Superpixel-Based Segmentation of Glioblastoma Multiforme from Multimodal MR Images
MBIA(2013)
摘要
Due to complex imaging characteristics such as large diversity in shapes and appearances combining with deformation of surrounding tissues, it is a challenging task to segment glioblastoma multiforme (GBM) from multimodal MR images. In particular, it is important to capture the heterogeneous features of enhanced tumor, necrosis, and non-enhancing T2 hyperintense regions (T2HI) to determine the aggressiveness of the tumor from neuroimaging. In this paper, we propose a superpixel-based graph spectral clustering method to improve the robustness of GBM segmentation. A new graph spectral clustering algorithm is designed to group superpixels to different tissue types. First, a local k-means clustering with weighted distances is employed to segment the MR images into a number of homogeneous regions, called superpixels. Then, the spectral clustering algorithm is utilized to extract the enhanced tumor, necrosis, and T2HI by considering the superpixel map as a graph. Experiment results demonstrate better performance of the proposed method by comparing with pixel-based and the normalized cut segmentation methods.
更多查看译文
关键词
gbm,multimodal mr images,spectral clustering,superpixel
AI 理解论文
溯源树
样例
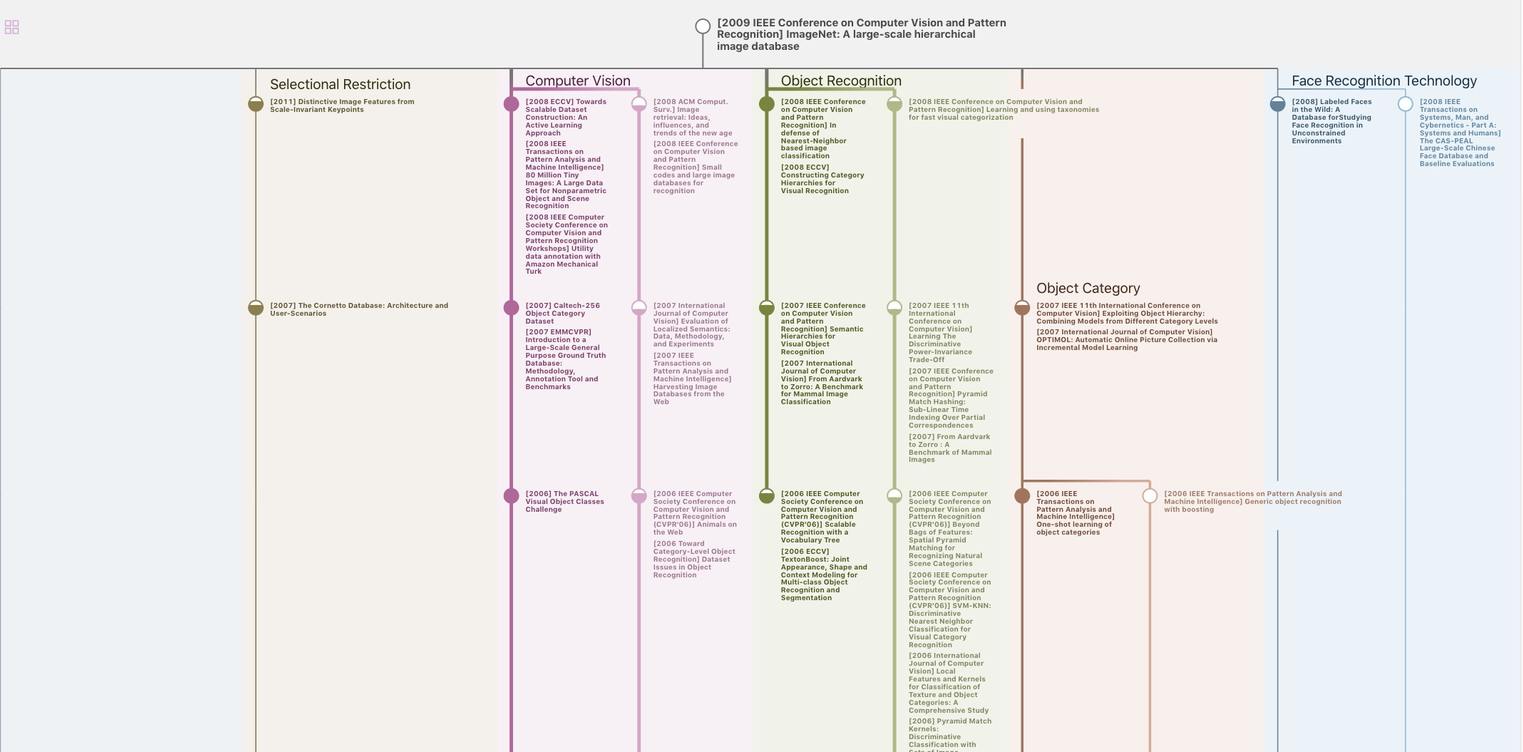
生成溯源树,研究论文发展脉络
Chat Paper
正在生成论文摘要