Traffic Sign Recognition With Multi-Scale Convolutional Networks
2011 INTERNATIONAL JOINT CONFERENCE ON NEURAL NETWORKS (IJCNN)(2011)
摘要
We apply Convolutional Networks (ConvNets) to the task of traffic sign classification as part of the GTSRB competition. ConvNets are biologically-inspired multi-stage architectures that automatically learn hierarchies of invariant features. While many popular vision approaches use hand-crafted features such as HOG or SIFT, ConvNets learn features at every level from data that are tuned to the task at hand. The traditional ConvNet architecture was modified by feeding 1(st) stage features in addition to 2(nd) stage features to the classifier. The system yielded the 2(nd)-best accuracy of 98.97% during phase I of the competition (the best entry obtained 98.98%), above the human performance of 98.81%, using 32x32 color input images. Experiments conducted after phase 1 produced a new record of 99.17% by increasing the network capacity, and by using greyscale images instead of color. Interestingly, random features still yielded competitive results (97.33%).
更多查看译文
关键词
image classification,computer vision,human performance
AI 理解论文
溯源树
样例
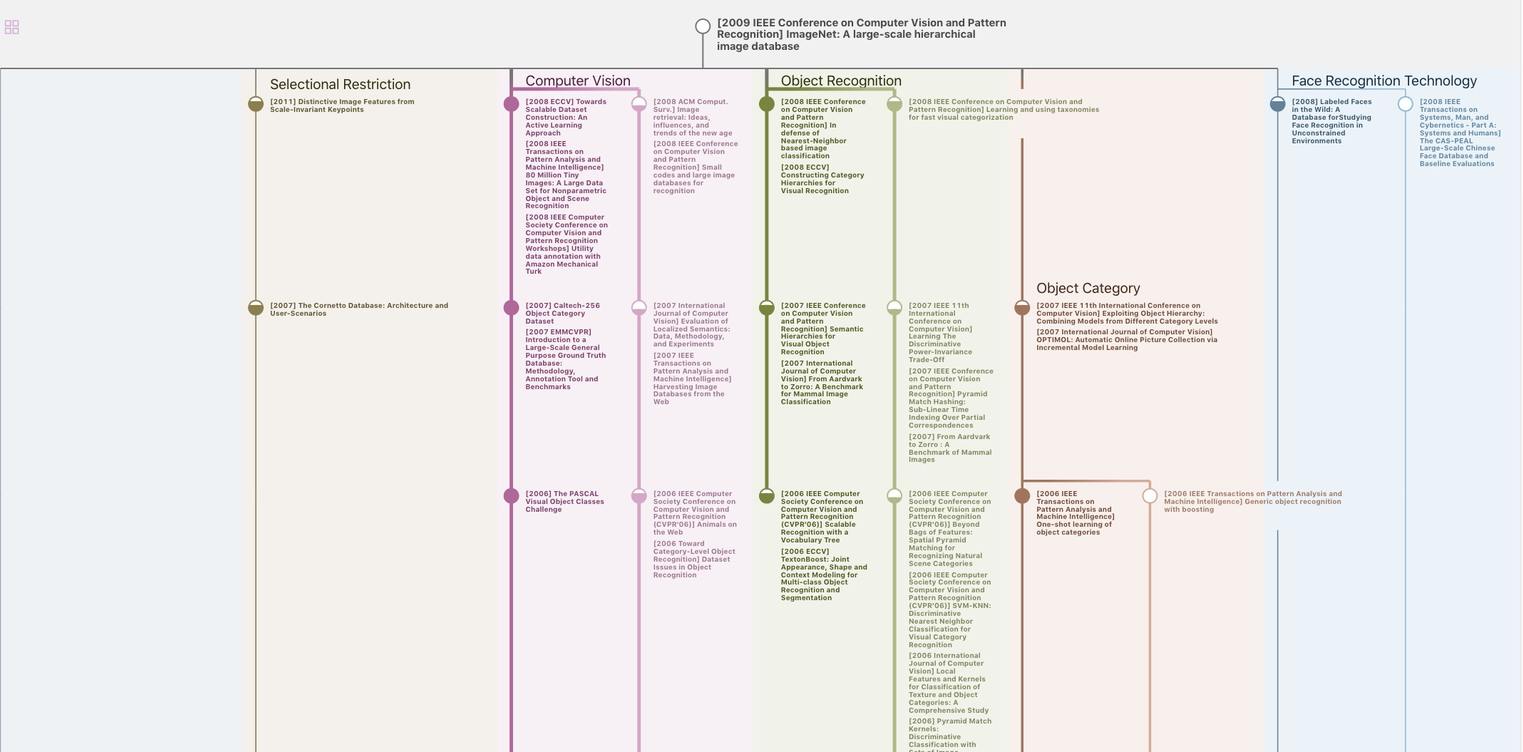
生成溯源树,研究论文发展脉络
Chat Paper
正在生成论文摘要