Adaptive Weighted Least Squares Svm Based Snowing Model For Image Denoising
INTERNATIONAL JOURNAL OF WAVELETS MULTIRESOLUTION AND INFORMATION PROCESSING(2013)
摘要
We propose a snowing model to iteratively smoothe the various image noises while preserving the important image structures such as edges and lines. Considering the gray image as a digital terrain model, we develop an adaptive weighted least squares support vector machine (LS-SVM) to iteratively estimate the optimal gray surface underlying the noisy image. The LS-SVM works on Gaussian noise while the weighted LS-SVM works on the outliers and non-Gaussian noise. To improve its performance in preserving the directional signal while suppressing the noise, the dominant orientation information of the gradients is integrated into the weighting scheme. The contribution of each attribute to the final LS-SVM model is adaptively determined in a fitness modulated, elongated, elliptical contour spread along the direction of the local edge structure. The farther away from the hyperplane in kernel space the point is, the less weight it gets, while the point on the direction of the local structure gets more weight. With adaptive weighting scheme, the robust LS-SVM smoothes most strongly along the edges, rather than across them, while it minimizes the effects of outliers, and results in strong preservation of details in the final output. The iteratively adaptive reweighted LS-SVM simulated the snowing process. The investigation on real images contaminated by mixture Gaussian noise has demonstrated that the performance of the present method is stable and reliable under noise distributions varying from Gaussian to impulsive.
更多查看译文
关键词
Snowing model, image denoising, weighted least squares SVM, directional support value transform (SVT), support value matrix
AI 理解论文
溯源树
样例
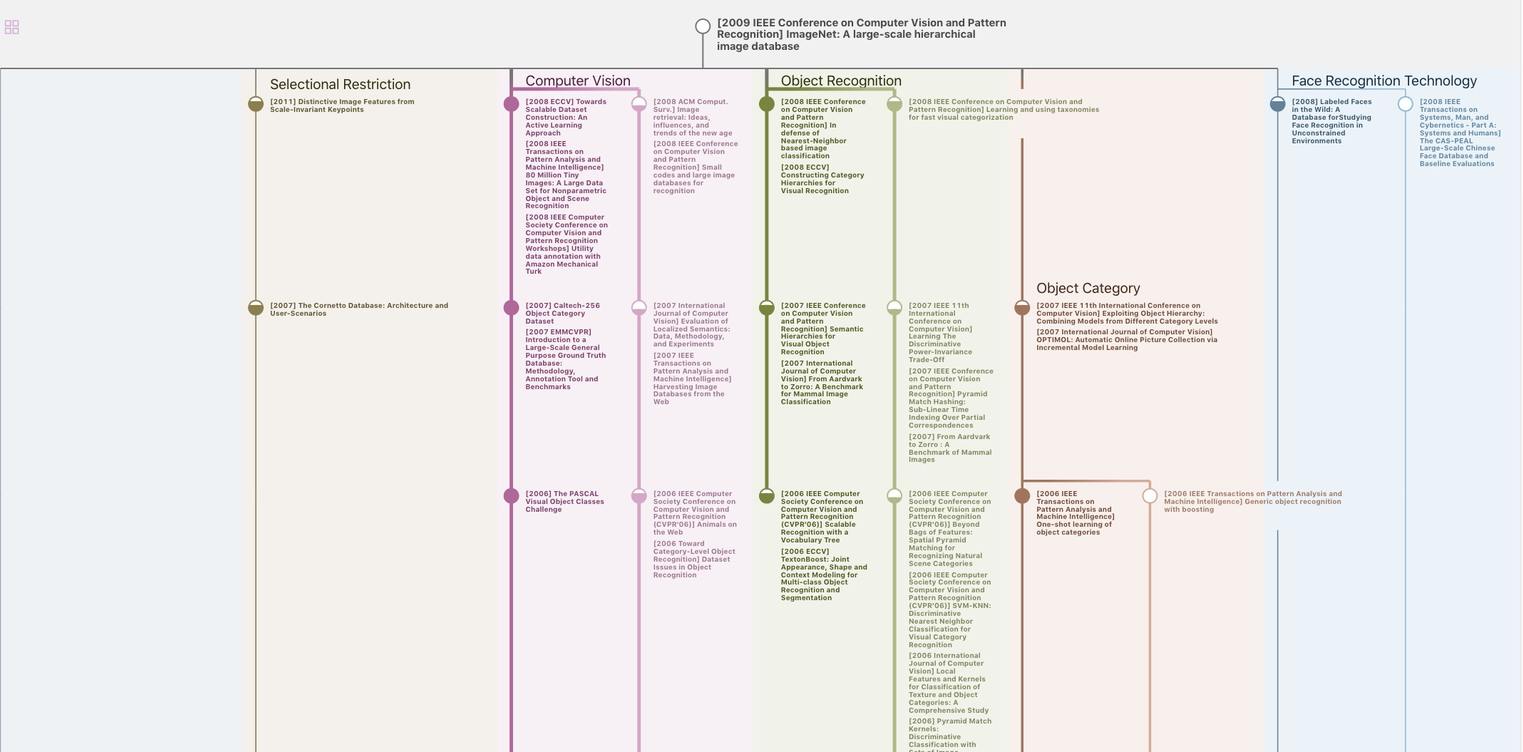
生成溯源树,研究论文发展脉络
Chat Paper
正在生成论文摘要