Semantics and aesthetics inference for image search: statistical learning approaches
Semantics and aesthetics inference for image search: statistical learning approaches(2009)
摘要
The automatic inference of image semantics is an important but highly challenging research problem whose solutions can greatly benefit content-based image search and automatic image annotation. In this thesis, I present algorithms and statistical models for inferring image semantics and aesthetics from visual content, specifically aimed at improving real-world image search. First, a novel approach to automatic image tagging is presented which furthers the state-of-the-art in both speed and accuracy. The direct use of automatically generated tags in real-world image search is then explored, and its efficacy demonstrated experimentally. An assumption which makes most annotation models misrepresent reality is that the state of the world is static, whereas it is fundamentally dynamic. I explore learning algorithms for adapting automatic tagging to different scenario changes. Specifically, a meta-learning model is proposed which can augment a black-box annotation model to help provide adaptability for personalization, time evolution, and contextual changes. Instead of retraining expensive annotation models, adaptability is achieved through efficient incremental learning of only the meta-learning component. Large scale experiments convincingly support this approach. In image search, when semantics alone yields many matches, one way to rank images further is to look beyond semantics and consider visual quality. I explore the topic of data-driven inference of aesthetic quality of images. Owing to minimal prior art, the topic is first explored in detail. Then, methods for extracting a number of high-level visual features, presumed to have correlation with aesthetics, are presented. Through feature selection and machine learning, an aesthetics inference model is trained and found to perform moderately on real-world data. The aesthetics-correlated visual features are then used in the problem of selecting and eliminating images at the high and low extremes of the aesthetics scale respectively, using a novel statistical model. Experimentally, this approach is found to work well in visual quality based filtering. Finally, I explore the use of image search techniques for designing a novel image-based CAPTCHA, a Web security test aimed at distinguishing humans from machines. Assuming image search metrics to be potential attack tools, they are used in the loop to design attack-resistant CAPTCHAs.
更多查看译文
关键词
real-world image search,statistical learning approach,image search technique,content-based image search,image search,automatic image tagging,image search metrics,inferring image semantics,aesthetics inference,automatic image annotation,image semantics,visual quality
AI 理解论文
溯源树
样例
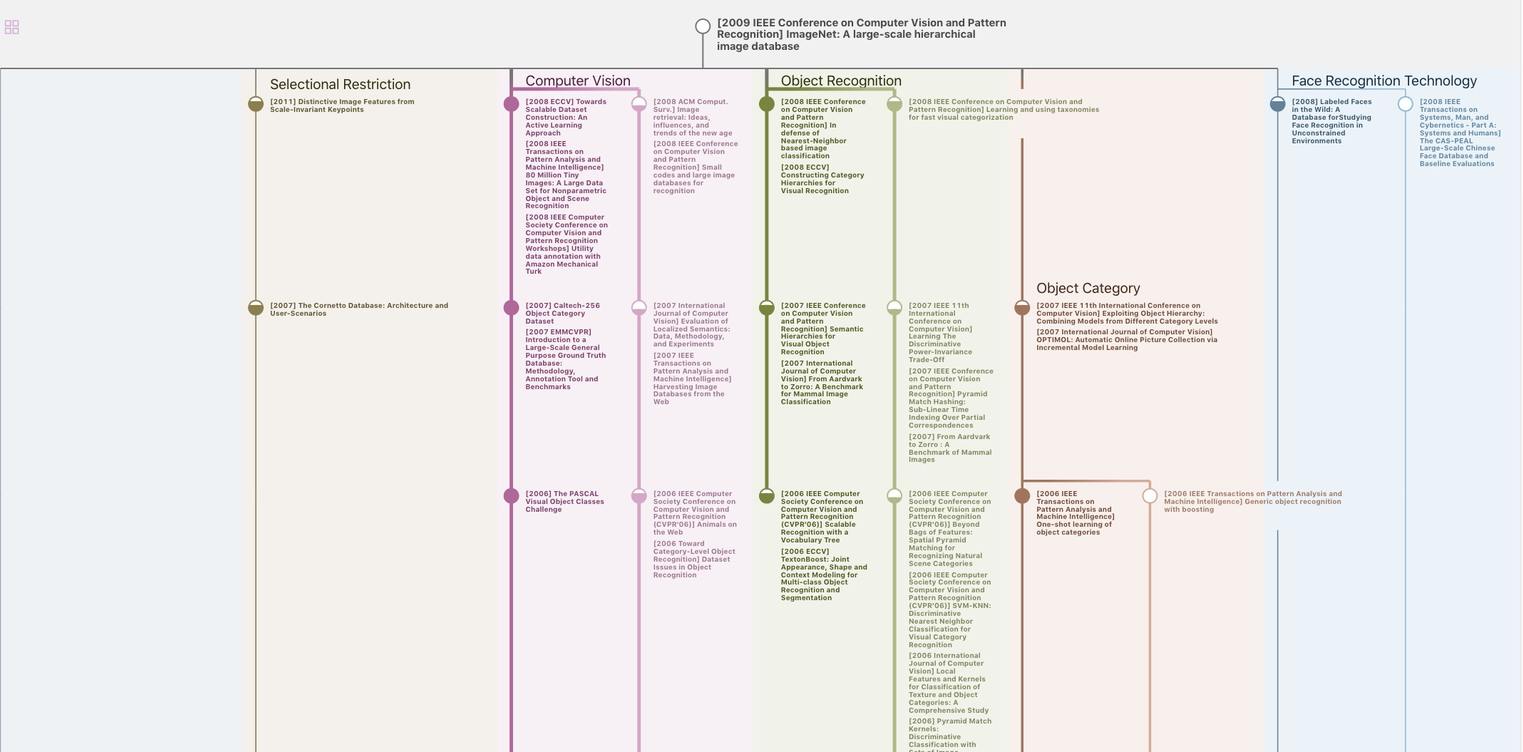
生成溯源树,研究论文发展脉络
Chat Paper
正在生成论文摘要