A Study on Expert Sourcing Enterprise Question Collection and Classification.
LREC 2014 - NINTH INTERNATIONAL CONFERENCE ON LANGUAGE RESOURCES AND EVALUATION(2014)
摘要
Large enterprises, such as IBM, accumulate petabytes of free-text data within their organizations. To mine this big data, a critical ability is to enable meaningful question answering beyond keywords search. In this paper, we present a study on the characteristics and classification of IBM sales questions. The characteristics are analyzed both semantically and syntactically, from where a question classification guideline evolves. We adopted an enterprise level expert sourcing approach to gather questions, annotate questions based on the guideline and manage the quality of annotations via enhanced inter-annotator agreement analysis. We developed a question feature extraction system and experimented with rule-based, statistical and hybrid question classifiers. We share our annotated corpus of questions and report our experimental results. Statistical classifiers separately based on n-grams and hand-crafted rule features give reasonable macro-f1 scores at 61.7% and 63.1% respectively. Rule based classifier gives a macro-f1 at 77.1%. The hybrid classifier with n-gram and rule features using a second guess model further improves the macro-f1 to 83.9%.
更多查看译文
关键词
question classification,expert sourcing,machine learning
AI 理解论文
溯源树
样例
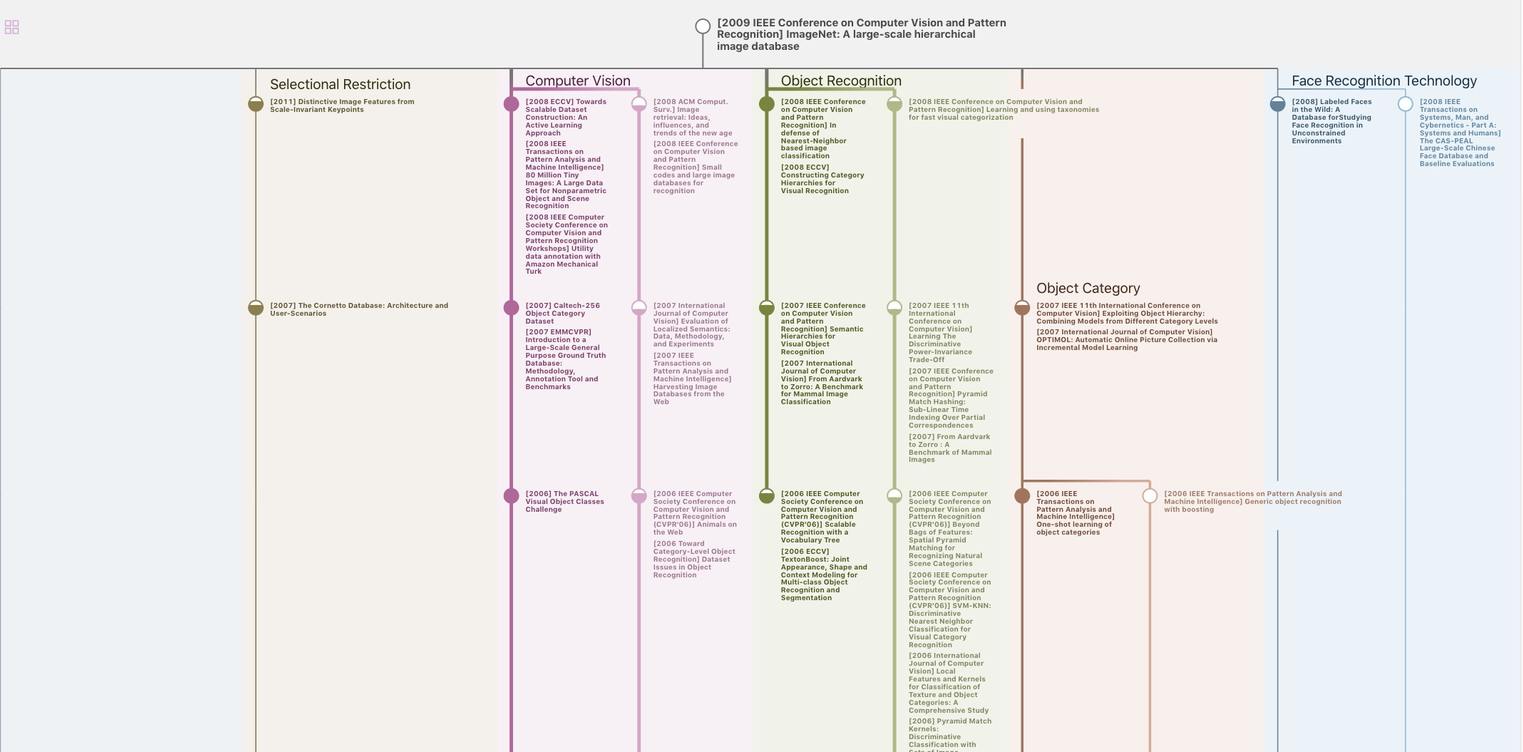
生成溯源树,研究论文发展脉络
Chat Paper
正在生成论文摘要