Learning holographic horizons
arxiv(2023)
Abstract
We apply machine learning to understand fundamental aspects of holographic
duality, specifically the entropies obtained from the apparent and event
horizon areas. We show that simple features of only the time series of the
pressure anisotropy, namely the values and half-widths of the maxima and
minima, the times these are attained, and the times of the first zeroes can
predict the areas of the apparent and event horizons in the dual bulk geometry
at all times with a fixed maximum length of the input vector. Given that simple
Vaidya-type metrics constructed just from the apparent and event horizon areas
can be used to approximately obtain unequal time correlation functions, we
argue that the corresponding entropy functions are the information that can be
learnt from a single one-point function (without explicit knowledge of the
gravitational dynamics) and that is necessary for reconstructing specific
aspects of the dual quantum state to the best possible approximations.
MoreTranslated text
AI Read Science
Must-Reading Tree
Example
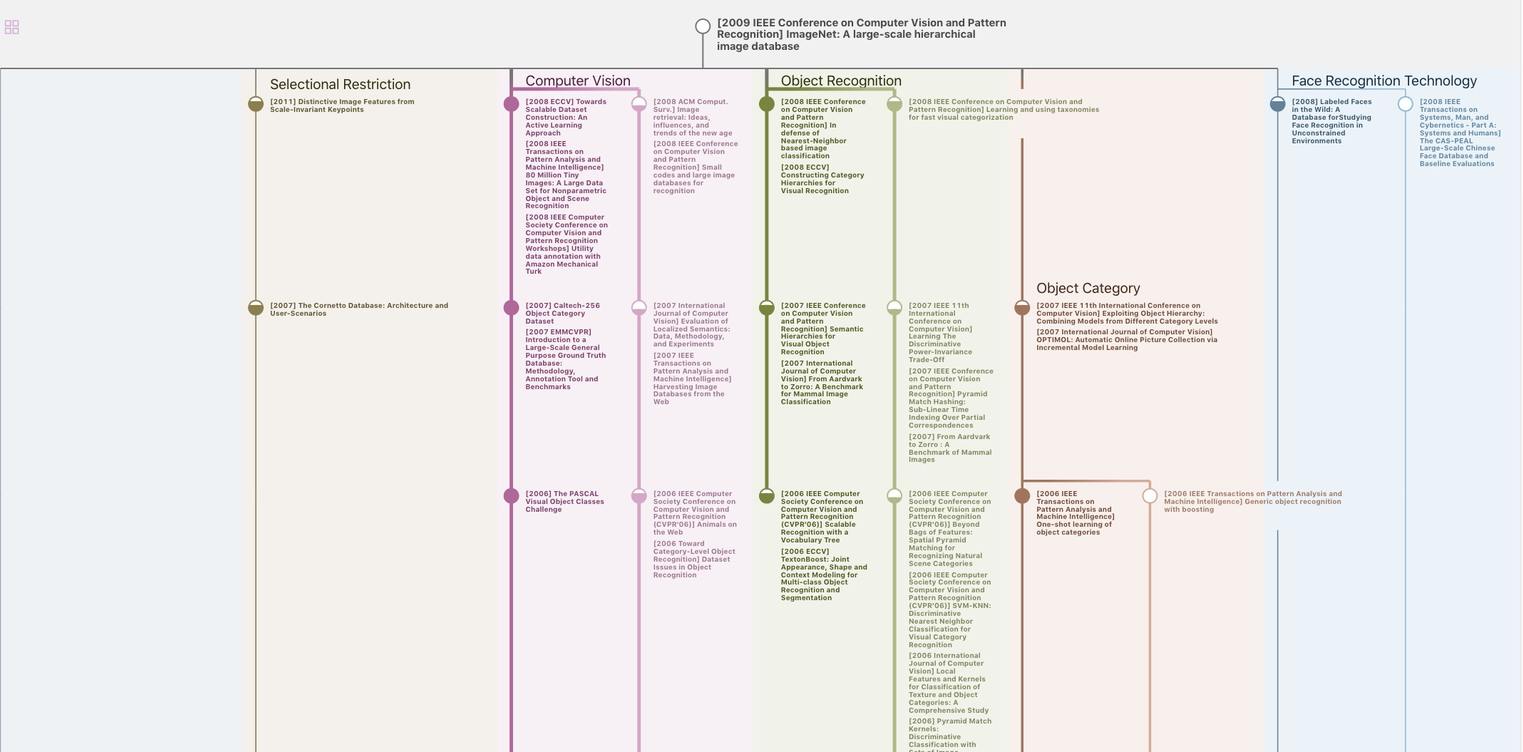
Generate MRT to find the research sequence of this paper
Chat Paper
Summary is being generated by the instructions you defined