Online Learning For Collaborative Filtering
2012 INTERNATIONAL JOINT CONFERENCE ON NEURAL NETWORKS (IJCNN)(2012)
摘要
Collaborative filtering (CF), aiming at predicting users' unknown preferences based on observational preferences from some users, has become one of the most successful methods to building recommender systems. Various approaches to CF have been proposed in this area, but seldom do they consider the dynamic scenarios: 1) new items arriving in the system, 2) new users joining the system; or 3) new rating updating the system are all dynamically obtained with respect to time. To capture these changes, in this paper, we develop an online learning framework for collaborative filtering. Specifically, we construct this framework consisting of two state-of-the-art matrix factorization based CF methods: the probabilistic matrix factorization and the top-one probability based ranking matrix factorization. Moreover, we demonstrate that the proposed online algorithms bring several attractive advantages: 1) they scale linearly with the number of observed ratings and the size of latent features; 2) they obviate the need to load all ratings in memory; 3) they can adapt to new ratings easily. Finally, we conduct a series of detailed experiments on real-world datasets to demonstrate the merits of the proposed online learning algorithms under various settings.
更多查看译文
关键词
collaboration,recommender systems,entropy,matrix decomposition,probability,stochastic processes,tv,collaborative filtering,vectors
AI 理解论文
溯源树
样例
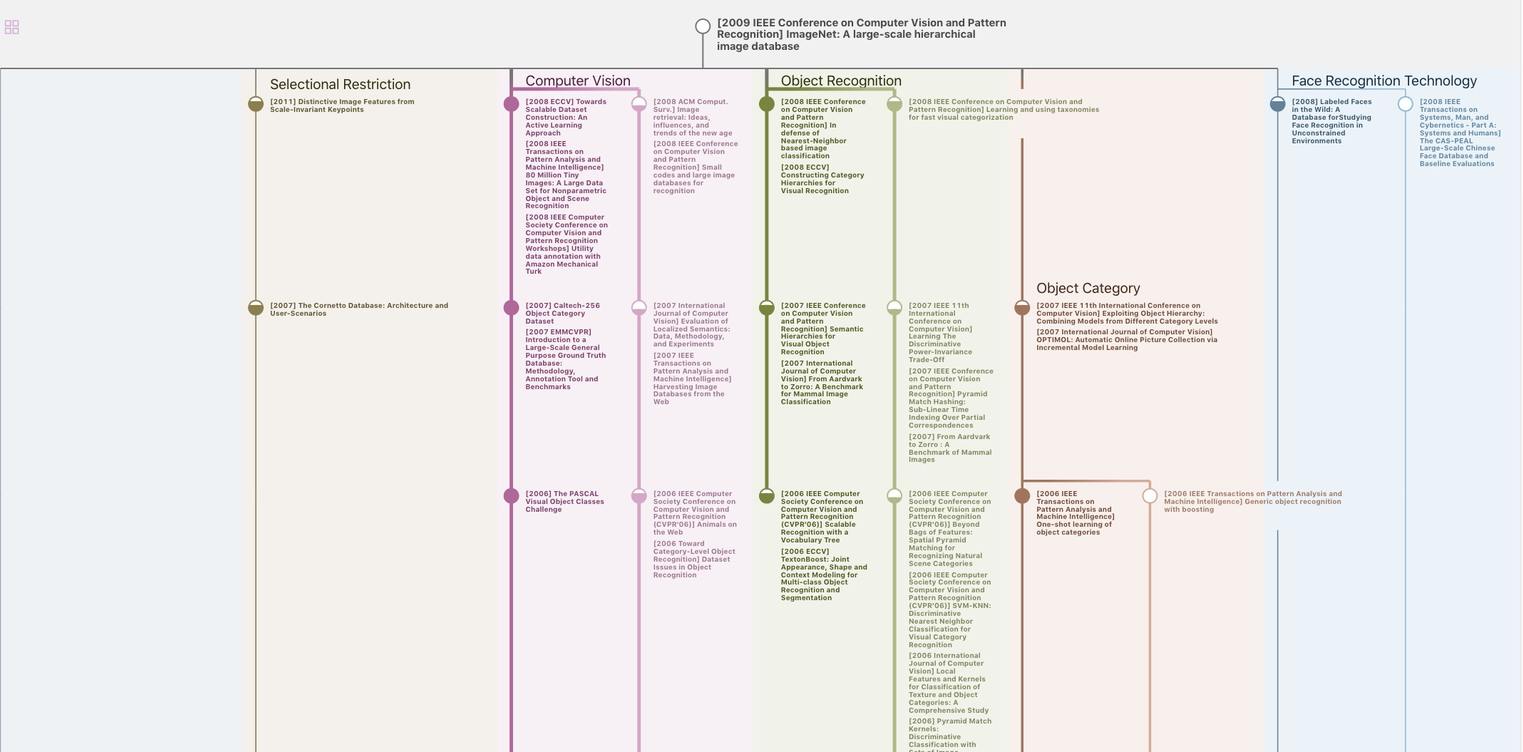
生成溯源树,研究论文发展脉络
Chat Paper
正在生成论文摘要