Learning contextually-aware dynamic models
Learning contextually-aware dynamic models(2011)
摘要
Modern information systems produce time series data that reflects the behavior of entities, such as people, computers, and organizations, as they interacting in a common environment. In many of these systems, much of the exploitable information is mutual information shared among the entities in the form of business processes typical to the environment. We demonstrate that the failure to model such interdependencies of entities can result in degradation in the performance of models. Dynamic system models have historically focused on the independent modeling of atomic processes. This modeling is a result of the early applications—tracking entities such as aircraft through RADAR and SONAR. In these scenarios, the mutual information among the entities was assumed negligible when compared to the quality of sensors and individual dynamics; these models made no use of cross entity dependencies. Our intent is to develop new models and associated learning techniques that are both dynamic and capable of capturing dependencies among entities. To this end, we construct models that lie at the intersection of Dynamic Bayesian Networks and mixture and feature model representations. We first develop a dynamic mixture model, the multi-Hidden Markov Model, which models a set of observation sequences where each is influenced by a single latent sequence. We then we describe the development of the multi-Factorial HMM, a feature model in which each observation sequence is influenced by multiple latent sequences. A key contribution is the development of learning algorithms presented in a “Bayesian non-parametric” setting that allows the number of latent features to scale in a data-driven manner. Experimental results are presented on synthetic data, Twitter activity data, and a college campus' wireless access point logs. These results demonstrate the semantic fit of the models to real data and their ability to detect anomalous data.
更多查看译文
关键词
twitter activity data,anomalous data,contextually-aware dynamic model,mutual information,exploitable information,time series data,observation sequence,dynamic mixture model,synthetic data,real data,dynamic system model
AI 理解论文
溯源树
样例
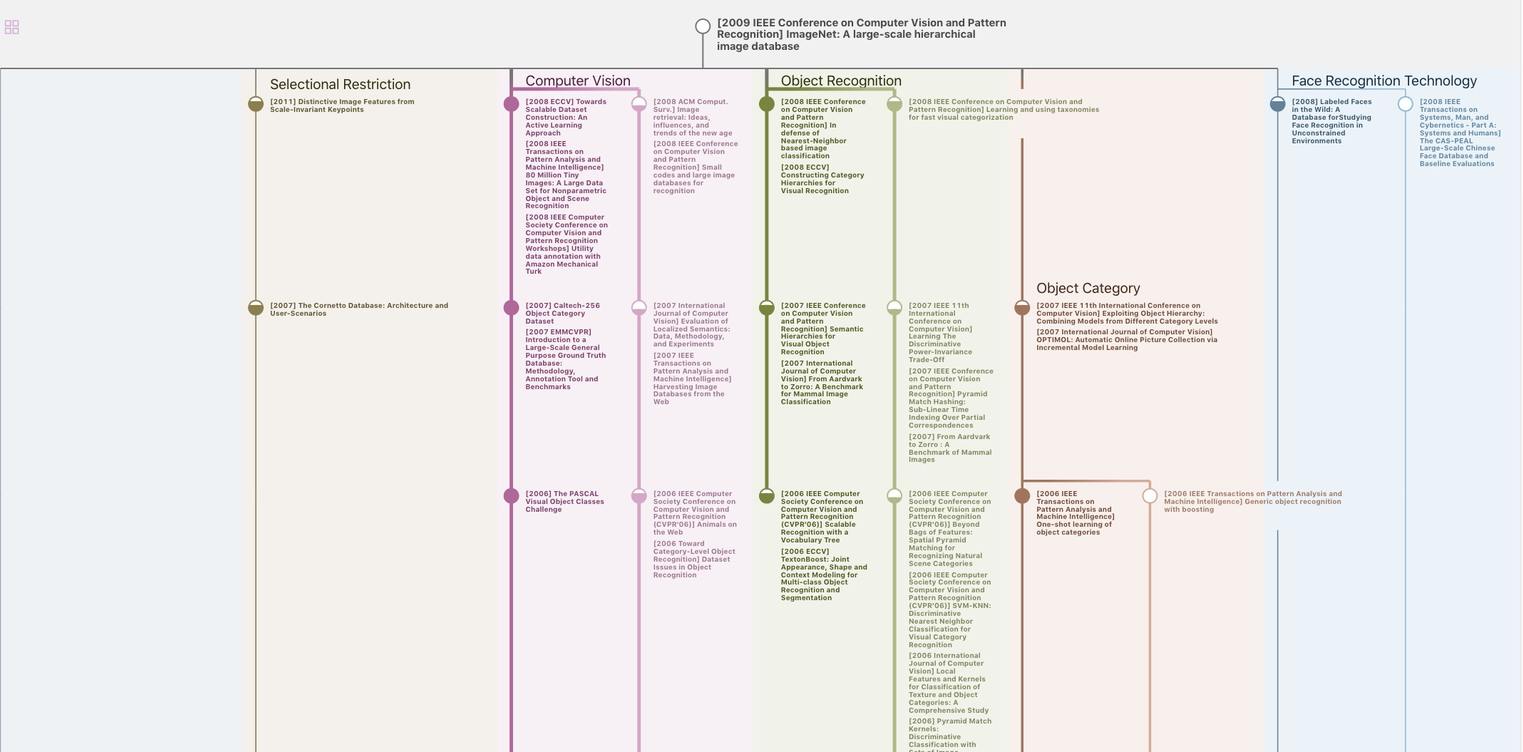
生成溯源树,研究论文发展脉络
Chat Paper
正在生成论文摘要