Spectro-temporal features for noise-robust speech recognition using power-law nonlinearity and power-bias subtraction
ICASSP(2013)
摘要
Previous work has demonstrated that spectro-temporal Gabor features reduced word error rates for automatic speech recognition under noisy conditions. However, the features based on mel spectra were easily corrupted in the presence of noise or channel distortion. We have exploited an algorithm for power normalized cepstral coefficients (PNCCs) to generate a more robust spectro-temporal representation. We refer to it as power normalized spectrum (PNS), and to the corresponding output processed by Gabor filters and MLP nonlinear weighting as PNS-Gabor. We show that the proposed feature outperforms state-of-the-art noise-robust features, ETSI-AFE and PNCC for both Aurora2 and a noisy version of the Wall Street Jounal (WSJ) corpus. A comparison of the individual processing steps of mel spectra and PNS shows that power bias subtraction is the most important aspect of PNS-Gabor features to provide an improvement over Mel-Gabor features. The result indicates that Gabor processing compensates the limitation of PNCC for channels with frequency-shift characteristic. Overall, PNS-Gabor features decrease the word error rate by 32% relative to MFCC and 13% relative to PNCC in Aurora2. For noisy WSJ, they decrease the word error rate by 30.9% relative to MFCC and 24.7% relative to PNCC.
更多查看译文
关键词
power-law nonlinearity,speech recognition,power normalized spectrum,power normalized cepstral coefficient,etsi-afe,gabor filters,frequency-shift characteristic,power-bias subtraction,word error rate,wall street jounal,pns-gabor,pncc,cepstral analysis,wsj corpus,noise-robust speech recognition,mlp nonlinear weighting,wsj,robust speech recognition,large vocabulary speech recognizion,gabor processing,mfcc,spectro-temporal feature,spectro-temporal features,automatic speech recognition,noise measurement,robustness,mel frequency cepstral coefficient,noise
AI 理解论文
溯源树
样例
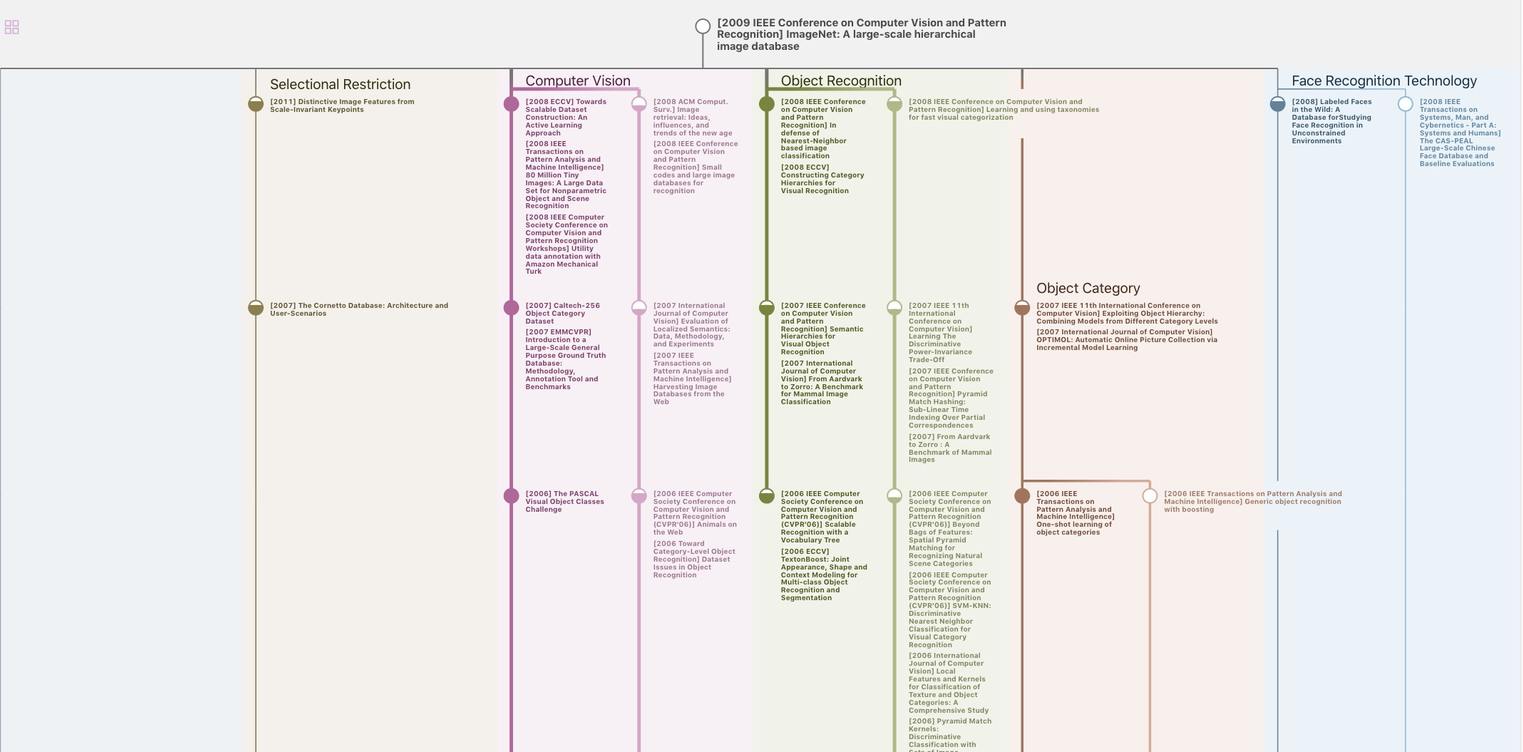
生成溯源树,研究论文发展脉络
Chat Paper
正在生成论文摘要