Multiclass Semi-supervised Learning for Animal Behavior Recognition from Accelerometer Data
ICTAI(2012)
摘要
In this paper we present a new Multiclass semi-supervised learning algorithm that uses a base classifier in combination with a similarity function applied to all data to find a classifier that maximizes the margin and consistency over all data. A novel multiclass loss function is presented and used to derive the algorithm. We apply the algorithm to animal behavior recognition from accelerometer data. Animal-borne accelerometer data are collected from free-ranging animals and then labeled by a human expert. The resulting data are used to train a classifier. However, labeling is not easy from accelerometer data only and it is often not feasible to observe animals fitted with an accelerometer. All current approaches to this behavior recognition task use supervised or unsupervised learning. Since unlabeled data are easy to acquire and collect, a semi-supervised approach seems appropriate and reduces the human efforts for labeling. Experiments with accelerometer data collected from free-ranging gulls and benchmark UCI datasets show that the algorithm is effective and compares favorably with existing algorithms for multiclass semi-supervised learning.
更多查看译文
关键词
free-ranging gull,animal behavior recognition,free-ranging animal,base classifier,multiclass semi-supervised learning,accelerometer data,unsupervised learning,behavior recognition task use,semi-supervised approach,unlabeled data,animal-borne accelerometer data,zoology,behavioural sciences,learning artificial intelligence
AI 理解论文
溯源树
样例
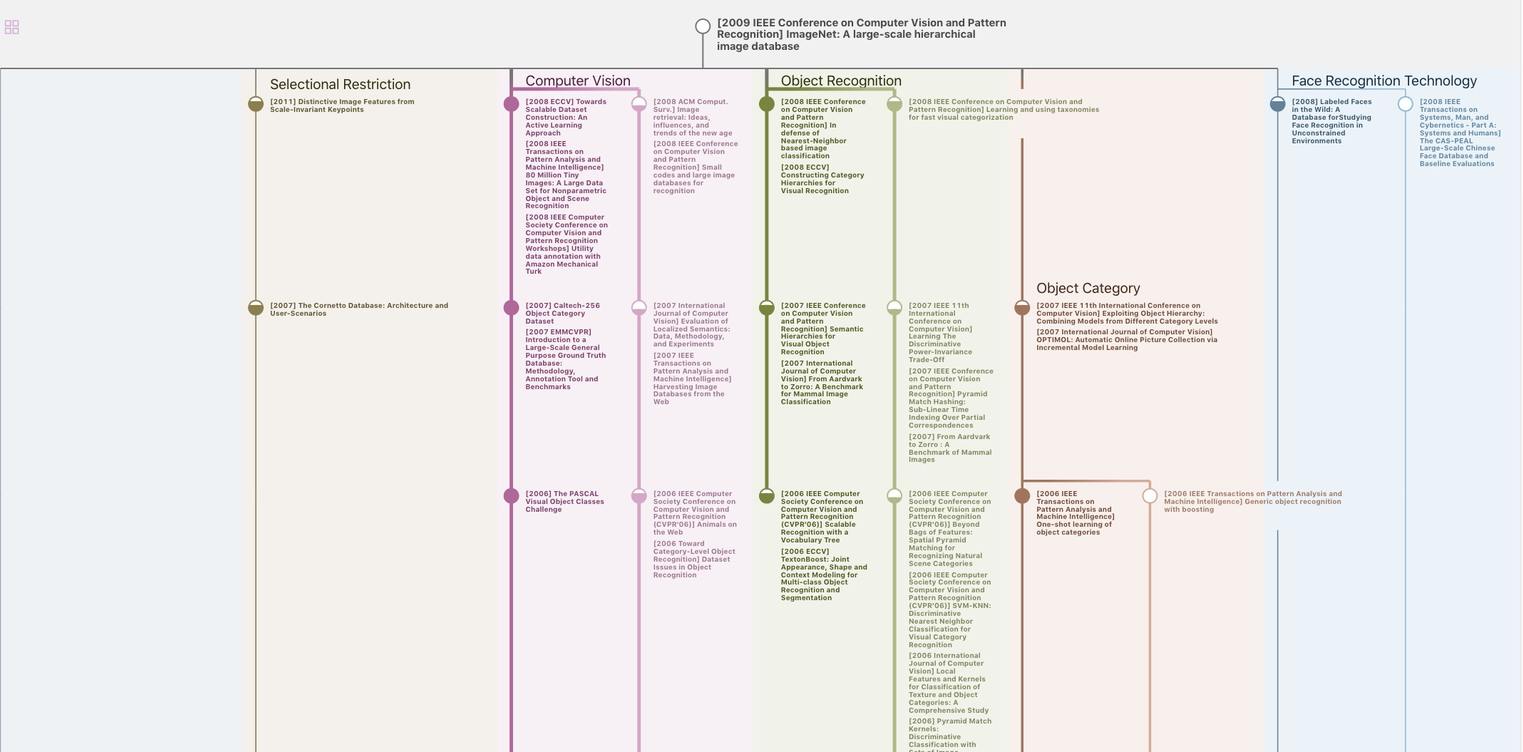
生成溯源树,研究论文发展脉络
Chat Paper
正在生成论文摘要