Locality Constrained Dictionary Learning for Nonlinear Dimensionality Reduction
IEEE Signal Process. Lett.(2013)
摘要
Current nonlinear dimensionality reduction (NLDR) algorithms have quadratic or cubic complexity in the number of data, which limits their ability to process real-world large-scale datasets. Learning over a small set of landmark points can potentially allow much more effective NLDR and make such algorithms scalable to large dataset problems. In this paper, we show that the approximation to an unobservable intrinsic manifold by a few latent points residing on the manifold can be cast in a novel dictionary learning problem over the observation space. This leads to the presented locality constrained dictionary learning (LCDL) algorithm, which effectively learns a compact set of atoms consisting of locality-preserving landmark points on a nonlinear manifold. Experiments comparing state-of-the-art DL algorithms, including K-SVD, LCC and LLC, show that LCDL improves the embedding quality and greatly reduces the complexity of NLDR algorithms.
更多查看译文
关键词
lcc,locality-preserving landmark point,nldr algorithm,llc,k-svd,lcdl,face recognition,nonlinear dimensionality reduction,quadratic complexity,dimensionality reduction,locality constrained dictionary learning,lcdl algorithm,state-of-the-art dl algorithm,dictionary learning,real-world large-scale dataset processing,cubic complexity,computer vision,nonlinear manifold,manifold learning,approximation algorithms,geometry,k svd,dictionaries,manifolds,image reconstruction
AI 理解论文
溯源树
样例
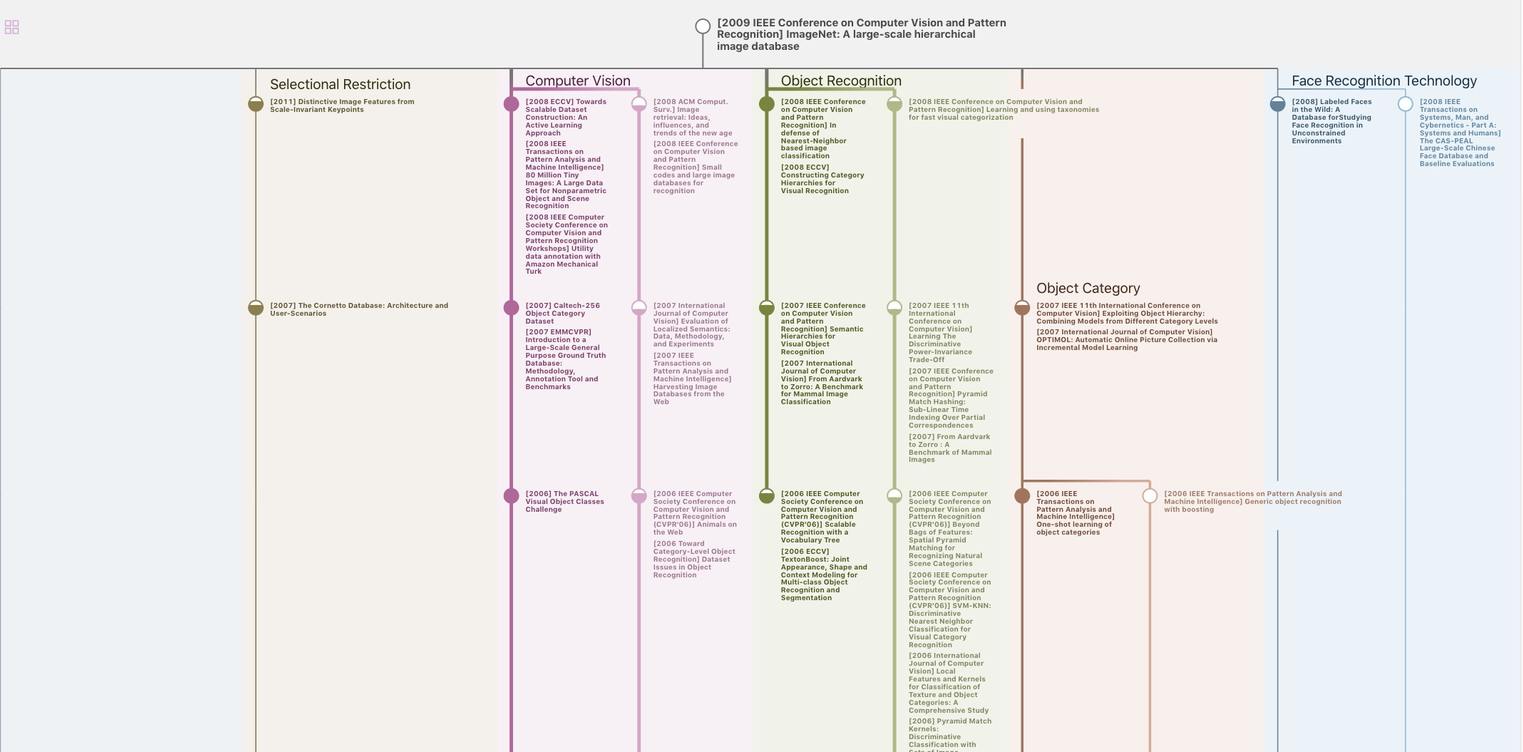
生成溯源树,研究论文发展脉络
Chat Paper
正在生成论文摘要