Parameter estimation in distributed hydrological modelling: comparison of global and local optimisation techniques
NORDIC HYDROLOGY(2007)
摘要
Much research has been spent in the last three decades in developing more effective and efficient automatic calibration procedures and in demonstrating their applicability to hydrological problems. Several problems have emerged when applying these procedures to calibration of conceptual rainfall-runoff and groundwater (GW) models, such as computational time, large number of calibration parameters, parameter identifiability, model response surface complexity, handling of multiple objectives and parameter equifinality. All these are expected to be much more severe for more complex models, for which comprehensive calibration studies have not so far been conducted. The scope of this paper is to investigate the performance of a global and a local optimisation technique, respectively, the Shuffled Complex Evolution algorithm and the gradient-based Gauss-Marquard-Levenberg algorithm, in calibration of physically based distributed models of different complexity. The models considered are a steady-state GW model, a transient GW model and a fully integrated model of the same catchment. The calibration is conducted in a multi-objective framework where two different aspects of the model response, the simulated runoff and the groundwater elevation are aggregated and simultaneously optimised. Different aggregated objective functions are used to give different weights to the calibration criteria. The results of the calibration procedures are compared in terms of effectiveness and efficiency and demonstrate the different performance of the methods. Moreover, a combination of the global and local techniques is investigated as an attempt to exploit the advantages of both procedures, while overcoming their drawbacks.
更多查看译文
关键词
distributed hydrological models,global and local optimisation methods,multi-objective calibration,parameter estimation
AI 理解论文
溯源树
样例
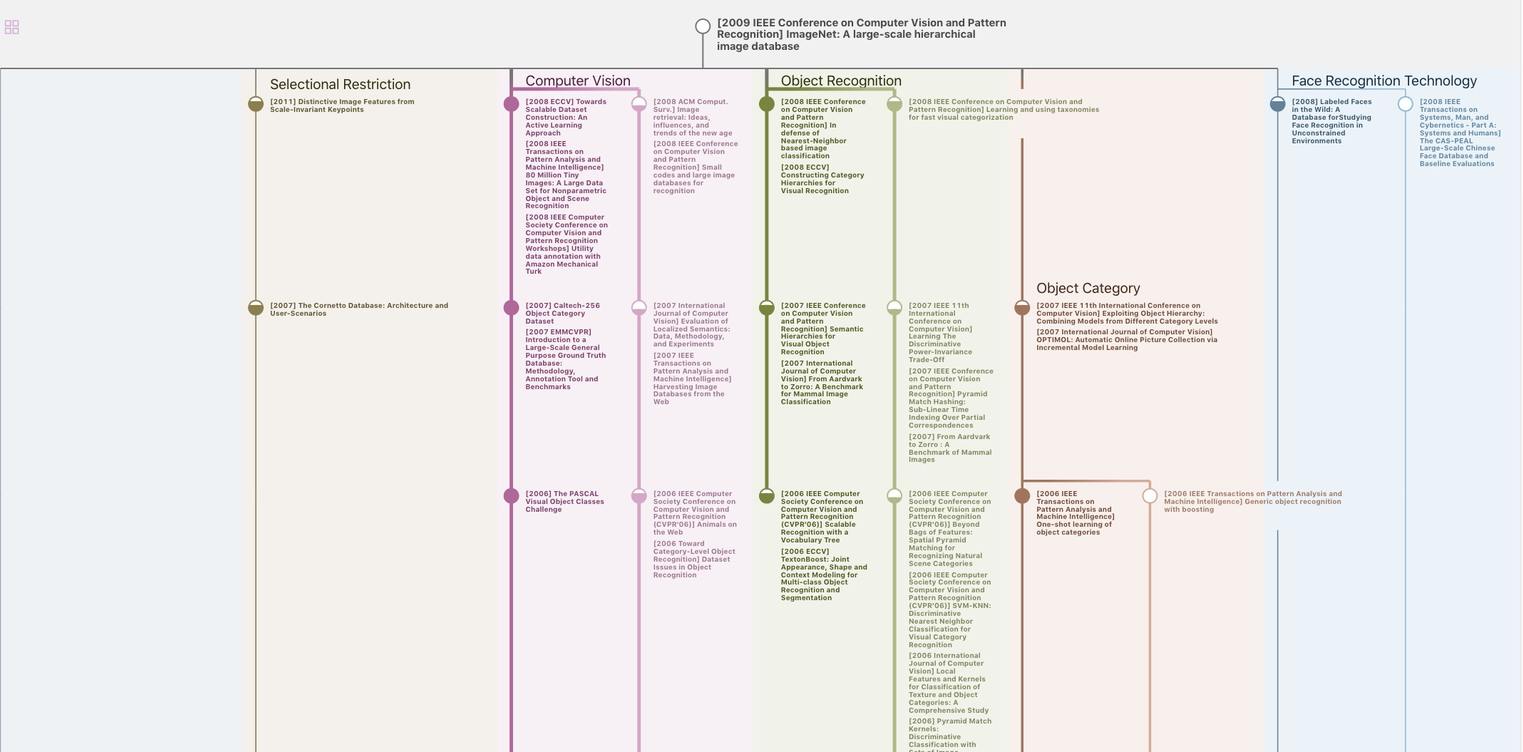
生成溯源树,研究论文发展脉络
Chat Paper
正在生成论文摘要