Online Bayesian Passive-Aggressive Learning
ICML'14: Proceedings of the 31st International Conference on International Conference on Machine Learning - Volume 32(2017)
摘要
We present online Bayesian Passive-Aggressive (BayesPA) learning, a generic online learning framework for hierarchical Bayesian models with max-margin posterior regularization. We show that BayesPA subsumes the standard online Passive-Aggressive (PA) learning and extends naturally to incorporate latent variables for both parametric and nonparametric Bayesian inference, therefore providing great flexibility for explorative analysis. As an important example, we apply BayesPA to topic modeling and derive efficient online learning algorithms for max-margin topic models. We further develop nonparametric BayesPA topic models to infer the unknown number of topics in an online manner. Experimental results on 20newsgroups and a large Wikipedia multi-label dataset (with 1.1 millions of training documents and 0.9 million of unique terms in the vocabulary) show that our approaches significantly improve time efficiency while achieving comparable accuracy with the corresponding batch algorithms.
更多查看译文
AI 理解论文
溯源树
样例
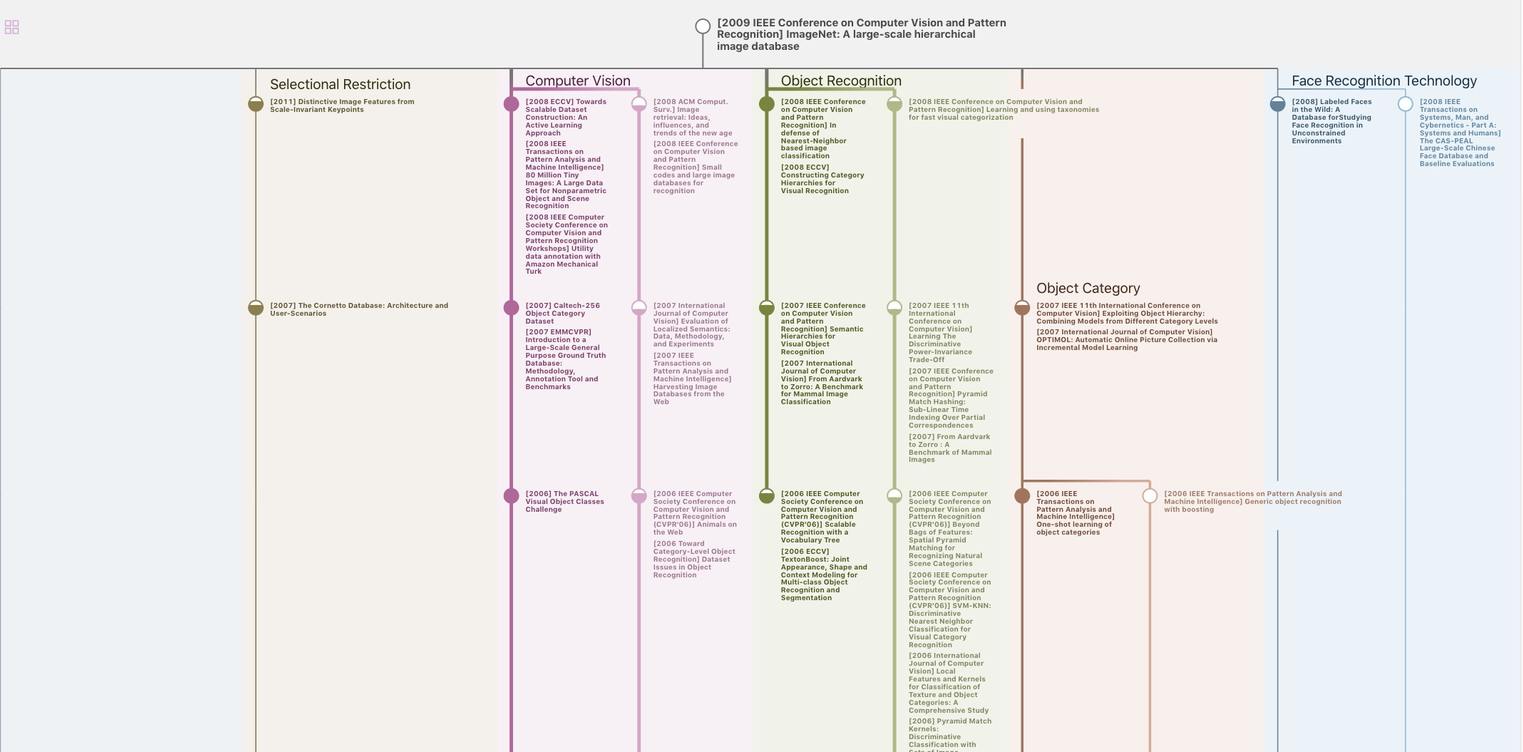
生成溯源树,研究论文发展脉络
Chat Paper
正在生成论文摘要