Selecting good expansion terms for pseudo-relevance feedback.
IR(2008)
摘要
ABSTRACTPseudo-relevance feedback assumes that most frequent terms in the pseudo-feedback documents are useful for the retrieval. In this study, we re-examine this assumption and show that it does not hold in reality - many expansion terms identified in traditional approaches are indeed unrelated to the query and harmful to the retrieval. We also show that good expansion terms cannot be distinguished from bad ones merely on their distributions in the feedback documents and in the whole collection. We then propose to integrate a term classification process to predict the usefulness of expansion terms. Multiple additional features can be integrated in this process. Our experiments on three TREC collections show that retrieval effectiveness can be much improved when term classification is used. In addition, we also demonstrate that good terms should be identified directly according to their possible impact on the retrieval effectiveness, i.e. using supervised learning, instead of unsupervised learning.
更多查看译文
AI 理解论文
溯源树
样例
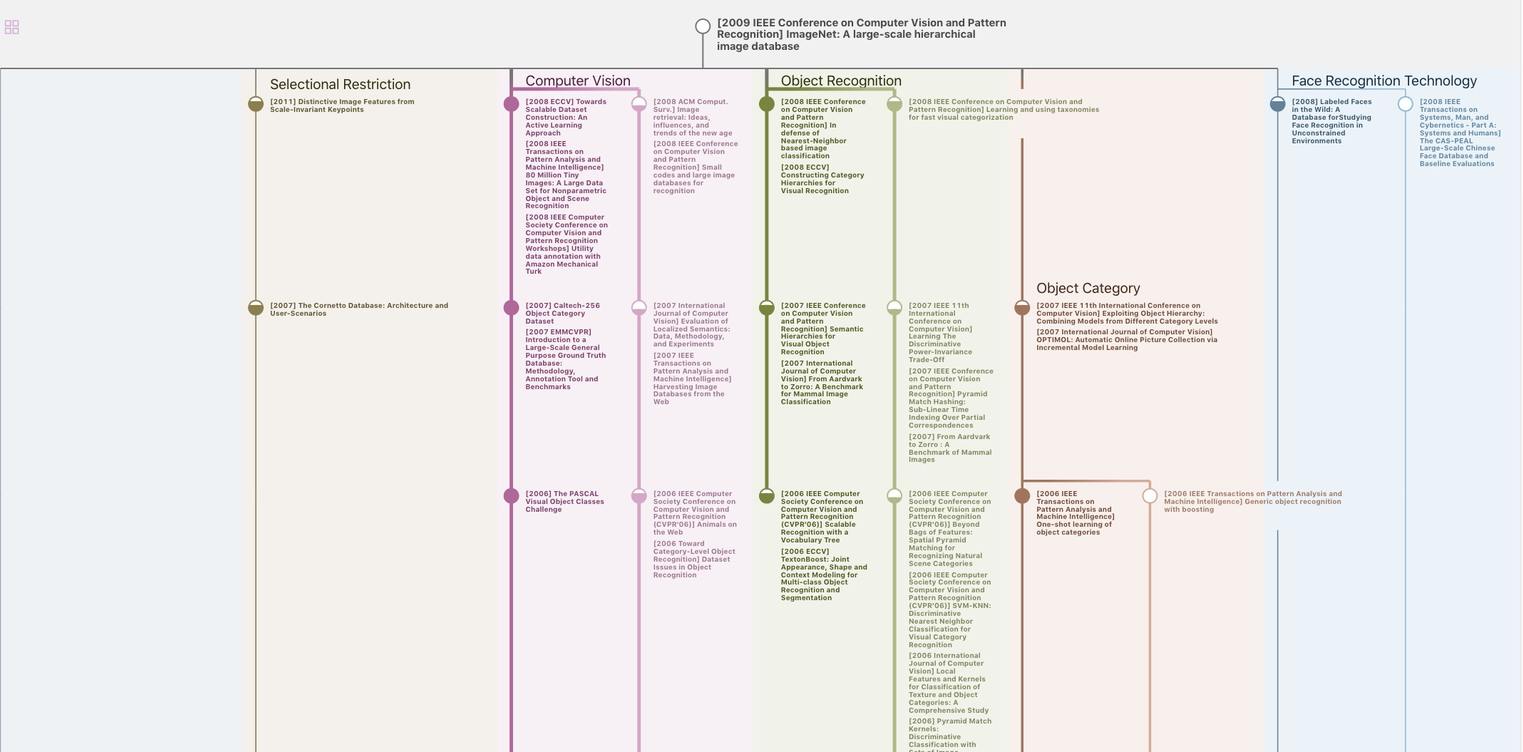
生成溯源树,研究论文发展脉络
Chat Paper
正在生成论文摘要