Social Media Annotation and Tagging Based on Folksonomy Link Prediction in a Tripartite Graph.
MMM(2013)
摘要
Social tagging has become a popular way for users to annotate, search, navigate and discover online social media, resulting in the sheer amount of metadata collectively generated by people. This paper focuses on two tagging problems—(1) recommending the most suitable tags during a user’s tagging process and (2) labeling latent tags relevant to a social media item—so that social media can be more browsable, searchable, and shareable by users. The proposed approach employs the Katz measure, a path-ensemble based proximity measure, to predict links in a weighted tripartite graph which represents folksonomy. From a graph-based proximity perspective, our method recommends appropriate tags for a given user-item pair, as well as uncovers hidden tags potentially relevant to a given item. We evaluate our method on real-world folksonomy collected from Last.fm. From our experiments, we show that not only does our algorithm outperform existing algorithms, but it can also obtain significant gains in cold start situations where relatively little information is known about a user or an item.
更多查看译文
关键词
folksonomy link prediction,tagging,social media
AI 理解论文
溯源树
样例
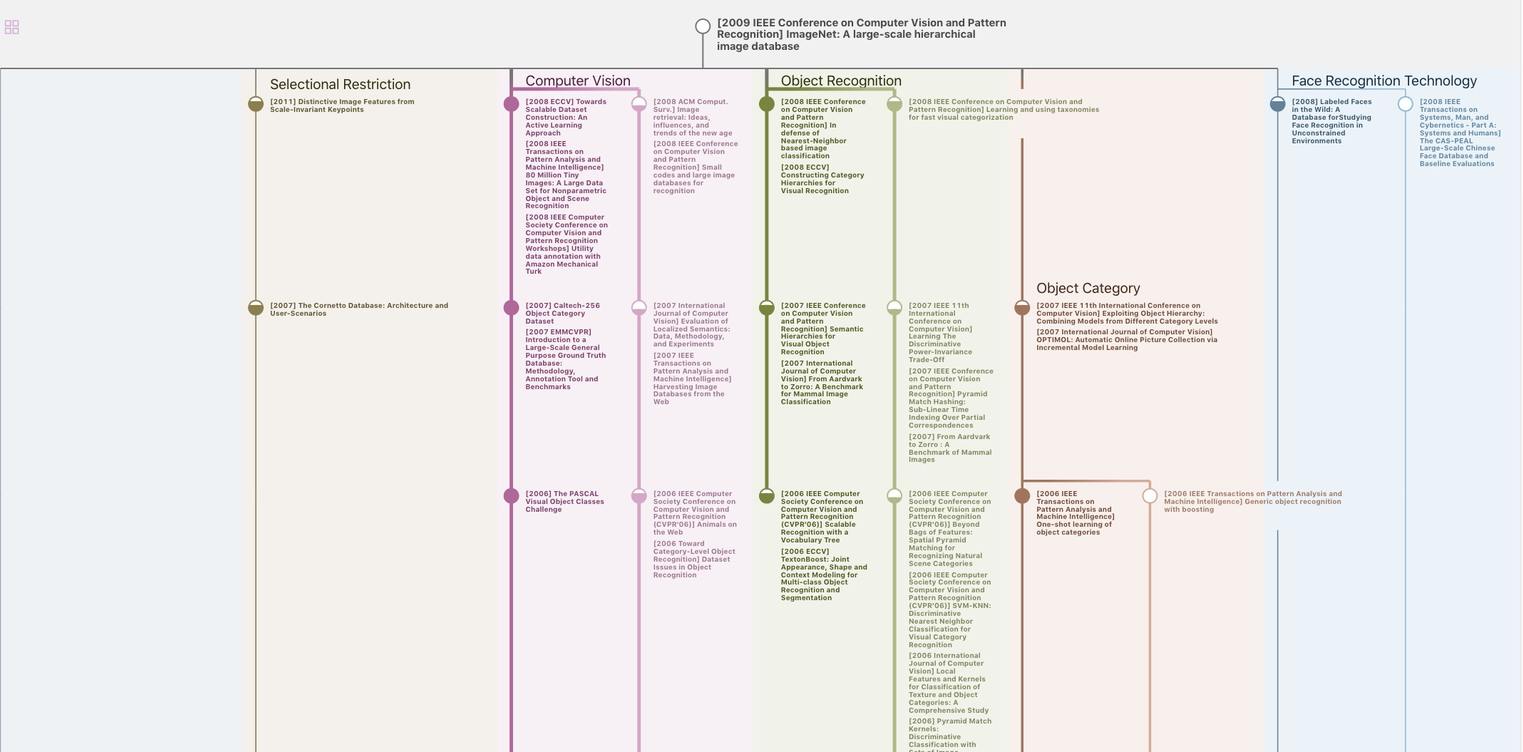
生成溯源树,研究论文发展脉络
Chat Paper
正在生成论文摘要