Deep networks for robust visual recognition
ICML(2010)
摘要
Deep Belief Networks (DBNs) are hierarchi- cal generative models which have been used successfully to model high dimensional visual data. However, they are not robust to com- mon variations such as occlusion and random noise. We explore two strategies for improv- ing the robustness of DBNs. First, we show that a DBN with sparse connections in the rst layer is more robust to variations that are not in the training set. Second, we de- velop a probabilistic denoising algorithm to determine a subset of the hidden layer nodes to unclamp. We show that this can be ap- plied to any feedforward network classier with localized rst layer connections. Recog- nition results after denoising are signicantly better over the standard DBN implementa- tions for various sources of noise.
更多查看译文
关键词
belief network
AI 理解论文
溯源树
样例
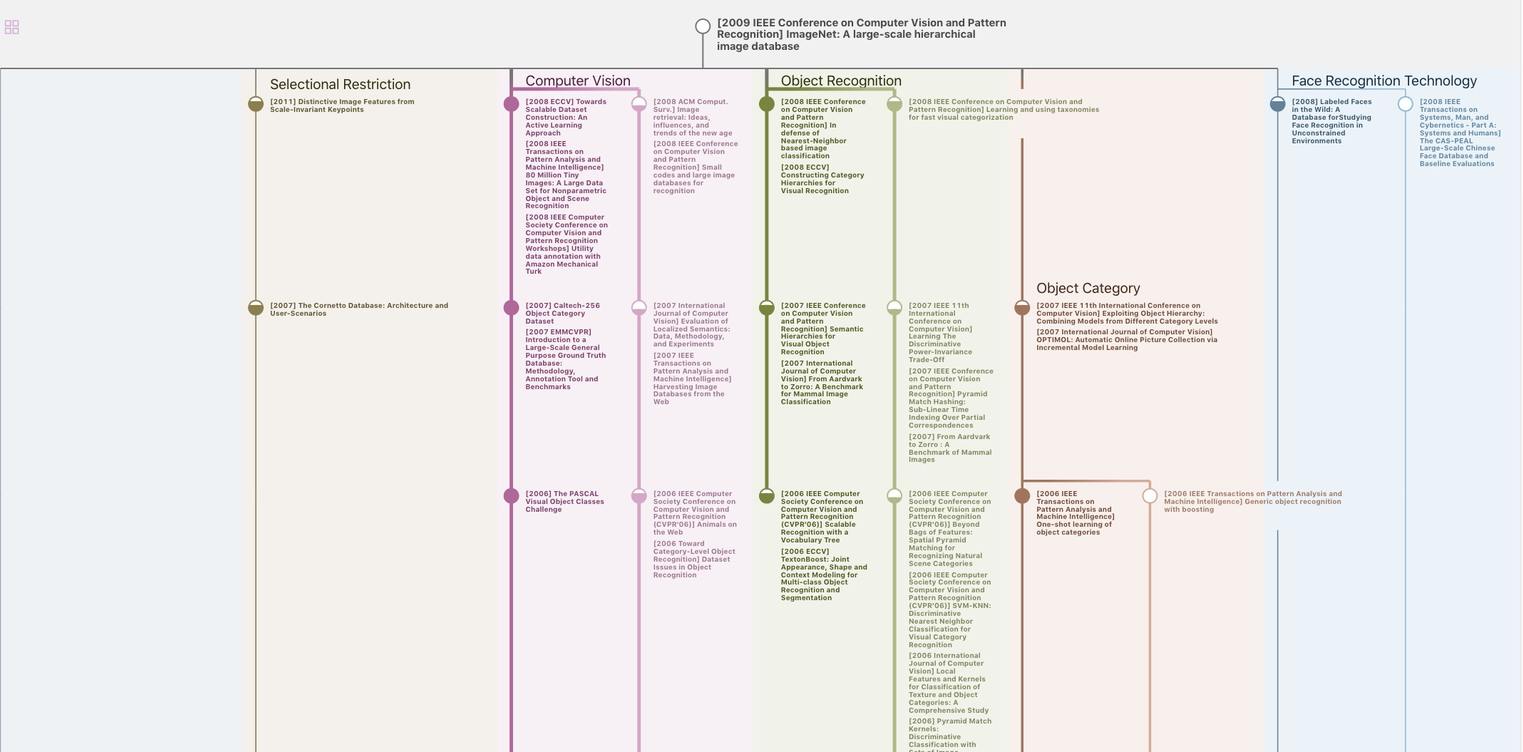
生成溯源树,研究论文发展脉络
Chat Paper
正在生成论文摘要