Novel Approach To Neural Network-Based Motion Cueing Algorithm For A Driving Simulator
2020 IEEE INTELLIGENT VEHICLES SYMPOSIUM (IV)(2020)
摘要
Generating realistic motion in a motion-based (dynamic) driving simulator is challenging due to the limited workspace of the motion system of the simulator compared to the motion range of the simulated vehicle. Motion Cueing Algorithms (MCAs) render accelerations by controlling the motion system of the simulators to provide the driver with a realistic driving experience. Commonly used methods such as Classical Washout-based MCA (CW-MCA) typically achieves suboptimal results due to scaling and filtering, which results in an inefficient usage of the workspace. The Model Predictive Control-based MCA (MPC-MCA) has been shown to achieve superior results and more efficient workspace use. However, it's performance is in practice constrained due to the computationally expensive operations and the requirement of an accurate prediction of future vehicle states. Finally, the Optimal Control (OC) has been shown to provide optimal cueing in an open-loop setup wherein the precalculated control signals are re-played to the driver. However, OC cannot be used in real-time with the driver-in-the-loop. Our work introduces a novel Neural Network-based MCA (NN-MCA), which is trained to imitate the behavior of the OC. After training, the NN-MCA provides an approximated model of the OC, which can run in real-time with the driver in-the-loop, while achieving similar quality. The experiments demonstrate the potential of this approach through objective evaluations of the generated motion-cues on the simulator model and the real simulator. A demonstration video for the performance comparison of the CW-MCA, Optimal-Control-based MCA (OC-MCA) and our proposed method is available at http://go.tum.de/708350.
更多查看译文
关键词
Neural Networks, Imitation Learning, Optimal Control, Motion Cueing Algorithms, Driving Simulators
AI 理解论文
溯源树
样例
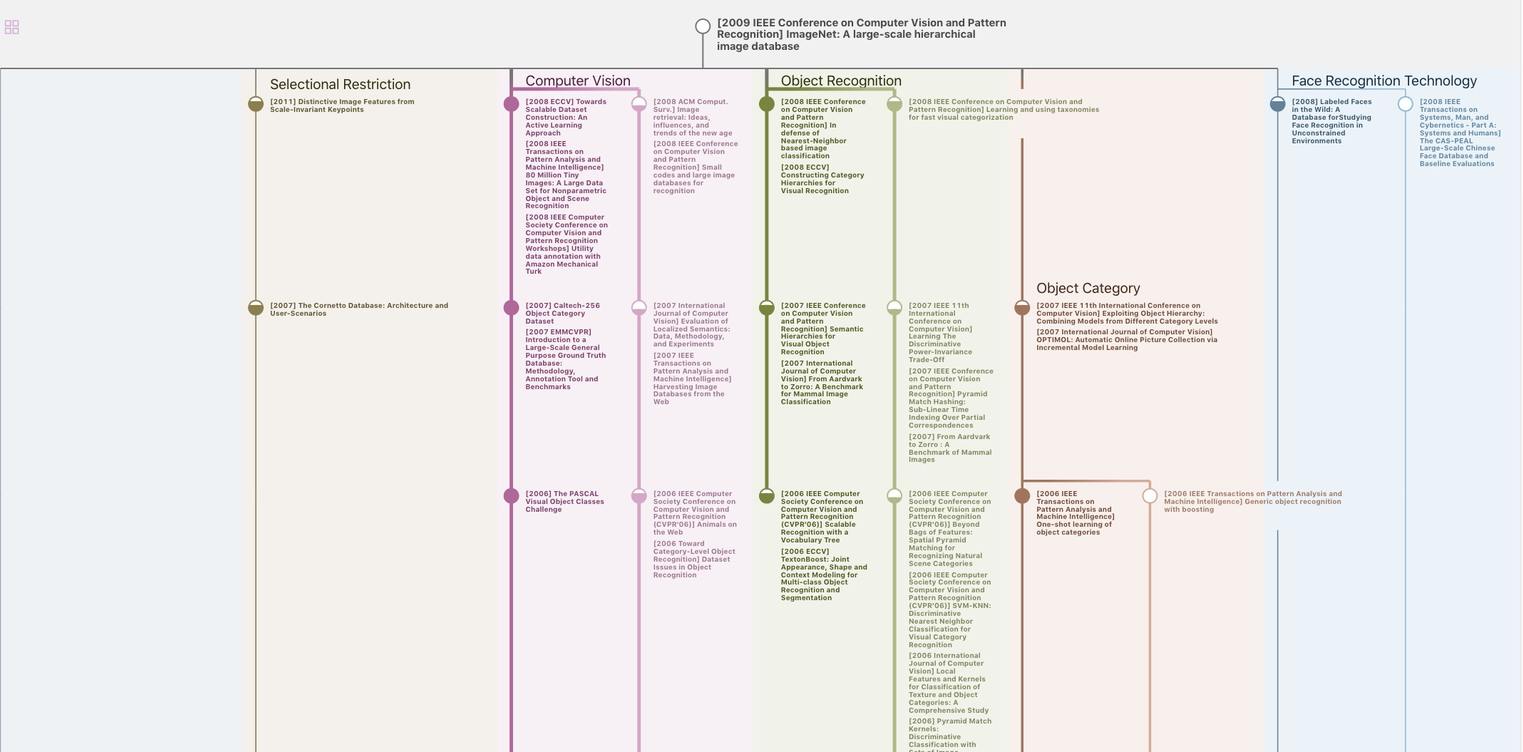
生成溯源树,研究论文发展脉络
Chat Paper
正在生成论文摘要