Spline Regression Hashing for Fast Image Search
IEEE Transactions on Image Processing(2012)
摘要
Techniques for fast image retrieval over large databases have attracted considerable attention due to the rapid growth of web images. One promising way to accelerate image search is to use hashing technologies, which represent images by compact binary codewords. In this way, the similarity between images can be efficiently measured in terms of the Hamming distance between their corresponding binary codes. Although plenty of methods on generating hash codes have been proposed in recent years, there are still two key points that needed to be improved: 1) how to precisely preserve the similarity structure of the original data and 2) how to obtain the hash codes of the previously unseen data. In this paper, we propose our spline regression hashing method, in which both the local and global data similarity structures are exploited. To better capture the local manifold structure, we introduce splines developed in Sobolev space to find the local data mapping function. Furthermore, our framework simultaneously learns the hash codes of the training data and the hash function for the unseen data, which solves the out-of-sample problem. Extensive experiments conducted on real image datasets consisting of over one million images show that our proposed method outperforms the state-of-the-art techniques.
更多查看译文
关键词
regression analysis,image retrieval,binary codes
AI 理解论文
溯源树
样例
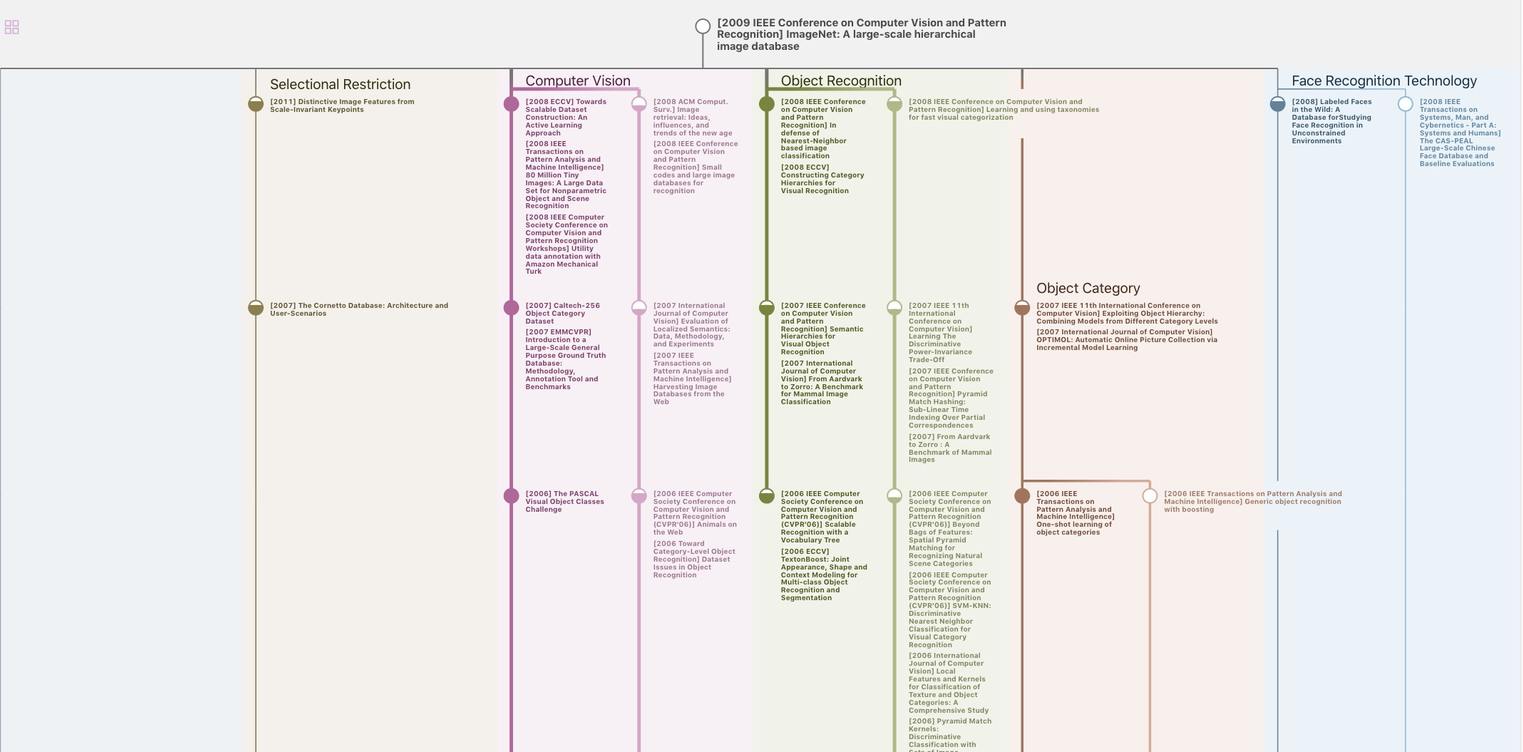
生成溯源树,研究论文发展脉络
Chat Paper
正在生成论文摘要