Hyperspectral Image Classification Using Spectral And Spatial Information Based Linear Discriminant Analysis
2011 IEEE INTERNATIONAL GEOSCIENCE AND REMOTE SENSING SYMPOSIUM (IGARSS)(2011)
摘要
Feature extraction plays an essential role in Hyperspectral image classification. Linear discriminant analysis (LDA) is a commonly used feature extraction (FE) method to resolve the Hughes phenomenon for classification. The Hughes phenomenon (also called the curse of dimensionality) is often encountered in classification when the dimensionality of the space grows and the size of the training set is fixed, especially in the small sampling size problem. Recent studies show that the spatial information can greatly improve the classification performance. Hence, for hyperspectral image classification, it is not only necessary to use the available spectral information but also to exploit the spatial information. In this paper, spatial information is acquired by the concept of the Markov random field (MRF), and this spatial information is used to form the membership values of every pixel in the hyperspectral image. The experimental results on two hyperspectral images, the Washington DC Mall and the Indian Pine Site, show that the proposed method can yield a better classification performance than LDA in the small sampling size problem.
更多查看译文
关键词
feature extraction,linear discriminant analysis
AI 理解论文
溯源树
样例
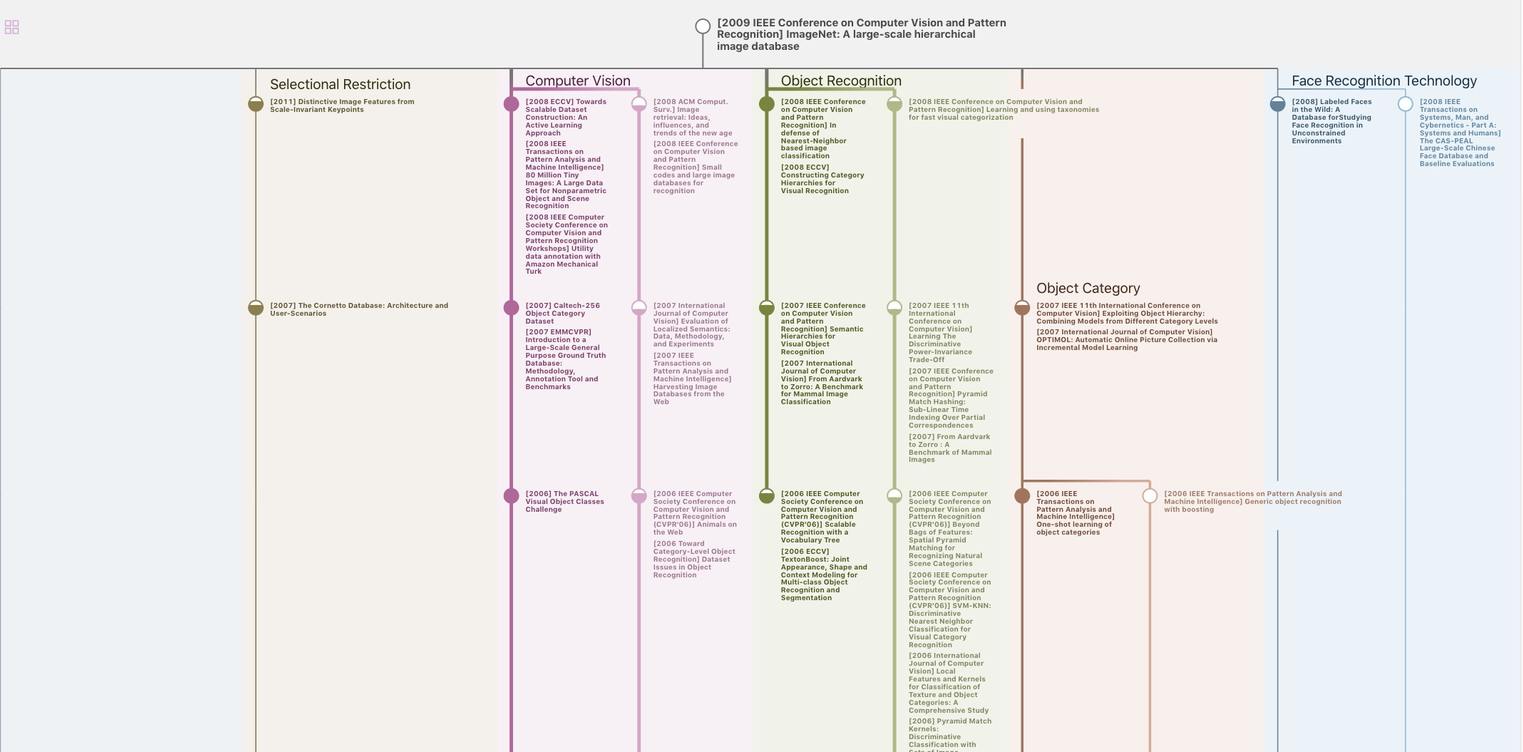
生成溯源树,研究论文发展脉络
Chat Paper
正在生成论文摘要