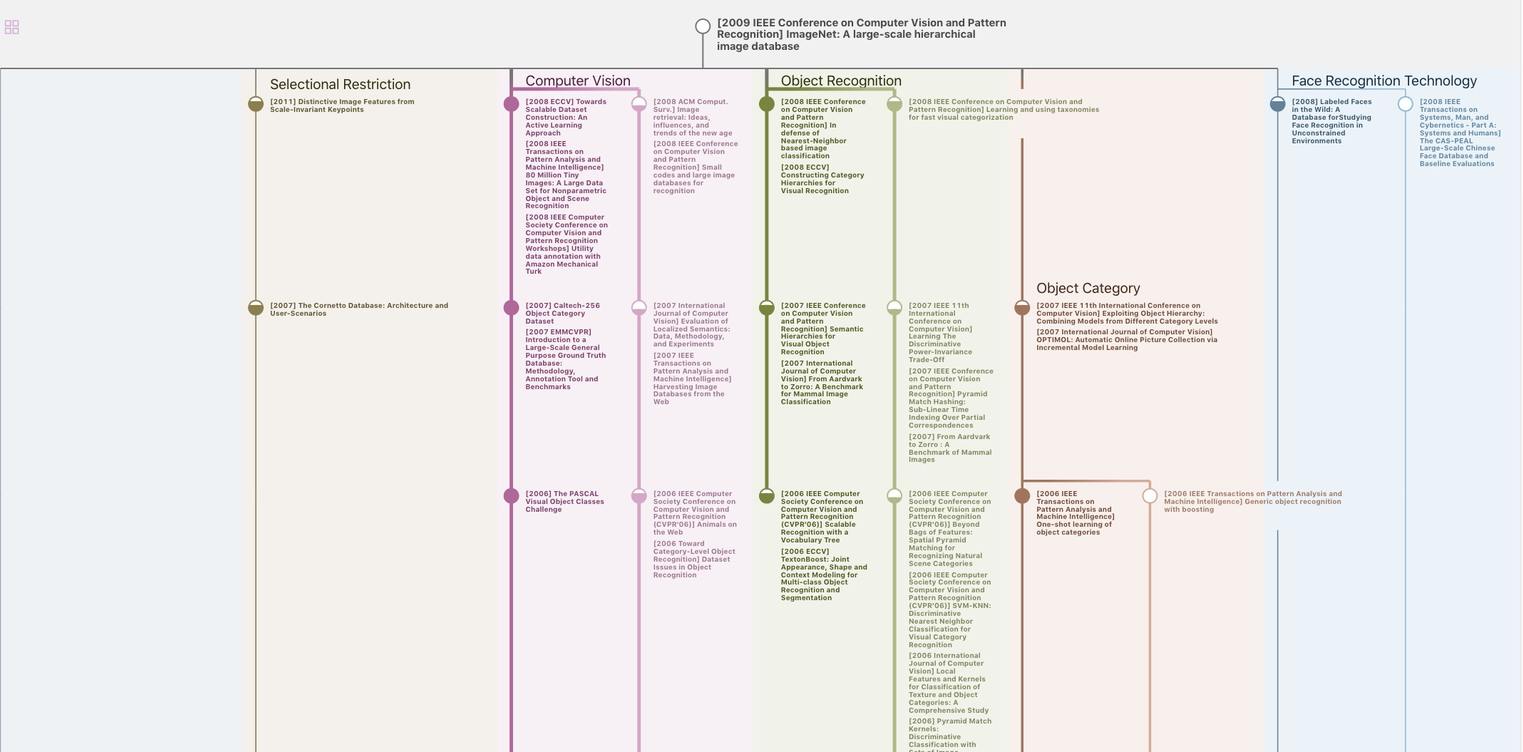
生成溯源树,研究论文发展脉络
Chat Paper
正在生成论文摘要