Sensitivity of regionalized transfer-function noise models to the input and parameter transfer method
HYDROLOGICAL SCIENCES JOURNAL-JOURNAL DES SCIENCES HYDROLOGIQUES(2009)
摘要
Monthly shallow water-table depths can be predicted from climate forcings on a large scale by the combination of a transfer-function noise (TFN) model with a parameter regionalization scheme. To investigate the sensitivity of regionalized transfer-function noise (RTFN) models, simulations with different input series and parameter regionalization methods, combined with topographic external drifts, are presented. The input series include the precipitation, precipitation surplus or infiltration calculated by the variable infiltration capacity (VIC) land surface model, and the regionalization methods include those based on a combination of the principal component analysis (PCA) classification or Gaussian mixture model (GMM) clustering, with the external drifts, such as the elevation or terrain slope, generated from digital elevation models (DEM). Verification and cross-validation show that the infiltration series reduces the errors for regionalization by 12.5-18.8%, but cannot improve the calibration. The PCA method outperforms its alternative GMM method for the input series and the external drifts. The combination of the infiltration series and the regionalization method based on PCA classification and elevation produces the best results with respect to the mean absolute error and root mean squared error. The spatial and temporal variations of water-table depths at a macro-scale in continental China are predicted by the combination.
更多查看译文
关键词
sensitivity,transfer-function noise,regionalization,principal component analysis,water table
AI 理解论文
溯源树
样例
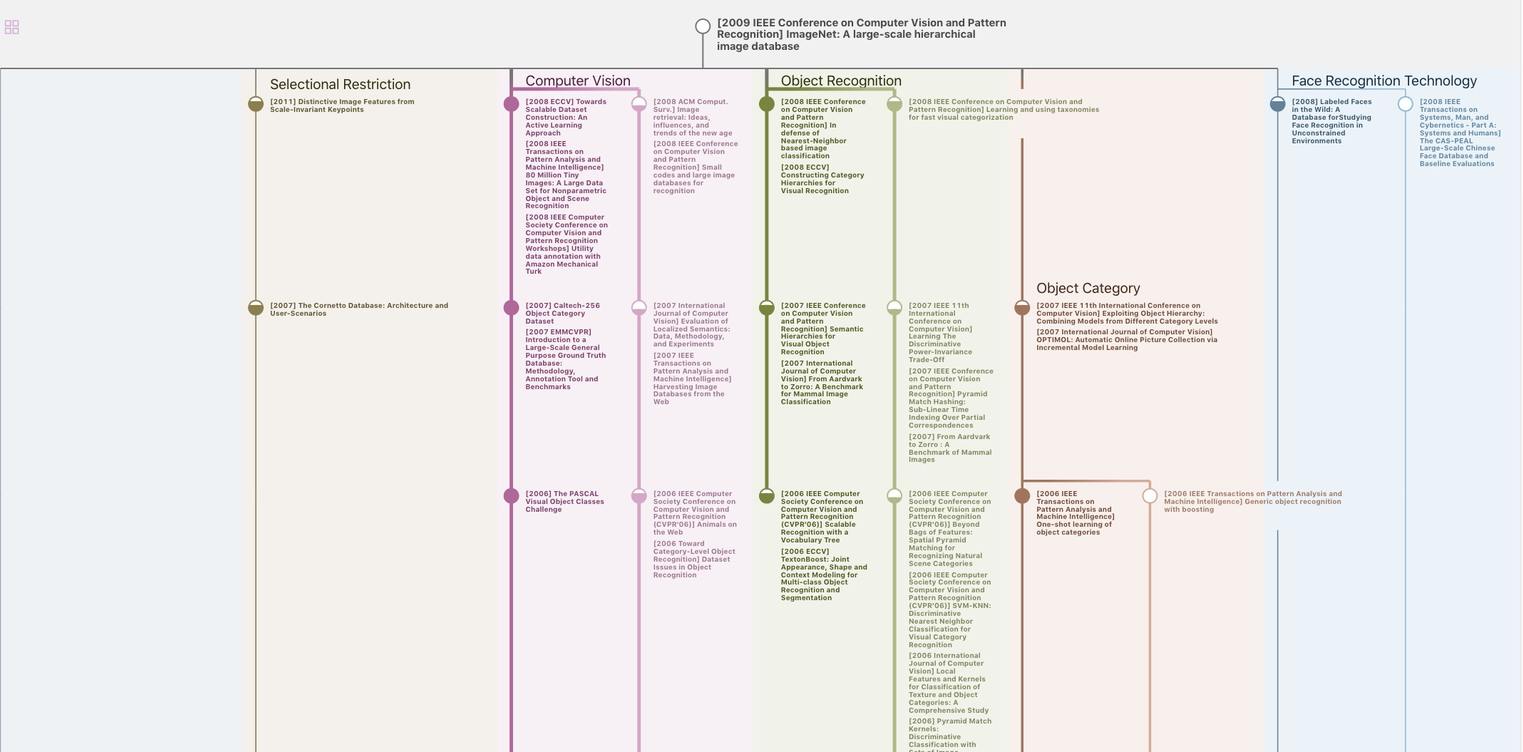
生成溯源树,研究论文发展脉络
Chat Paper
正在生成论文摘要