On The Use Of Nonlinear Polynomial Kernel Svms In Language Recognition
13TH ANNUAL CONFERENCE OF THE INTERNATIONAL SPEECH COMMUNICATION ASSOCIATION 2012 (INTERSPEECH 2012), VOLS 1-3(2012)
摘要
Reduced-dimensional supervector representations are shown to outperform their supervector counterparts in a variety of speaker recognition tasks. They have been exploited in automatic language verification (ALV) tasks as well but, to the best of our knowledge, their performance is comparable with their supervector counterparts. This paper demonstrates that nonlinear polynomial kernel support vector machines (SVMs) trained with low dimensional supervector representations almost halve the equal error rate (EER) of the SVMs trained with supervectors. Principal component analysis (PCA) is typically used for dimension reduction in ALV. Nonlinear kernel SVMs then implicitly transform these low dimensional representations onto higher-dimensional spaces. Unlike linear kernels, the transformations onto high dimensional feature spaces exploit the language-specific dependencies across different input dimensions. Mapping input training examples onto higher dimensional feature spaces is known to be generally effective when the number of instances is much larger than the input dimensionality. Our experiments demonstrate that fifth-order polynomial kernel SVMs trained with low dimensional representations reduce the ERR by 56% relative when compared to linear SVMs trained with supervectors, and by 40% relative to nonlinear SVMs trained with supervectors. Furthermore, they also reduce the ERR of linear kernel SVMs trained with the low dimensional representations by 71% relative.
更多查看译文
关键词
language recognition,support vector machines
AI 理解论文
溯源树
样例
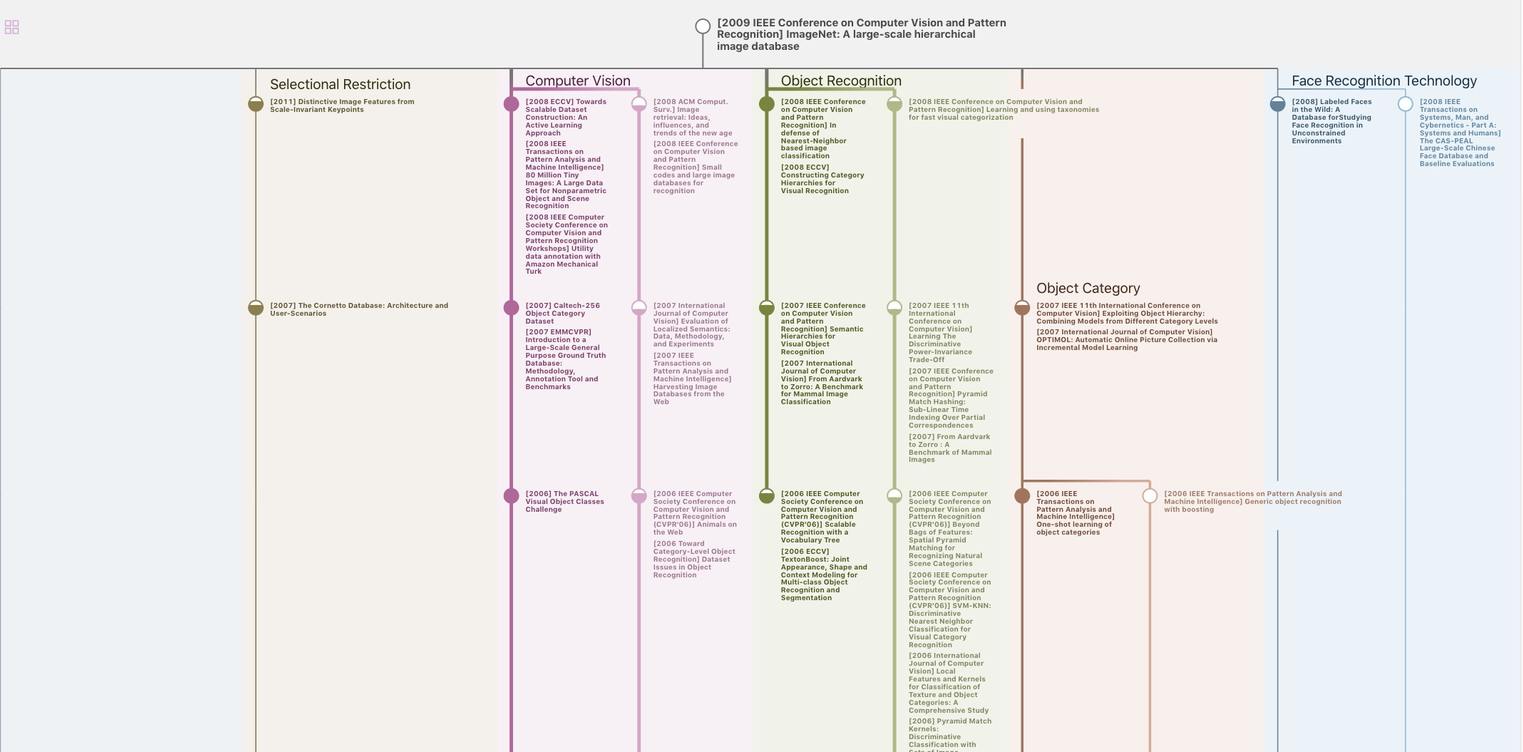
生成溯源树,研究论文发展脉络
Chat Paper
正在生成论文摘要