Subjectivity Recognition on Word Senses via Semi-supervised Mincuts.
NAACL '09: Proceedings of Human Language Technologies: The 2009 Annual Conference of the North American Chapter of the Association for Computational Linguistics(2009)
摘要
We supplement WordNet entries with information on the subjectivity of its word senses. Supervised classifiers that operate on word sense definitions in the same way that text classifiers operate on web or newspaper texts need large amounts of training data. The resulting data sparseness problem is aggravated by the fact that dictionary definitions are very short. We propose a semi-supervised minimum cut framework that makes use of both WordNet definitions and its relation structure. The experimental results show that it outperforms supervised minimum cut as well as standard supervised, non-graph classification, reducing the error rate by 40%. In addition, the semi-supervised approach achieves the same results as the supervised framework with less than 20% of the training data.
更多查看译文
关键词
training data,data sparseness problem,supervised framework,WordNet definition,WordNet entry,minimum cut,semi-supervised approach,semi-supervised minimum cut framework,word sense,word sense definition,semi-supervised mincuts,subjectivity recognition
AI 理解论文
溯源树
样例
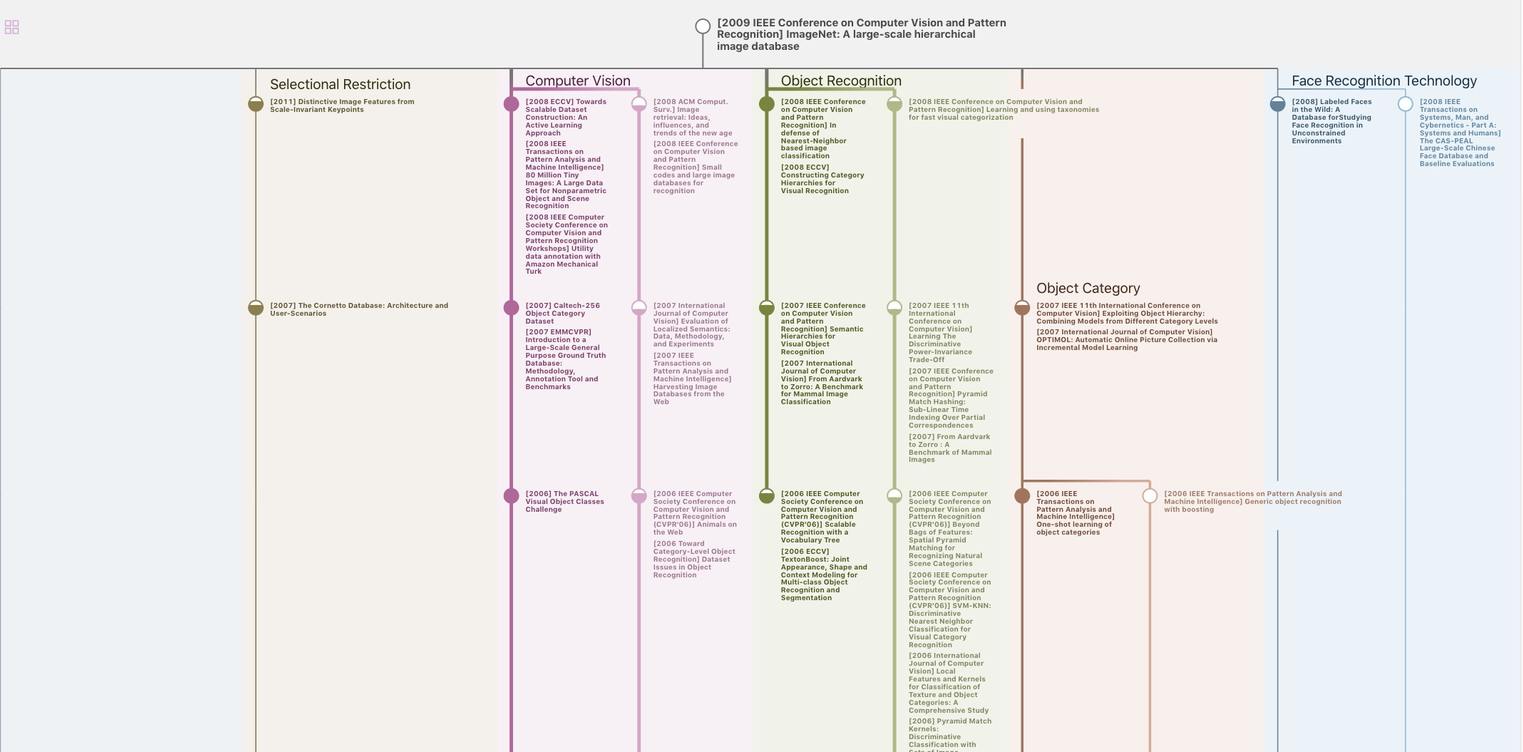
生成溯源树,研究论文发展脉络
Chat Paper
正在生成论文摘要