Provable Deterministic Leverage Score Sampling
KDD '14: The 20th ACM SIGKDD International Conference on Knowledge Discovery and Data Mining New York New York USA August, 2014(2014)
摘要
We explain theoretically a curious empirical phenomenon: "Approximating a matrix by deterministically selecting a subset of its columns with the corresponding largest leverage scores results in a good low-rank matrix surrogate". To obtain provable guarantees, previous work requires randomized sampling of the columns with probabilities proportional to their leverage scores. In this work, we provide a novel theoretical analysis of deterministic leverage score sampling. We show that such deterministic sampling can be provably as accurate as its randomized counterparts, if the leverage scores follow a moderately steep power-law decay. We support this power-law assumption by providing empirical evidence that such decay laws are abundant in real-world data sets. We then demonstrate empirically the performance of deterministic leverage score sampling, which many times matches or outperforms the state-of-the-art techniques.
更多查看译文
关键词
Subset selection,low-rank matrix approximation,leverage scores,deterministic sampling,power law distributions
AI 理解论文
溯源树
样例
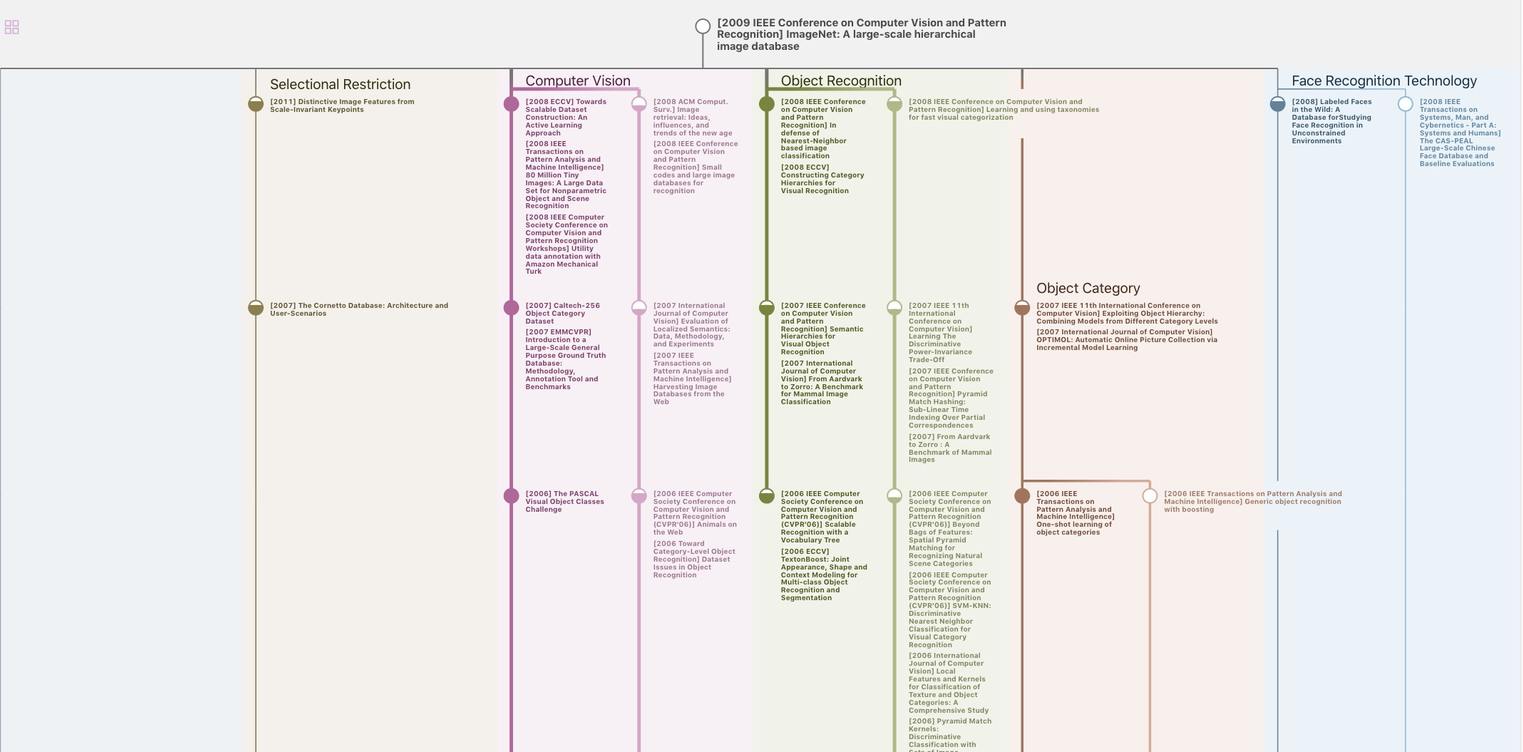
生成溯源树,研究论文发展脉络
Chat Paper
正在生成论文摘要