Evaluation and comparison of GLM- and CVA-based fMRI processing pipelines with Java-based fMRI processing pipeline evaluation system.
NeuroImage(2008)
摘要
Activation patterns identified by fMRI processing pipelines or fMRI software packages are usually determined by the preprocessing options, parameters, and statistical models used. Previous studies that evaluated options of GLM (general linear model)-based fMRI processing pipelines are mainly based on simulated data with receiver operating characteristics (ROC) analysis, but evaluation of such fMRI processing pipelines on real fMRI data is rare. To understand the effect of processing options on performance of GLM-based fMRI processing pipelines with real fMRI data, we investigated the impact of commonly-used fMRI preprocessing steps; optimized the associated GLM-based single-subject processing pipelines; and quantitatively compared univariate GLM (in FSL.FEAT and NPAIRS.GLM) and multivariate CVA (canonical variates analysis) (in NPAIRS.CVA)-based analytic models in single-subject analysis with a recently developed fMRI processing pipeline evaluation system based on prediction accuracy (classification accuracy) and reproducibility performance metrics. For block-design data, we found that with GLM analysis (1) slice timing correction and global intensity normalization have little consistent impact on fMRI processing pipelines, spatial smoothing and high-pass filtering or temporal detrending significantly increases pipeline performance and thus are essential for robust fMRI statistical analysis; (2) combined optimization of spatial smoothing and temporal detrending improves pipeline performance; and (3) in general, the prediction performance of multivariate CVA is higher than that of the univariate GLM, while univariate GLM is more reproducible than multivariate CVA. Because of the different bias–variance trade-offs of univariate and multivariate models, it may be necessary to consider a consensus approach to obtain more accurate activation patterns in fMRI data.
更多查看译文
关键词
fMRI,GLM,CVA,Prediction accuracy,Classification accuracy,Reproducibility
AI 理解论文
溯源树
样例
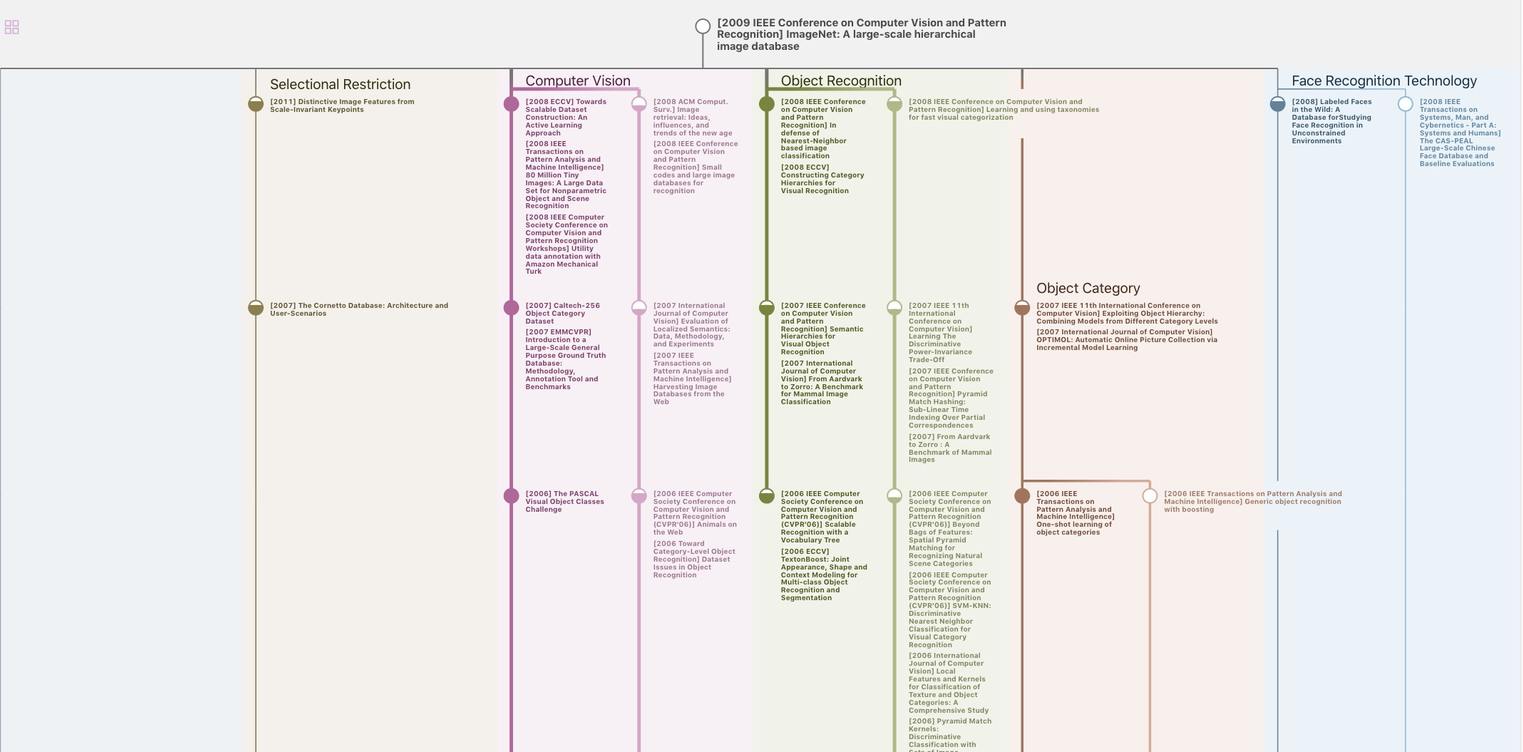
生成溯源树,研究论文发展脉络
Chat Paper
正在生成论文摘要