Measure oriented training: a targeted approach to imbalanced classification problems
Frontiers of Computer Science(2012)
摘要
Since the overall prediction error of a classifier on imbalanced problems can be potentially misleading and biased, alternative performance measures such as G-mean and F-measure have been widely adopted. Various techniques including sampling and cost sensitive learning are often employed to improve the performance of classifiers in such situations. However, the training process of classifiers is still largely driven by traditional error based objective functions. As a result, there is clearly a gap between themeasure according to which the classifier is evaluated and how the classifier is trained. This paper investigates the prospect of explicitly using the appropriate measure itself to search the hypothesis space to bridge this gap. In the case studies, a standard threelayer neural network is used as the classifier, which is evolved by genetic algorithms (GAs) with G-mean as the objective function. Experimental results on eight benchmark problems show that the proposed method can achieve consistently favorable outcomes in comparison with a commonly used sampling technique. The effectiveness of multi-objective optimization in handling imbalanced problems is also demonstrated.
更多查看译文
关键词
imbalanced datasets,genetic algorithms (GAs),neural networks,G-mean,synthetic minority over-sampling technique (SMOTE)
AI 理解论文
溯源树
样例
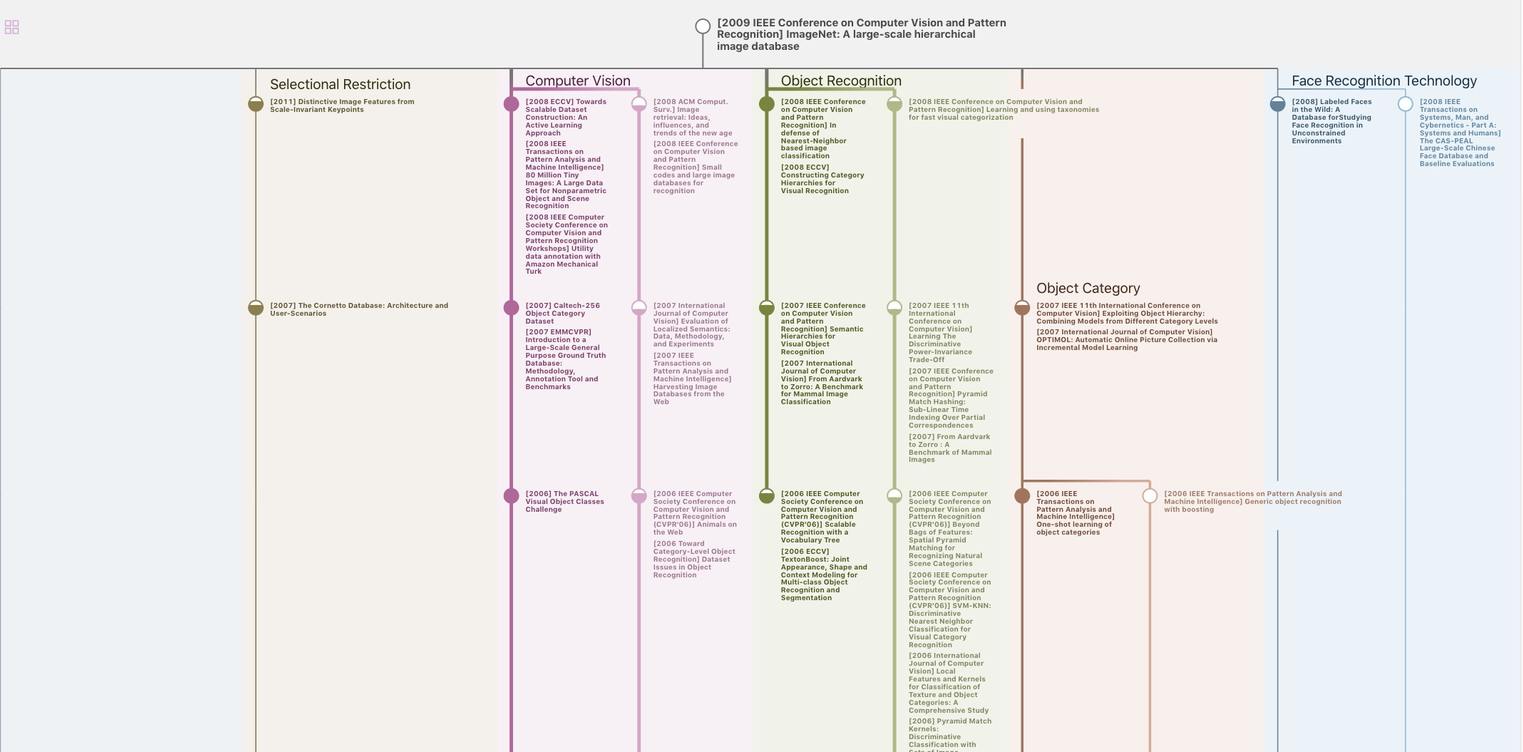
生成溯源树,研究论文发展脉络
Chat Paper
正在生成论文摘要