Parameter learning for alpha integration.
Neural Computation(2013)
摘要
In pattern recognition, data integration is an important issue, and when properly done, it can lead to improved performance. Also, data integration can be used to help model and understand multimodal processing in the brain. Amari proposed α-integration as a principled way of blending multiple positive measures (e.g., stochastic models in the form of probability distributions), enabling an optimal integration in the sense of minimizing the α-divergence. It also encompasses existing integration methods as its special case, for example, a weighted average and an exponential mixture. The parameter α determines integration characteristics, and the weight vector w assigns the degree of importance to each measure. In most work, however, α and w are given in advance rather than learned. In this letter, we present a parameter learning algorithm for learning α and ω from data when multiple integrated target values are available. Numerical experiments on synthetic as well as real-world data demonstrate the effectiveness of the proposed method.
更多查看译文
关键词
important issue,real-world data,exponential mixture,integration characteristic,multiple integrated target value,integration method,multiple positive measure,alpha integration,data integration,optimal integration
AI 理解论文
溯源树
样例
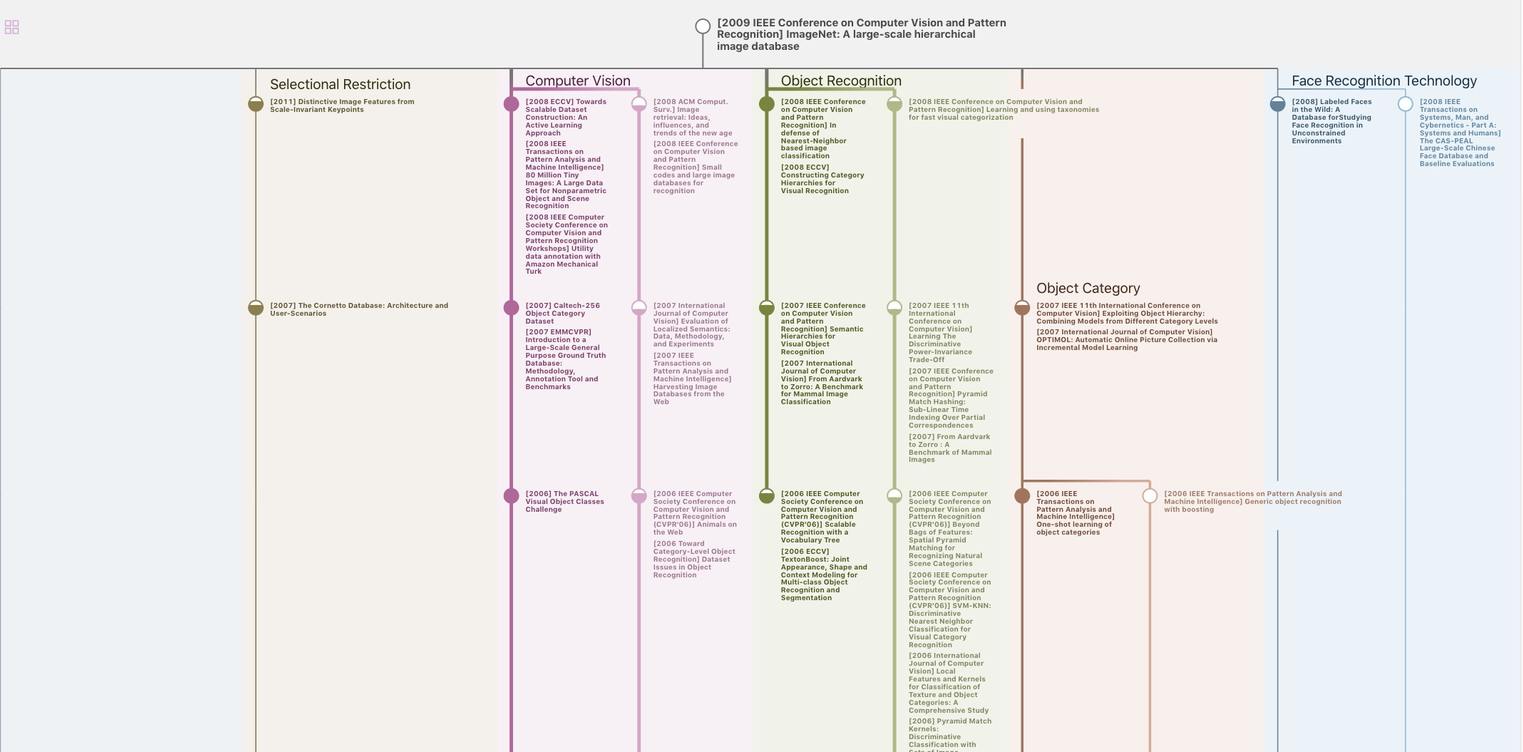
生成溯源树,研究论文发展脉络
Chat Paper
正在生成论文摘要