Unified Blind Method for Multi-Image Super-Resolution and Single/Multi-Image Blur Deconvolution
IEEE Transactions on Image Processing(2013)
摘要
This paper presents, for the first time, a unified blind method for multi-image super-resolution (MISR or SR), single-image blur deconvolution (SIBD), and multi-image blur deconvolution (MIBD) of low-resolution (LR) images degraded by linear space-invariant (LSI) blur, aliasing, and additive white Gaussian noise (AWGN). The proposed approach is based on alternating minimization (AM) of a new cost function with respect to the unknown high-resolution (HR) image and blurs. The regularization term for the HR image is based upon the Huber-Markov random field (HMRF) model, which is a type of variational integral that exploits the piecewise smooth nature of the HR image. The blur estimation process is supported by an edge-emphasizing smoothing operation, which improves the quality of blur estimates by enhancing strong soft edges toward step edges, while filtering out weak structures. The parameters are updated gradually so that the number of salient edges used for blur estimation increases at each iteration. For better performance, the blur estimation is done in the filter domain rather than the pixel domain, i.e., using the gradients of the LR and HR images. The regularization term for the blur is Gaussian (L2 norm), which allows for fast noniterative optimization in the frequency domain. We accelerate the processing time of SR reconstruction by separating the upsampling and registration processes from the optimization procedure. Simulation results on both synthetic and real-life images (from a novel computational imager) confirm the robustness and effectiveness of the proposed method.
更多查看译文
关键词
super resolution,computer simulation,image resolution,iterative methods,photography,diagnostic imaging,computational imaging,random processes,image restoration,markov chains,optimization,additive white gaussian noise,estimation theory,frequency domain analysis,noise,image reconstruction,hr,markov processes,minimisation,deconvolution,estimation,awgn,image registration,algorithms
AI 理解论文
溯源树
样例
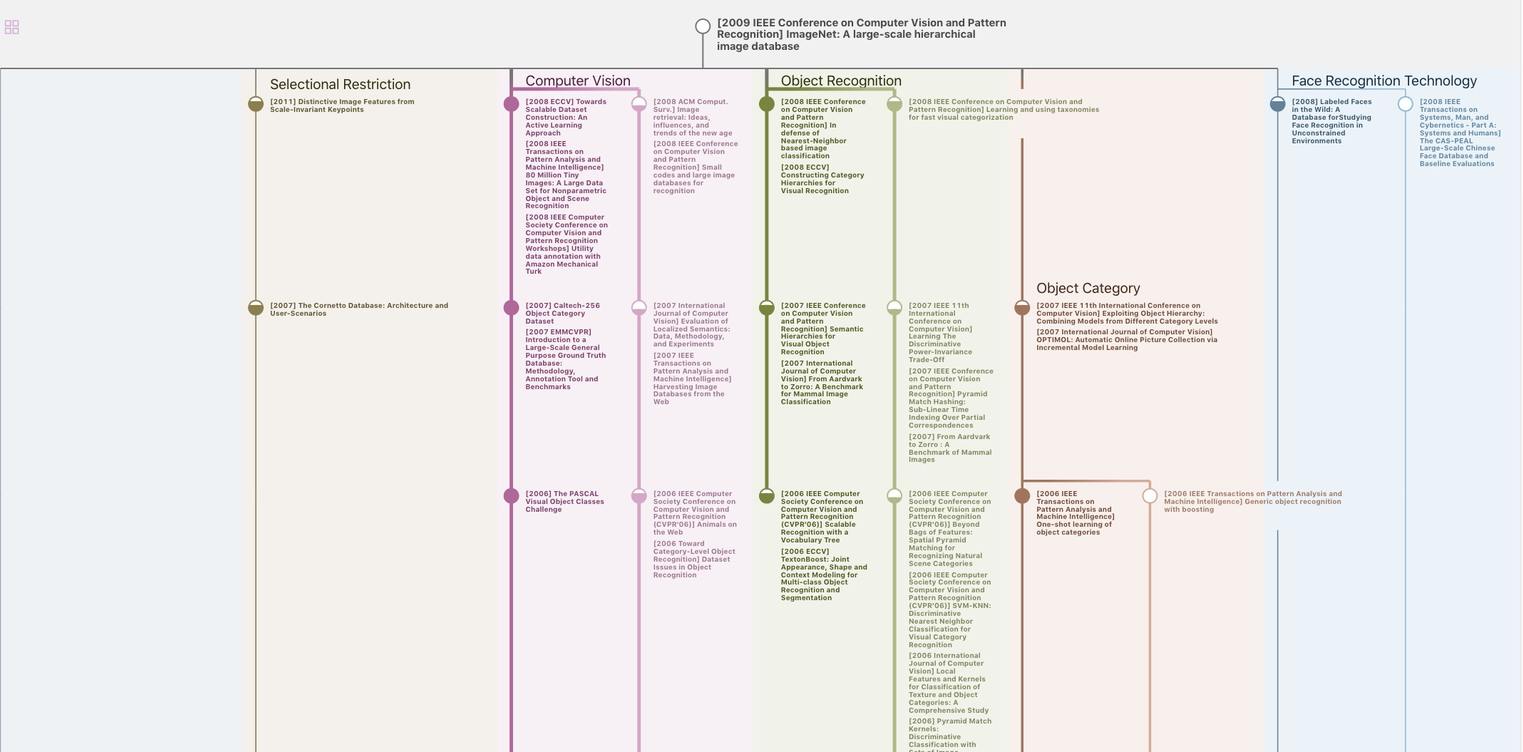
生成溯源树,研究论文发展脉络
Chat Paper
正在生成论文摘要