Leveraging Multi-Domain Prior Knowledge in Topic Models.
IJCAI '13: Proceedings of the Twenty-Third international joint conference on Artificial Intelligence(2013)
摘要
Topic models have been widely used to identify topics in text corpora. It is also known that purely unsupervised models often result in topics that are not comprehensible in applications. In recent years, a number of knowledge-based models have been proposed, which allow the user to input prior knowledge of the domain to produce more coherent and meaningful topics. In this paper, we go one step further to study how the prior knowledge from other domains can be exploited to help topic modeling in the new domain. This problem setting is important from both the application and the learning perspectives because knowledge is inherently accumulative. We human beings gain knowledge gradually and use the old knowledge to help solve new problems. To achieve this objective, existing models have some major difficulties. In this paper, we propose a novel knowledge-based model, called MDK-LDA, which is capable of using prior knowledge from multiple domains. Our evaluation results will demonstrate its effectiveness.
更多查看译文
关键词
prior knowledge,human beings gain knowledge,input prior knowledge,old knowledge,knowledge-based model,meaningful topic,multiple domain,new domain,new problem,novel knowledge-based model,multi-domain prior knowledge,topic model
AI 理解论文
溯源树
样例
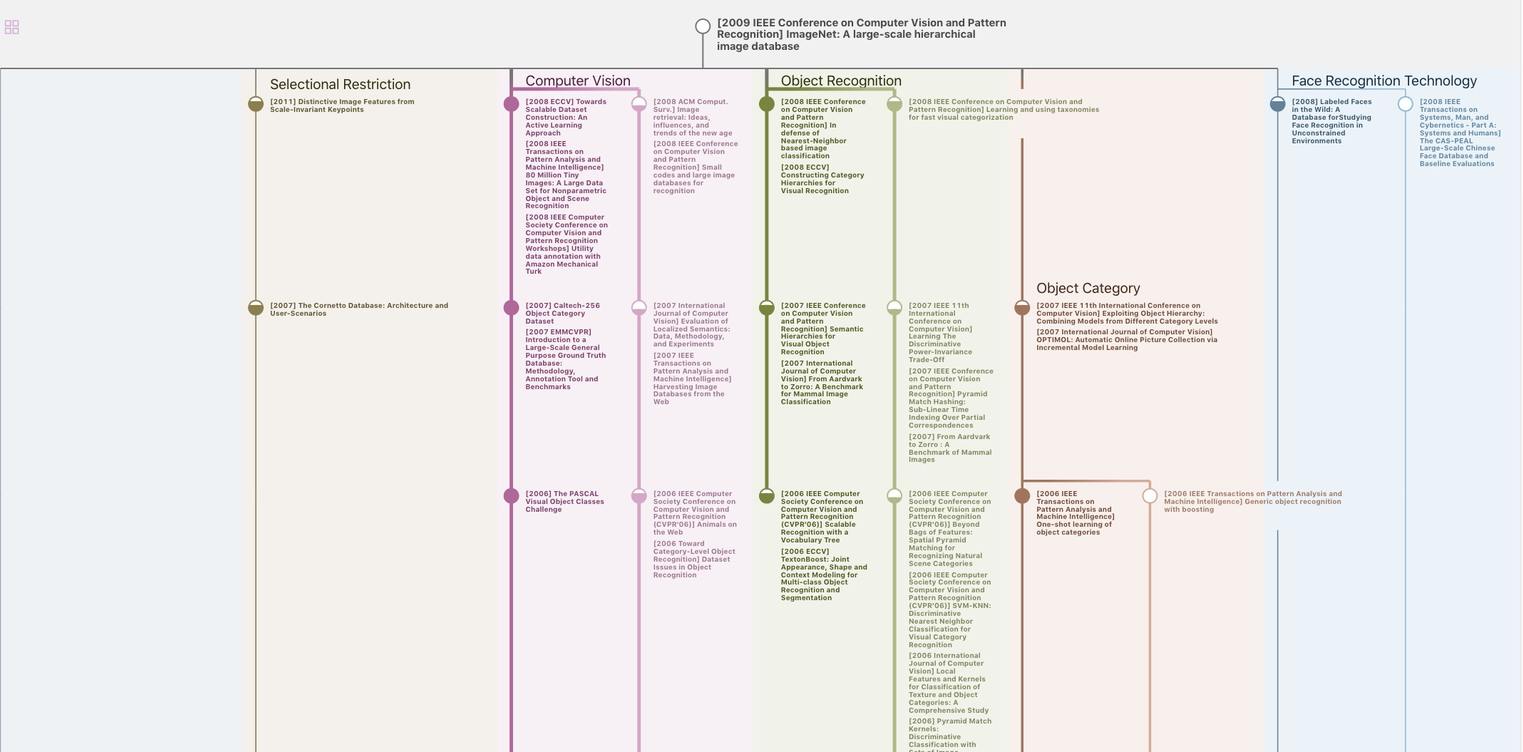
生成溯源树,研究论文发展脉络
Chat Paper
正在生成论文摘要