Clustering for Data Reduction: A Divide and Conquer Approach
msra(2007)
摘要
We consider the problem of reducing a potentially very large dataset to a subset of representative prototypes. Rather than searching over the entire space of prototypes, we rst roughly divide the data into balanced clusters using bisecting k-means and spectral cuts, and then nd the prototypes for each cluster by anity propagation. We apply our algorithm to text data, where we perform an order of magnitude faster than simply looking for prototypes on the entire dataset. Furthermore, our \divide and conquer" approach actually performs more accurately on datasets which are well bisected, as the greedy decisions of anity propagation are conned to classes of already similar items.
更多查看译文
AI 理解论文
溯源树
样例
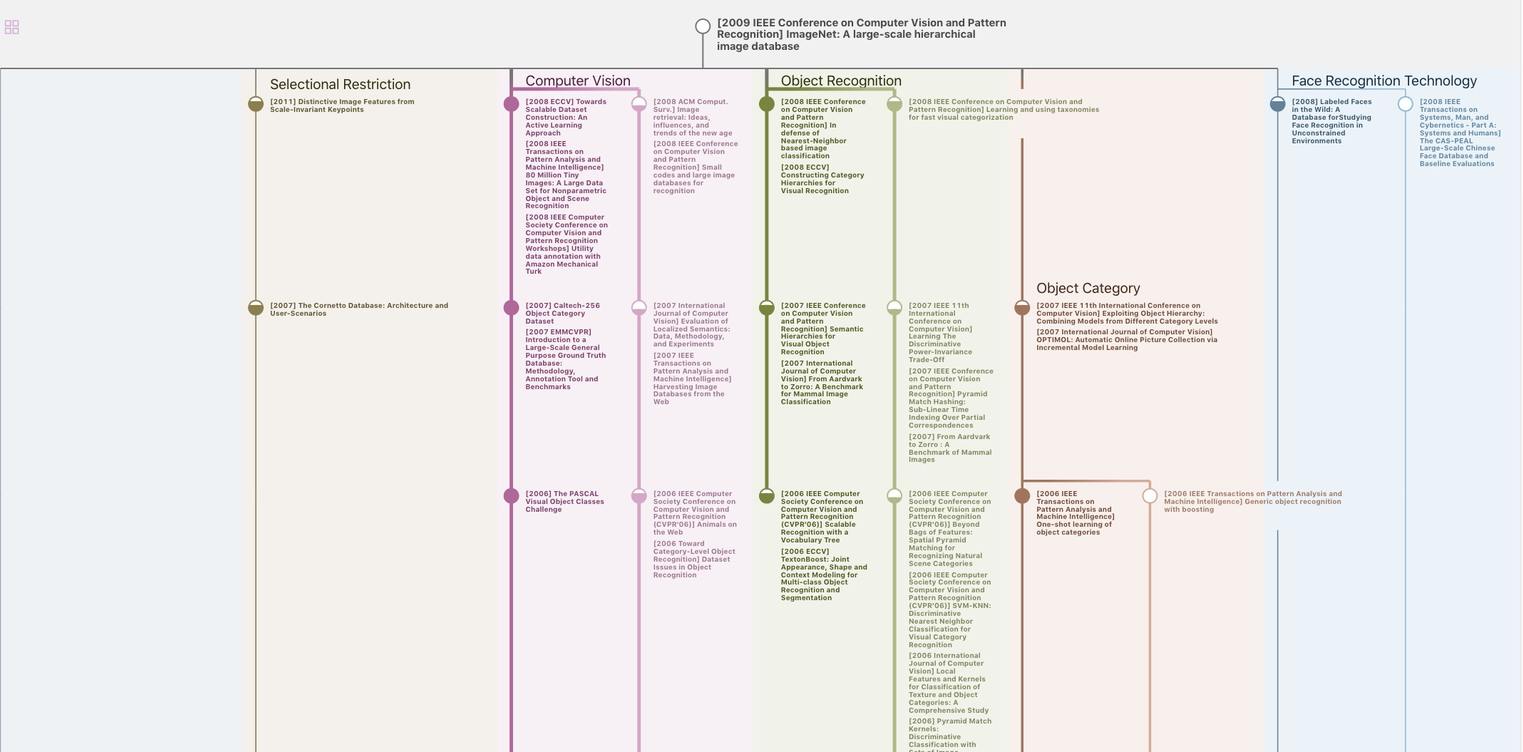
生成溯源树,研究论文发展脉络
Chat Paper
正在生成论文摘要