Density-Based Mining of Quantitative Association Rules
PAKDD(2000)
摘要
Many algorithms have been proposed for mining of boolean association rules. However, very little work has been done in mining quantitative association rules. Although we can transform quantitative attributes into boolean attributes, this approach is not effective and is difficult to scale up for high dimensional case and also may result in many imprecise association rules. Newly designed algorithms for quantitative association rules still are persecuted by nonscalable and noise problem. In this paper, an efficient algorithm, QAR-miner, is proposed. By using the notion of "density" to capture the characteristics of quantitative attributes and an efficient procedure to locate the "dense regions", QAR-miner not only can solve the problems of previous approaches, but also can scale up well for high dimensional case. Evaluations on QAR-miner have been performed using both synthetic and real databases. Preliminary results show that QAR-miner is effective and can scale up quite linearly with the increasing number of attributes.
更多查看译文
关键词
quantitative association rules,quantitative attribute,density-based mining,increasing number,efficient procedure,boolean attribute,quantitative association rule,dense region,efficient algorithm,boolean association rule,high dimensional case,imprecise association rule,association rule
AI 理解论文
溯源树
样例
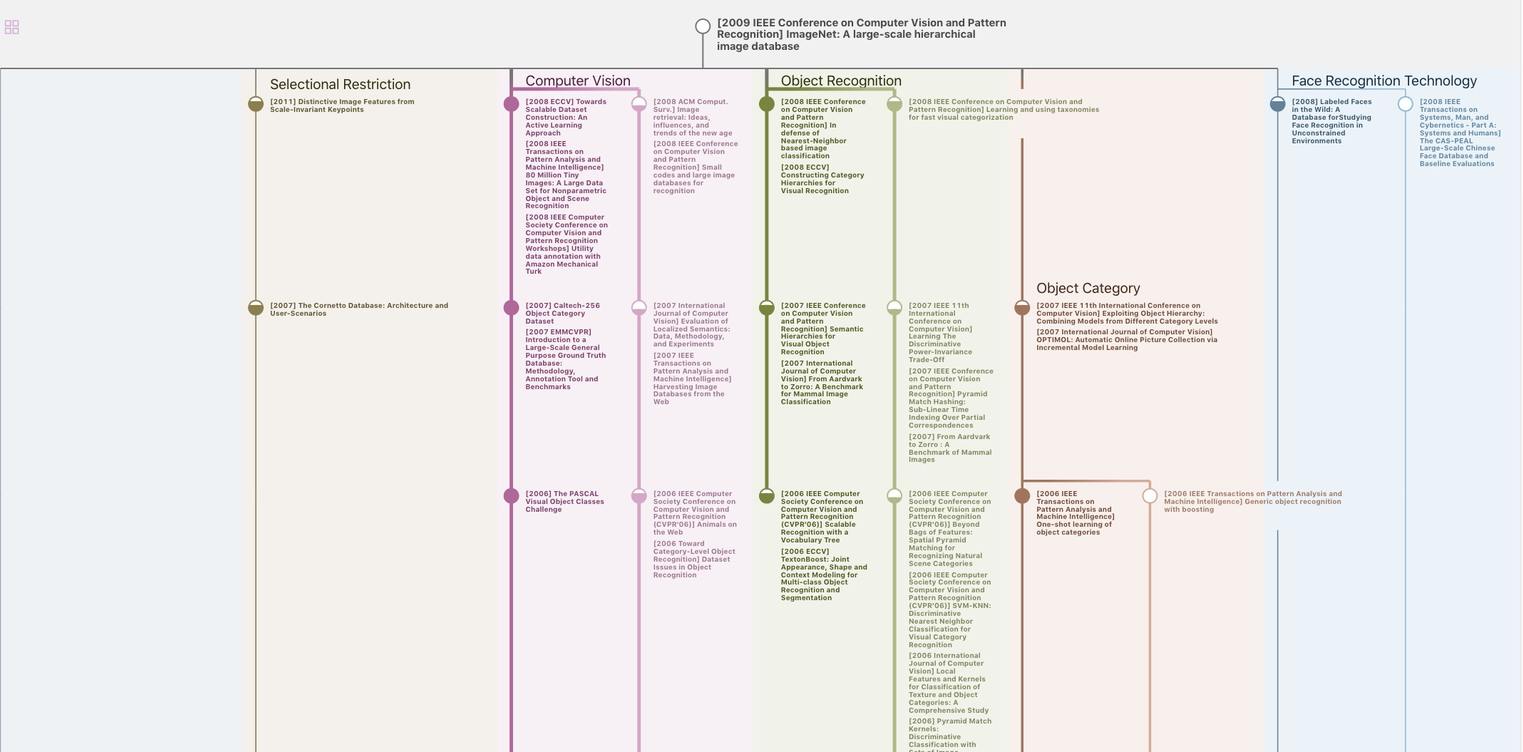
生成溯源树,研究论文发展脉络
Chat Paper
正在生成论文摘要