Improving The Robustness To Outliers Of Mixtures Of Probabilistic Pcas
PAKDD'08: Proceedings of the 12th Pacific-Asia conference on Advances in knowledge discovery and data mining(2008)
摘要
Principal Component Analysis, when formulated as a probabilistic model, can be made robust to outliers by using a Student-t assumption on the noise distribution instead of a Gaussian one. On the other hand, mixtures of PCA is a model aimed to discover nonlinear dependencies in data by finding clusters and identifying local linear sub-manifolds. This paper shows how mixtures of PCA can be made robust to outliers too. Using a hierarchical probabilistic model, parameters are set by likelihood maximization. The method is shown to be effectively robust to outliers, even in the context of high-dimensional data.
更多查看译文
关键词
hierarchical probabilistic model,probabilistic model,high-dimensional data,Principal Component Analysis,Student-t assumption,likelihood maximization,local linear submanifolds,noise distribution,nonlinear dependency,probabilistic PCAs
AI 理解论文
溯源树
样例
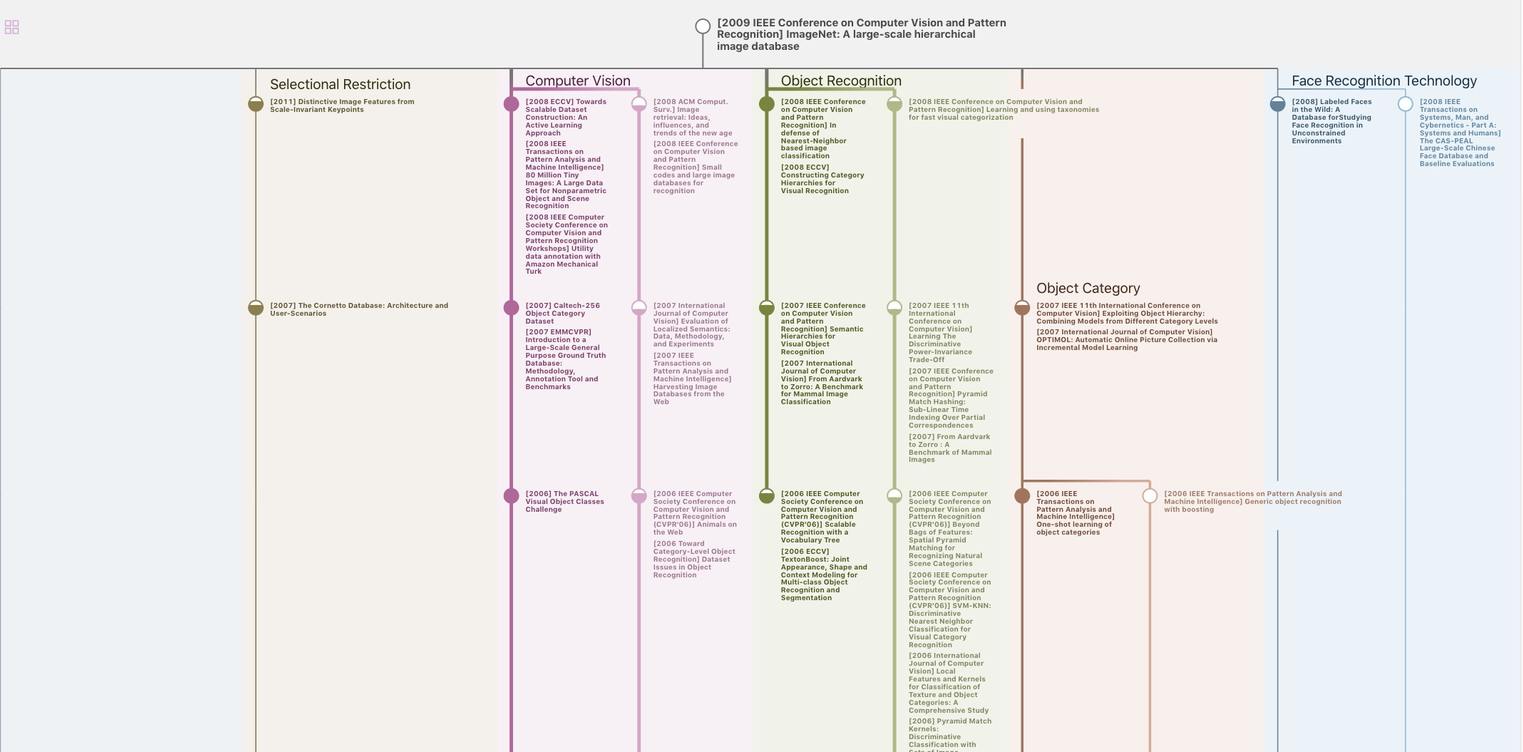
生成溯源树,研究论文发展脉络
Chat Paper
正在生成论文摘要